Menu
Publications
2025
2024
2023
2022
2021
2020
2019
2018
2017
2016
2015
2014
2013
2012
2011
2010
2009
2008
2007
2006
2005
2004
2003
2002
2001
Editor-in-Chief
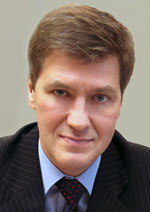
Nikiforov
Vladimir O.
D.Sc., Prof.
Partners
doi: 10.17586/2226-1494-2021-21-6-951-961
Monte Carlo Concrete DropPath for epistemic uncertainty estimation in pollen images classification
Read the full article

Article in Russian
For citation:
Abstract
For citation:
Khanzhina N.E. Monte Carlo Concrete DropPath for epistemic uncertainty estimation in pollen images classification. Scientific and Technical Journal of Information Technologies, Mechanics and Optics, 2021, vol. 21, no. 6, pp. 951–961 (in Russian). doi: 10.17586/2226-1494-2021-21-6-951-961
Abstract
The paper presents the results of a new method for training the NASNet neural network called Monte Carlo Concrete DropPath for epistemic uncertainty estimation to classify pollen images. The developed method is compared with existing methods for epistemic uncertainty estimation. The method turns an arbitrary multipath neural network into a Bayesian one by sampling from the predictive distribution. The Monte Carlo method samples different masks of DropPath to estimate uncertainty. Moreover, the probability of DropPath is optimized using continuous relaxation. The proposed method was tested for the classification task on the state-of-the-art NASNet architecture. The method demonstrated advantages on the task of classifying pollen images. The classification accuracy increased for 13 pollen species of allergen plants by 0.73 % on average compared to the baseline NASNet, reaching 98.34 % by F1 measure. Furthermore, the method increased calibration and reduced the epistemic uncertainty of the model by two times compared to the NASNets ensemble. It is shown that continuous relaxation of the DropPath probability parameter increases the accuracy of problem solving and reduces the epistemic uncertainty of the model. These results contribute to the automation of aeropalinological monitoring to reduce the time of informing patients who suffer from pollinosis and hence to prevent allergy symptoms. The developed method can be applied to train a neural network for other computer vision tasks on any image dataset.
Keywords: Bayesian deep learning, variational inference, epistemic uncertainty, pollen recognition, image recognition, uncertainty estimation
Acknowledgements. The author would like to thank Maxim Kashirin, Maxim Petukhov, Natalia Minaeva, Larisa Novoselova, Georgiy Zamorin, Tatyana Polevaya, Andrey Filchenkov, Elena Zamyatina, Irina Kharisova and Yuliya Pinaeva for their great help and useful comments.
References
Acknowledgements. The author would like to thank Maxim Kashirin, Maxim Petukhov, Natalia Minaeva, Larisa Novoselova, Georgiy Zamorin, Tatyana Polevaya, Andrey Filchenkov, Elena Zamyatina, Irina Kharisova and Yuliya Pinaeva for their great help and useful comments.
References
-
WAO White Book on Allergy. Ed by R. Pawankar, G.W. Canonica, S.T. Holgate, R.F. Lockey, M.S. Blaiss. USA, World Allergy Organization, 2013.
-
Bousquet P.J., Chinn S., Janson C., Kogevinas M., Burney P., Jarvis D. Geographical variation in the prevalence of positive skin tests to environmental aeroallergens in the European Community Respiratory Health Survey I. Allergy, 2007, vol. 62, no. 3, pp. 301–309. https://doi.org/10.1111/j.1398-9995.2006.01293.x
-
Eigenmann P.A., Eigenmann P.A., Atanaskovic-Markovic M., O'B Hourihane J., Lack G., Lau S., Matricardi P.M., Muraro A., Namazova Baranova L., Nieto A., Papadopoulos N.G., Réthy L.A., Roberts G., Rudzeviciene O., Wahn U., Wickman M., Høst A. Testing children for allergies: why, how, who and when: an updated statement of the European Academy of Allergy and Clinical Immunology (EAACI) Section on Pediatrics and the EAACI‐Clemens von Pirquet Foundation. Pediatric Allergy and Immunology, 2013, vol. 24, no. 2, pp. 195–209. https://doi.org/10.1111/pai.12066
-
Bousquet J., Schunemann H.J., Fonseca J., Samolinski B. et al. MACVIA‐ARIA Sentinel NetworK for allergic rhinitis (MASK‐rhinitis): the new generation guideline implementation. Allergy, 2015, vol. 70, no. 11, pp. 1372–1392. https://doi.org/10.1111/all.12686
-
Chikhladze M.V., Khachapuridze D.R., Sepiashvili R.I. The use of the Burkhard Pollen Trap to study the aeroecological profile in Georgia. International Journal on Immunorehabilitation, 2009, vol. 11, no. 2, pp. 200b–200b.
