Menu
Publications
2025
2024
2023
2022
2021
2020
2019
2018
2017
2016
2015
2014
2013
2012
2011
2010
2009
2008
2007
2006
2005
2004
2003
2002
2001
Editor-in-Chief
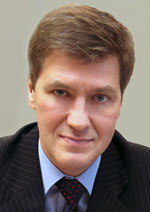
Nikiforov
Vladimir O.
D.Sc., Prof.
Partners
doi: 10.17586/2226-1494-2022-22-1-179-186
A mathematical model of an epidemic with an arbitrary law of recovery
Read the full article

Article in Russian
For citation:
Abstract
For citation:
Semenov V.K., Ivanova N.B. A mathematical model of an epidemic with an arbitrary law of recovery. Scientific and Technical Journal of Information Technologies, Mechanics and Optics, 2022, vol. 22, no. 1, pp. 179–186 (in Russian). doi: 10.17586/2226-1494-2022-22-1-179-186
Abstract
The paper proposes a mathematical model of the epidemic process, taking into account the dependence of the rates of cure and loss of immunity on time. Today, mathematical models of epidemics based on the basic Kermack–McKendrick model have become widespread. The most famous models are Susceptible-Infected-Recovered (SIR) and Susceptible-Exposed-Infected-Recovered (SEIR). These models are based on dividing the population into separate groups that are in different epidemic conditions. The description of the models is based on differential equations similar to the equations of birth and death in the process of radioactive transformations of elements in a radioactive chain. However, this approach does not take into account the dependence of the probabilities of the transition of the population from group to group on time spent in the treatment process and in the process of loss of acquired immunity. The known models do analyze the nature of the course of the epidemic for long periods of time, when the process can enter a stationary state. The paper proposes a mathematical model based on dividing the population into separate groups. The first group consists of healthy people susceptible to infection due to contact with members of the second group, which includes the infected population. Members of the third group are being treated, the fourth group includes members of the society who have recovered with antibodies and are vaccinated. The fifth group consists of deceased members of society. In contrast to the SIR and SEIR models, the proposed approach takes into account that immunity is lost over time, and people who survived again move to the group susceptible to infection. The dependences of the probabilities of transition from group to group on time spent both during the treatment process and in the loss of acquired immunity have been taken into account. Thus, the proposed mathematical model is based on five integro-differential equations, two of which are partial differential equations. A new mathematical model has been formulated that makes it possible to take into account the dependence of the cure rate and the probability of transition from the vaccinated state to the initial state on time spent in the corresponding state. It is shown that the proposed model is autocatalytic. With increasing time, a state of bistability is observed, when, under the same boundary conditions, two stable states coexist. Switching between states is determined by the epidemic spread control parameter found in the work. One of the stable states is stationary and leads to the end of the epidemic, the second one leads to the population’s extinction. It has been shown that, for the stationary regime, the form of the distribution function in terms of treatment time and exposure time in the vaccinated state does not affect the final result in any way. The conditions for suppressing the epidemic for managing the process of its development are formulated. Numerical experiments were carried out to simulate the epidemic spreading process, taking into account the constancy of all transition probabilities. Integration of the original system of equations was carried out using the Radaus algorithm for stiff differential equations. The results of numerical simulations have confirmed that the experimental data agree with the theory of the control parameter. The results of the work can be used to organize the management of the epidemic spreading process in order to suppress it as soon as possible by changing the value of the control parameter.
