Menu
Publications
2024
2023
2022
2021
2020
2019
2018
2017
2016
2015
2014
2013
2012
2011
2010
2009
2008
2007
2006
2005
2004
2003
2002
2001
Editor-in-Chief
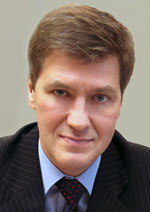
Nikiforov
Vladimir O.
D.Sc., Prof.
Partners
doi: 10.17586/2226-1494-2021-21-6-942-950
Social media user identity linkage by graphic content comparison
Read the full article

Article in Russian
For citation:
Abstract
For citation:
Korepanova A.A., Abramov M.V., Tulupyev A.L. Social media user identity linkage by graphic content comparison. Scientific and Technical Journal of Information Technologies, Mechanics and Optics, 2021, vol. 21, no. 6, pp. 942–950 (in Russian). doi: 10.17586/2226-1494-2021-21-6-942-950
Abstract
The article proposes a new approach to comparing accounts of the social media “VKontakte” and “Instagram” to determine those accounts which belong to the same user. The approach is based on the comparison of graphic content; the novelty of the approach consists in combining several methods for matching graphic content, also for the first time a method is proposed for matching accounts of the mentioned social media. The proposed method combines three methods of matching graphic content: by extracting the faces of the account users from the photos in the account and matching them, by matching all faces in both accounts, and by pairwise comparison of images to determine the same images in both accounts using the perceptual pHash method. The described method was tested on a dataset of more than 8,000 pairs of accounts. According to the results of the experiment, the value of the F1-score metric reached 0.87. The practical significance lies in automating the comparison of user accounts in various social networks by implementing of the developed algorithm in the prototype of the software package. A further direction for research lies in expanding the set of data and attributes of profiles considered for comparison. The results can be introduced into a software package for the analysis of the security of a user of information systems against social engineering attacks. It seems promising to combine the obtained findings with account matching methods based on the structural similarity of social graphs.
Keywords: social media, user identity linkage, image processing, machine learning, social engineering attacks
Acknowledgements. This work was carried out within the framework of the project under the state assignment of SPC RAS SPIIRAS No. 0073-2019-0003 (approach formation); supported by Saint Petersburg State University, project No. 73555239 (implementation of the approach and its approbation); with the financial support of the RFBR, project No. 20-07-00839 (approbation of the results in the prototype of the software package).
References
Acknowledgements. This work was carried out within the framework of the project under the state assignment of SPC RAS SPIIRAS No. 0073-2019-0003 (approach formation); supported by Saint Petersburg State University, project No. 73555239 (implementation of the approach and its approbation); with the financial support of the RFBR, project No. 20-07-00839 (approbation of the results in the prototype of the software package).
References
-
Camacho D., Panizo-LLedot Á., Bello-Orgaz G., Gonzalez-Pardo A., Cambria E. The four dimensions of social network analysis: An overview of research methods, applications, and software tools // Information Fusion. 2020. V. 63. P. 88–120. https://doi.org/10.1016/j.inffus.2020.05.009
-
Yamane D., Yamane P., Ivory S.L. Targeted advertising: Documenting the emergence of Gun Culture 2.0 in Guns magazine, 1955–2019 // Palgrave Communications. 2020. V. 6. N 1. P. 61. https://doi.org/10.1057/s41599-020-0437-0
-
Hinds J., Williams E.J., Joinson A.N. “It wouldn't happen to me”: Privacy concerns and perspectives following the Cambridge Analytica scandal // International Journal of Human Computer Studies. 2020. V. 143. P. 102498. https://doi.org/10.1016/j.ijhcs.2020.102498
-
Yu X., Yang Q., Wang R., Fang R., Deng M. Data cleaning for personal credit scoring by utilizing social media data: An empirical study // IEEE Intelligent Systems. 2020. V. 35. N 2. P. 7–15. https://doi.org/10.1109/MIS.2020.2972214
-
Óskarsdóttir M., Bravo C., Sarraute C., Vanthienen J., Baesens B. The value of big data for credit scoring: Enhancing financial inclusion using mobile phone data and social network analytics // Applied Soft Computing Journal. 2019. V. 74. P. 26–39. https://doi.org/10.1016/j.asoc.2018.10.004
-
Guo G., Zhu F., Chen E., Liu Q., Wu L., Guan C. From footprint to evidence: An exploratory study of mining social data for credit scoring // ACM Transactions on the Web. 2016. V. 10. N 4. P. 1–38. https://doi.org/10.1145/2996465
-
Азаров А.А., Тулупьева Т.В., Суворова А.В., Тулупьев А.Л., Абрамов М.В., Юсупов Р.М.Социоинженерные атаки. Проблемы анализа. СПб.: Наука, 2016. 349 с.
-
Абрамов М.В., Тулупьева Т.В., Тулупьев А.Л. Социоинженерные атаки: социальные сети и оценки защищенности пользователей. СПб.: ГУАП, 2018. 266 с.
-
CinelliM., QuattrociocchiW., GaleazziA., ValensiseC.M., Brugnoli E., Schmidt A.L., Zola P., Zollo F., Scala A. The COVID-19 social media infodemic // Scientific Reports. 2020. V. 10. P. 16598. https://doi.org/10.1038/s41598-020-73510-5
-
Khlobystova A.O., Abramov M.V., Tulupyev A.L. Soft estimates for social engineering attack propagation probabilities depending on interaction rates among instagram users // Studies in Computational Intelligence. 2020. V. 868. P. 272–277.https://doi.org/10.1007/978-3-030-32258-8_32
-
Oliseenko V., Korepanova A. How old users are? Community analysis // CEUR Workshop Proceedings. 2020. V. 2782. P. 246–251.