-
Kishikawa R., Sahashi N., Saitoh A., Kotoh E., Shimoda T., Shoji S., Akiyama K., Nishima S. Japanese cedar airborne pollen monitoring by Durham’s and burkard samplers in Japan-estimation of the usefulness of Durham’s sampler on Japanese cedar pollinosis. Global Environmental Research, 2009, vol. 13, no. 1, pp. 55–62.
-
Puc M., Kotrych D., Lipiec A., Rapiejko P., Siergiejko G. Birch pollen grains without cytoplasmic content in the air of Szczecin and Bialystok. Alergoprofil, 2016, vol. 12, no. 2, pp. 101–105.
-
Piotrowska-Weryszko K., Weryszko-Chmielewska E., Rapiejko P., Rapiejko A., Malkiewicz M., Myszkowska D., Sulborska A., Żuraw B., Chłopek K., Voloshchuk K. Mugwort pollen season in southern Poland and Lviv (Ukraine) in 2015. Alergoprofil, 2016, vol. 12, no. 1, pp. 26–30.
-
Giorato M., Bordin A., Gemignani C., Turatello F., Marcer G. Airborne pollen in Padua (NE-Italy): A comparison between two pollen samplers. Aerobiologia, 2003, vol. 19, no. 2, pp. 129–131. https://doi.org/10.1023/A:1024405624565
-
Allergenic Pollen: A Review of the Production, Release, Distribution and Health Impacts. Ed. by M. Sofiev, K.C. Bergmann. Springer, 2012, 252 p.
-
Novoselova L.V., Minaeva N. Pollen monitoring in Perm Krai (Russia)-experience of 6 years. Acta Agrobotanica, 2015, vol. 68, no. 4, pp. 343–348. https://doi.org/10.5586/aa.2015.042
-
D'Amato G., Cecchi L., Liccardi G. Thunderstorm-related asthma: not only grass pollen and spores. Journal of Allergy and Clinical Immunology, 2008, vol. 121, no. 2, pp. 537–358. https://doi.org/10.1016/j.jaci.2007.10.046
-
Sikoparija B., Skjøth C.A., Celenk S. et al. Spatial and temporal variations in airborne Ambrosia pollen in Europe. Aerobiologia, 2017, vol. 33, no. 2, pp. 181–189. https://doi.org/10.1007/s10453-016-9463-1
-
Pfaar O., Bastl K., Berger U., Buters J., Calderon M.A., Clot B., Darsow U., Demoly P., Durham S.R., Gala’n C., Gehrig R., Gerth van Wijk R., Jacobsen L., Klimek L., Sofiev M., Thibaudon M., Bergmann K.C. Definition von Pollenexpositionszeiten für klinische Studien zur Allergen-Immuntherapie bei polleninduzierter Rhinokonjunktivitis - ein EAACI-Positionspapier. Allergologie, 2018, vol. 41, no. 9, pp. 386–389. https://doi.org/10.5414/ALX02053
-
Holt K.A., Bennett K.D. Principles and methods for automated palynology. New Phytologist, 2014, vol. 203, no. 3, pp. 735–742. https://doi.org/10.1111/nph.12848
-
Flenley J.R. The problem of pollen recognition. Problems in Picture Interpretation. Ed by M.B. Clowes, J.P. Penny. CSIRO, Canberra, 1968, pp. 141–145.
-
Boucher A., Hidalgo P.J., Thonnat M., Belmonte J., Galan C., Bonton P., Tomczak R. Development of a semi-automatic system for pollen recognition. Aerobiologia, 2002, vol. 18, no. 3-4, pp. 195–201. https://doi.org/10.1023/A:1021322813565
-
Chen C., Hendriks E.A., Duin R.P.W., Reiber J.H.C., Hiemstra P.S., De Weger L.A., Stoel B.C. Feasibility study on automated recognition of allergenic pollen: grass, birch and mugwort. Aerobiologia, 2006, vol. 22, no. 4, pp. 275–284. https://doi.org/10.1007/s10453-006-9040-0
-
Ronneberger O., Schultz E., Burkhardt H. Automated pollen recognition using 3D volume images from fluorescence microscopy. Aerobiologia, 2002, vol. 18, no. 2, pp. 107–115. https://doi.org/10.1023/A:1020623724584
-
Chica M. Authentication of bee pollen grains in bright‐field microscopy by combining one‐class classification techniques and image processing. Microscopy Research and Technique, 2012, vol. 75, no. 11, pp. 1475–1485. https://doi.org/10.1002/jemt.22091
-
Chudyk C., Castaneda H., Leger R., Yahiaoui I., Boochs F. Development of an automatic pollen classification system using shape, texture and aperture features. CEUR Workshop Proceedings, 2015, vol. 1458, pp. 65–74.