Keywords: epidemic, mathematical model, distribution density over recovery time, bistable state, control parameter, suppression conditions
References
References
-
Koltsova E.M., Kurkina E.S., Vasetsky A.M. Mathematical modeling of the spread of COVID-19 in Moscow. Computational nanotechnology, 2020, vol. 7, no. 1, pp. 99–105. (in Russian). https://doi.org/10.33693/2313-223X-2020-7-1-99-105
-
Getz W.M., Dougherty E.R. Discrete stochastic analogs of Erlang epidemic models. Journal of Biological Dynamics, 2018, vol. 12, no. 1, pp. 16–38. https://doi.org/10.1080/17513758.2017.1401677
-
Verity R., Okell L.C., Dorigatti I., Winskill P., Whittaker C., Imai N., Cuomo-Dannenburg G., Thompson H., Walker P.G.T., Fu H., Dighe A., Griffin J.T., Baguelin M., Bhatia S., Boonyasiri A., Cori A., Cucunubá Z., FitzJohn R., Gaythorpe K., Green W., Hamlet A., Laydon D., Nedjati-Gilani G., Riley S., van Elsland S., Volz E., Wang H., Wang Y., Xi X., Donnelly C.A., Ghani A.C., Ferguson N.M. Estimates of the severity of coronavirus disease 2019: a model-based analysis. The Lancet Infectious Diseases, 2020, vol. 20, no. 6, pp. 669–677. https://doi.org/10.1016/S1473-3099(20)30243-7
-
Kucharski A.J., Russell T.W., Diamond C., Liu Y., Edmunds J., Funk S., Eggo R.M., Sun F., Jit M., Munday J.D., Davies N., Gimma A., van Zandvoort K., Gibbs H., Hellewell J., Jarvis C.I., Clifford S., Quilty B.J., Bosse N.I., Abbott S., Klepac P., Flasche S. Early dynamics of transmission and control of COVID-19: a mathematical modelling study. The Lancet Infectious Diseases, 2020, vol. 20, no. 5, pp. 553–558. https://doi.org/10.1016/S1473-3099(20)30144-4
-
Li R., Pei S., Chen B., Song Y., Zhang T., Yang W., Shaman J. Substantial undocumented infection facilitates the rapid dissemination of novel coronavirus (SARS-CoV-2). Science, 2020, vol. 368, no. 6490, pp. 489–493. https://doi.org/10.1126/science.abb3221
-
Liu Y., Gayle A.A., Wilder-Smith A., Rocklöv J. The reproductive number of COVID-19 is higher compared to SARS coronavirus. Journal of Travel Medicine, 2020, vol. 27, no. 2, pp. taaa021. https://doi.org/10.1093/jtm/taaa021
-
Singh S., Parmar K.S., Kumar J., Makkhan S.J.S. Development of new hybrid model of discrete wavelet decomposition and autoregressive integrated moving average (ARIMA) models in application to one month forecast the casualties cases of COVID-19. Chaos, Solitons & Fractals, 2020, vol. 135, pp. 109866. https://doi.org/10.1016/j.chaos.2020.109866
-
Chakraborty T., Ghosh I. Real-time forecasts and risk assessment of novel coronavirus (COVID-19) cases: A data-driven analysis. Chaos, Solitons & Fractals, 2020, vol. 135, pp. 109850. https://doi.org/10.1016/j.chaos.2020.109850
-
Srivastava A., Prasanna V.K. Learning to forecast and forecasting to learn from the COVID-19 PANDEMIC. 2020.Available at: https://arxiv.org/pdf/2004.11372.pdf(accessed: 01.12.2021).
-
Perone G. ARIMA forecasting of COVID-19 incidence in Italy, Russia, and the USA. 2020. Available at: https://arxiv.org/pdf/2006.01754.pdf (accessed: 01.12.2021).
-
Trubetskov D.I. Phenomenon of Lotka-Volterra mathematical model and similar models. Izvestiya Vysshikh Uchebnykh Zavedeniy. Prikladnaya Nelineynaya Dinamika, 2011, vol. 19, no. 2, pp. 69–88. (in Russian). https://doi.org/10.18500/0869-6632-2011-19-2-69-88
-
Tomchin D., Fradkov A. Prediction of the virus COVID-19 spread in Russia based on simple mathematical models of epidemics. 2020. Available at: http://onr-russia.ru/sites/default/files/tf20-5.pdf(accessed: 01.11.2021). (in Russian)
-
Krivorot’ko O.I., Kabanikhin S.I., Zyat’kov N.Y., Prikhod’ko A.Y., Shishlenin M.A., Prokhoshin N.M. Mathematical modeling and forecasting of COVID-19 in Moscow and Novosibirsk Region. Numerical Analysis and Applications, 2020, vol. 13, no. 4, pp. 332–348. https://doi.org/10.1134/S1995423920040047
-
Ed Fontes. Modeling the Spread of COVID-19 with COMSOL Multiphysics®. Available at: https://www.comsol.ru/blogs/modeling-the-spread-of-covid-19-with-comsol-multiphysics/(accessed: 01.11.2021).
-
Weiss H. The SIR model and the Foundations of Public Health. MATerials MATemàtics, 2013, vol. 2013, no. 3, pp. 17. Available at: https://mat.uab.cat/matmat_antiga/PDFv2013/v2013n03.pdf (accessed: 01.11.2021).
-
Barmparis G.D., Tsironis G.P. Estimating the infection horizon of COVID-19 in eight countries with a data-driven approach. Chaos, Solitons & Fractals, 2020, vol. 135, pp. 109842. https://doi.org/10.1016/j.chaos.2020.109842
-
Teles P. A time-dependent SEIR model to analyse the evolution of the SARS-CoV-2 epidemic outbreak in Portugal. 2020. Available at: https://arxiv.org/pdf/2004.04735.pdf(accessed: 01.12.2021).