-
Хлобыстова А.О., Абрамов М.В., Тулупьев А.Л., Золотин А.А. Поиск кратчайшей траектории социоинженерной атаки между парой пользователей в графе с вероятностями переходов // Информационно-управляющие системы. 2018. № 6. С. 74–81. https://doi.org/10.31799/1684-8853-2018-6-74-81
-
Корепанова А.А., Абрамов М.В., Тулупьева Т.В. Идентификация аккаунтов пользователей в социальных сетях "ВКонтакте" и "Одноклассники" // Семнадцатая Национальная конференция по искусственному интеллекту с международным участием. КИИ-2019: сборник научных трудов. в 2-х томах. Т. 2. 2019. С. 153–163.
-
Корепанова А.А., Тулупьева Т.В. Идентификация аккаунтов пользователя в различных социальных сетях по социальному окружению// Информационная безопасность регионов России (ИБРР-2019): материалы конференции. СПб., 2019. С. 442–443.
-
Liu J., Zhang F., Song X., Song Y.-I., Lin C.-Y., Hon H.-W. What’s in a name? An unsupervised approach to link users across communities // Proc. of the 6th ACM International Conference on Web Search and Data Mining (WSDM). 2013. P. 495–504. https://doi.org/10.1145/2433396.2433457
-
Zafarani R., Liu H. Connecting users across social media sites: a behavioral-modeling approach // Proc. of the 19th ACM International Conference on Knowledge Discovery and Data Mining (SIGKDD). 2013. P. 41–49.https://doi.org/10.1145/2487575.2487648
-
Zhang H., Kan M., Liu Y., Ma S. Online social network profile linkage // Lecture Notes in Computer Science. 2014. V. 8870. P. 197–208.https://doi.org/10.1007/978-3-319-12844-3_17
-
Mu X., Zhu F., Lim E., Xiao J., Wang J., Zhou Z. User identity linkage by latent user space modelling // Proceedings of the 22nd ACM International Conference on Knowledge Discovery and Data Mining (SIGKDD). 2016. P. 1775–1784.https://doi.org/10.1145/2939672.2939849
-
Nie Y., Jia Y., Li S., Zhu X., Li A., Zhou B. Identifying users across social networks based on dynamic core interests // Neurocomputing. 2016. V. 210. P. 107–115.https://doi.org/10.1016/j.neucom.2015.10.147
-
Riederer C.J., Kim Y., Chaintreau A., Korula N., Lattanzi S. Linking users across domains with location data: Theory and validation // Proc. of the 25th International Conference on World Wide Web (WWW). 2016. P. 707–719.ttps://doi.org/10.1145/2872427.2883002
-
Chen X., Song X., Cui S., Gan T., Cheng Z., Nie L. User identity linkage across social media via attentive time-aware user modeling // IEEE Transactions on Multimedia. 2020. in press. https://doi.org/10.1109/TMM.2020.3034540
-
Nurgaliev I., Qu Q., Bamakan S.M.H., Muzammal M. Matching user identities across social networks with limited profile data // Frontiers of Computer Science. 2020. V. 14. N 6. P. 146809.https://doi.org/10.1007/s11704-019-8235-9
-
Li Y., Su Z., Yang J., Gao C. Exploiting similarities of user friendship networks across social networks for user identification // Information Sciences. 2020. V. 506. P. 78–98.https://doi.org/10.1016/j.ins.2019.08.022
-
Ma T., Guo L., Wang X., Qian Y., Tian Y., Al-Nabhan N. Friend closeness based user matching cross social networks // Mathematical Biosciences and Engineering. 2021. V. 18. N 4. P. 4264–4292. https://doi.org/10.3934/mbe.2021214
-
Dalal N., Triggs B. Histograms of oriented gradients for human detection // Proc. of the 2005 IEEE Computer Society Conference on Computer Vision and Pattern Recognition (CVPR'05). 2005. V. 1. P. 886–893. https://doi.org/10.1109/CVPR.2005.177
-
Schubert E., Sander J., Ester M., Kriegel H.-P., Xu X. DBSCAN revisited, revisited: Why and how you should (still) use DBSCAN // ACM Transactions on Database Systems. 2017. V. 42. N 3. P. 19.https://doi.org/10.1145/3068335
-
Rymarczyk T., Kozłowski E., Kłosowski G., Niderla K. Logistic regression for machine learning in process tomography // Sensors. 2019. V. 19. N 15. P. 3400. https://doi.org/10.3390/s19153400
-
Олисеенко В.Д., Абрамов М.В., Тулупьев А.Л. Идентификация аккаунтов пользователей при помощи сравнения изображений: подход на основе phash// Научно-технический вестник информационных технологий, механики и оптики. 2021. Т. 21. № 4. С. 562–570. https://doi.org/10.17586/2226-1494-2021-21-4-562-570
-
Brigham E.O. The Fast Fourier Transform. New York, USA: Prentice-Hall, 2002.
-
MacKay D.J.C.Information Theory, Inference, and Learning Algorithms. Cambridge: Cambridge University Press, 2003. 628 p.
-
Воронцов К.В. Комбинаторный подход к оценке качества обучаемых алгоритмов // Математические вопросы кибернетики.2004. T. 13. С. 5–36.