-
Khanzhina N., Putin E. Pollen recognition for allergy and asthma management using gist features. Communications in Computer and Information Science, 2016, vol. 674, pp. 515–525. https://doi.org/10.1007/978-3-319-49700-6_51
-
Khanzhina N., Putin E., Filchenkov A., Zamyatina E. Pollen grain recognition using convolutional neural network. Proc. of the 26th European Symposium on Artificial Neural Networks, Computational Intelligence and Machine Learning (ESANN), 2018, pp. 409–414.
-
Krizhevsky A., Sutskever I., Hinton G.E. ImageNet classification with deep convolutional neural networks. Advances in Neural Information Processing Systems, 2012, vol. 2, pp. 1097–1105.
-
Daood A., Ribeiro E., Bush M. Classifying pollen using robust sequence alignment of sparse Z-stack volumes. Lecture Notes in Computer Science, 2016, vol. 10072, pp. 331–340. https://doi.org/10.1007/978-3-319-50835-1_31
-
Sevillano V., Aznarte J.L. Improving classification of pollen grain images of the POLEN23E dataset through three different applications of deep learning convolutional neural networks. PloS ONE, 2018, vol. 13, no. 9, pp. e0201807. https://doi.org/10.1371/journal.pone.0201807
-
Sevillano V., Holt K., Aznarte J.L. Precise automatic classification of 46 different pollen types with convolutional neural networks. Plos ONE, 2020, vol. 15, no. 6, pp. e0229751. https://doi.org/10.1371/journal.pone.0229751
-
de Geus A.R., Barcelos C.A.Z., Batista M.A., da Silva S.F. Large-scale pollen recognition with deep learning. Proc. of the 27th European Signal Processing Conference (EUSIPCO), 2019, pp. 1–5. https://doi.org/10.23919/EUSIPCO.2019.8902735
-
Gallardo-Caballero R., García-Orellana C.J., García-Manso A., González-Velasco H.M., Tormo-Molina R., Macías-Macías M. Precise pollen grain detection in bright field microscopy using deep learning techniques. Sensors, 2019, vol. 19, no. 16, pp. 3583. https://doi.org/10.3390/s19163583
-
Simonyan K., Zisserman A. Very deep convolutional networks for large-scale image recognition. Proc. of the 3rd International Conference on Learning Representations, ICLR, 2015.
-
Huang G., Liu Z., Van Der Maaten L., Weinberger K.Q. Densely connected convolutional networks. Proc. of the 30th IEEE Conference on Computer Vision and Pattern Recognition (CVPR), 2017, pp. 2261–2269. https://doi.org/10.1109/CVPR.2017.243
-
Menad H., Ben-Naoum F., Amine A. Deep convolutional neural network for pollen grains classification. CEUR Workshop Proceedings, 2019, vol. 2351.
-
Schiele J., Rabe F., Schmitt M., Glaser M., Häring F., Brunner J.O., Bauer B., Schuller B., Traidl-Hoffmann C., Damialis A. Automated classification of airborne pollen using neural networks. Proc. of the 41st Annual International Conference of the IEEE Engineering in Medicine and Biology Society (EMBC), 2019, pp. 4474–4478. https://doi.org/10.1109/EMBC.2019.8856910
-
Astolfi G., Gonçalves A.B., Menezes G.V., Borges F.S.B., Astolfi A.C.M.N., Matsubara E.T., Alvarez M., Pistori H. POLLEN73S: An image dataset for pollen grains classification. Ecological Informatics, 2020, vol. 60, pp. 101165. https://doi.org/10.1016/j.ecoinf.2020.101165
-
Gonçalves A.B., Souza J.S., Da Silva G.G., Cereda M.P., Pott A., Naka M.H., Pistori H. Feature extraction and machine learning for the classification of Brazilian Savannah pollen grains. PloS ONE, 2016, vol. 11, no. 6, pp. e0157044. https://doi.org/10.1371/journal.pone.0157044
-
Kendall A., Gal Y. What uncertainties do we need in bayesian deep learning for computer vision? Advances in Neural Information Processing Systems, 2017, vol. 30, pp. 5574–5584.
