Menu
Publications
2024
2023
2022
2021
2020
2019
2018
2017
2016
2015
2014
2013
2012
2011
2010
2009
2008
2007
2006
2005
2004
2003
2002
2001
Editor-in-Chief
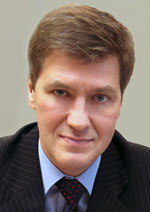
Nikiforov
Vladimir O.
D.Sc., Prof.
Partners
doi: 10.17586/2226-1494-2022-22-2-254-261
Research on the effectiveness of noise reduction when encoding a lossless speech signal
Read the full article

Article in English
For citation:
Abstract
For citation:
Akilan T., Raja L., Hariharan U. Research on the effectiveness of noise reduction when encoding a lossless speech signal. Scientific and Technical Journal of Information Technologies, Mechanics and Optics, 2022, vol. 22, no. 2, pp. 254–261. doi: 10.17586/2226-1494-2022-22-2-254-261
Abstract
In the meantime, speech coding is one of the methods to represent the digital speech signal as in possible fewer bits value and to maintain the quality and its clearness. In omnipresent situations, encryption and examination of speech maintain a crucial role in various acoustic-based coding systems. This paper, using subband and Huffman coding technique, has been used for speech signals description to reduce the occupied by the speech data memory. The amplitude values of the taken speech are segregated after pre-processing, windowing and decomposition techniques. These data are converted into the frequency domain using discrete cosine transform (DCT). Then 90 foremost coefficients have been coded by Huffman method, they contain the most valuable information of speech signals. Signals are segregated then and subband coding techniques applied. To reconstruct the input speech, the taken speech is re-transformed in the form of time-domain applying through inverse discrete cosine transform (IDCT). This experiment is carried out by speech data at 8 kHz with 16 bits/per sample. The SNR (Signal to Noise Ratio) shows the efficiency of this applied technique.
Keywords: decomposition, IDCT, DCT, Huffman, SNR, subband, quantization, windowing
References
References
-
Lv S., Hu Y., Zhang S., Xie L. DCCRN+: channel-wise subband DCCRN with SNR estimation for speech enhancement. Proc. of the 22nd Annual Conference of the International Speech Communication Association (INTERSPEECH), 2021, pp. 2816–2820. https://doi.org/10.21437/Interspeech.2021-1482
-
Taujuddin N.S.A.M., Ibrahim R., Sari S. Image compression using a new adaptive standard deviation thresholding estimation at the wavelet details subbands. Proc. of the 2nd International Conference on Computing Technology and Information Management (ICCTIM), 2015, pp. 109–114. https://doi.org/10.1109/ICCTIM.2015.7224602
-
Pal R. Speech compression with wavelet transform and huffman coding. Proc. of the 4th International Conference on Communication, Information and Computing Technology (ICCICT), 2021, pp. 1–4. https://doi.org/10.1109/ICCICT50803.2021.9510116
-
Li S., Zheng Z., Dai W., Xiong H. Lossy image compression with filter bank based convolutional networks. Proc. of the Data Compression Conference (DCC), 2019, pp. 23–32. https://doi.org/10.1109/DCC.2019.00010
-
Cooper C., Marcellin M. Lossless wideband RF compression via lifting-based IIR subband decomposition. IEEE Transactions on Aerospace and Electronic Systems, 2020, vol. 56, no. 1, pp. 823–829. https://doi.org/10.1109/TAES.2019.2919436
-
Vatsa S., Dr. Sahu O.P. Speech compression using discrete wavelet transform and discrete cosine transform. International Journal of Engineering Research & Technology (IJERT), 2012, vol. 1, no. 5, pp. 1–6.
-
Balaji V.R., Subramanian S. A novel speech enhancement approach based on modified DCT and improved pitch synchronous analysis. American Journal of Applied Sciences, 2014, vol. 11, no. 1, pp. 24–37. https://doi.org/10.3844/ajassp.2014.24.37
-
Vats S., Rathee G. An image-compression decomposition analysis of sub-bands using threshold implementation. Proc. of the 3rd International Conference on Image Information Processing (ICIIP), 2015, pp. 366–369. https://doi.org/10.1109/ICIIP.2015.7414797
-
Luneau J.-M., Lebrun J., Jensen S.H. Complex wavelet modulation subbands for speech compression. Proc. of the Data Compression Conference (DCC), 2009, pp. 457. https://doi.org/10.1109/DCC.2009.52
-
Mack W., Habets E.A.P. Deep filtering: Signal extraction and reconstruction using complex time-frequency filters. IEEE Signal Processing Letters, 2020, vol. 27, pp. 61–65. https://doi.org/10.1109/LSP.2019.2955818