-
Gal Y., Ghahramani Z. Dropout as a bayesian approximation: Representing model uncertainty in deep learning. Proc. of the 33rd International Conference on Machine Learning (ICML),2016, pp. 1651–1660.
-
Ghiasi G., Lin T.Y., Le Q.V. DropBlock: A regularization method for convolutional networks. Advances in Neural Information Processing Systems, 2018, vol. 31, pp. 10727–10737.
-
Izmailov P., Podoprikhin D., Garipov T., Vetrov D., Wilson A.G.Averaging weights leads to wider optima and better generalization. Proc. of the 34th Conference on Uncertainty in Artificial Intelligence (UAI). Vol. 2. 2018, pp. 876–885.
-
Tompson J., Goroshin R., Jain A., LeCun Y., Bregler C. Efficient object localization using convolutional networks. Proc. of the IEEE Conference on Computer Vision and Pattern Recognition, 2015, pp. 648–656. https://doi.org/10.1109/CVPR.2015.7298664
-
Larsson G., Maire M., Shakhnarovich G. Fractalnet: Ultra-deep neural networks without residuals. Proc. of the 5th International Conference on Learning Representations (ICLR), 2017.
-
Zoph B., Vasudevan V., Shlens J., Le Q.V. Learning transferable architectures for scalable image recognition. Proc. of the 31st Meeting of the IEEE/CVF Conference on Computer Vision and Pattern Recognition (CVPR), 2018, pp. 8697–8710. https://doi.org/10.1109/CVPR.2018.00907
-
Zhang Z., Dalca A.V., Sabuncu M.R. Confidence calibration for convolutional neural networks using structured dropout. arXiv.org, 2019, arXiv:1906.09551.
-
Gal Y., Hron J., Kendall A. Concrete dropout. Advances in Neural Information Processing Systems, 2017, vol. 30, pp. 3582–3591.
-
Maddison C.J., Mnih A., Teh Y.W. The concrete distribution: A continuous relaxation of discrete random variables. Proc. of the 5th International Conference on Learning Representations (ICLR), 2017.
-
Szegedy C., Ioffe S., Vanhoucke V., Alemi A.A. Inception-v4, inception-resnet and the impact of residual connections on learning. Proc. of the 31st AAAI Conference on Artificial Intelligence, 2017, pp. 4278–4284.
-
Xie S., Girshick R., Dollár P., Tu Z., He K. Aggregated residual transformations for deep neural networks. Proc. of the 30th IEEE Conference on Computer Vision and Pattern Recognition (CVPR), 2017, pp. 5987–5995. https://doi.org/10.1109/CVPR.2017.634
-
Khanzhina N., Kashirin M., Filchenkov A. Monte Carlo concrete DropPath for epistemic uncertainty estimation in brain tumor segmentation. Lecture Notes in Computer Science, 2021, vol. 12959, pp. 64–74. https://doi.org/10.1007/978-3-030-87735-4_7
-
Karras T., Laine S., Aila T. A style-based generator architecture for generative adversarial networks. Proc. of the 32nd IEEE/CVF Conference on Computer Vision and Pattern Recognition (CVPR), 2019, pp. 4396–4405. https://doi.org/10.1109/CVPR.2019.00453
-
Karras T., Aila T., Laine S., Lehtinen J.Progressive growing of GANs for improved quality, stability, and variation. Proc. of the 6th International Conference on Learning Representations (ICLR), 2018.
-
Huang X., Belongie S. Arbitrary style transfer in real-time with adaptive instance normalization. Proc. of the 16th IEEE International Conference on Computer Vision (ICCV), 2017, pp. 1510–1519. https://doi.org/10.1109/ICCV.2017.167
-
Loquercio A., Segu M., Scaramuzza D. A general framework for uncertainty estimation in deep learning. IEEE Robotics and Automation Letters, 2020, vol. 5, no. 2, pp. 3153–3160. https://doi.os in Neural Information Processing Systems, 2019, vol. 32.
-
Naeini M.P., Cooper G., Hauskrecht M. Obtaining well calibrated probabilities using bayesian binning. Proc. of the 29th AAAI Conference on Artificial Intelligence, 2015, pp. 2901–2907.
-
Ashukha A., Lyzhov A., Molchanov D., Vetrov D. Pitfalls of in-domain uncertainty estimation and ensembling in deep learning. arXiv.org, 2020, arXiv:2002.06470.