Menu
Publications
2025
2024
2023
2022
2021
2020
2019
2018
2017
2016
2015
2014
2013
2012
2011
2010
2009
2008
2007
2006
2005
2004
2003
2002
2001
Editor-in-Chief
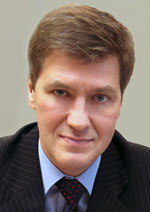
Nikiforov
Vladimir O.
D.Sc., Prof.
Partners
doi: 10.17586/2226-1494-2022-22-3-492-500
DC motor fault detection and isolation scheme with the use of directional residual set
Read the full article

Article in Russian
For citation:
Abstract
For citation:
Kolesnik N.S., Margun A.A. DC motor fault detection and isolation scheme with the use of directional residual set. Scientific and Technical Journal of Information Technologies, Mechanics and Optics, 2022, vol. 22, no. 3, pp. 492–500 (in Russian). doi: 10.17586/2226-1494-2022-22-3-492-500
Abstract
The subject of research is presented as online-estimation of characteristics of DC motors under various loads. The paper is devoted to a modern approach to solve the problem of detecting DC motor failures. Proposed detection method is based on the set of full state Luenberger observers. Isolation scheme uses directional residual set and relationships between fault direction and residual vector. The procedure of synthesizing the fault detection and isolation algorithm for DC motor is designed. This scheme performance is proved with computer modeling of typical DC motor RK 370CA with faults caused by unaccounted force momentum acting on rotor, input voltage disturbance, velocity and current sensors failures. The algorithm correctly defines motor state (fault presence or absence) and also properly isolates fault cause. Proposed method advantage is compared to other solutions based on hardware and timing redundancy, identification and observers lies in the opportunity to detect and isolate faults of input and output signals with trivial synthesis and absence of the need to expand system hardware. Proposed method is applicable to any second order system, and also there is a possibility to use it for higher order systems with the corresponding changing of the equation systems solving for observer synthesis. This algorithm allows realizing online fault isolation and does not require additional measuring which promotes decrease of diagnostic costs, repair and serving time saving, modern accident detection. The results can be applied to DC motor control to increase reliability and to develop DC motor control systems.
Keywords: failure detection, failure isolation, DC motor, directional residual generator
Acknowledgements. Research has been partially made with support of the Ministry of Science and Higher Education of the Russian Federation, government order № 2019-0898; this work has been partially completed in IPME RAS with support of government order № 121112500298-6 (USAIS RDTCW).
References
Acknowledgements. Research has been partially made with support of the Ministry of Science and Higher Education of the Russian Federation, government order № 2019-0898; this work has been partially completed in IPME RAS with support of government order № 121112500298-6 (USAIS RDTCW).
References
-
Chen J., Patton R.J. Robust Model-Based Fault Diagnosis for Dynamic Systems. Springer Science & Business Media, 2012, 356 p.
-
Wünnenberg J. Observer-based Fault Detection in Dynamic Systems. 1990, VDI Verlag, 127 p.
-
Averyanov A.V., Baranovsky A.M., Esaulov K. Analysis of hardware redundancy limits of the system of automated remote management, military. I-METHODS, 2015, vol. 7, no. 4, pp. 16–20. (in Russian)
-
Chen J., Patton R., Zhang H.-Y. Design of unknown input observers and robust fault detection filters. International Journal of Control, 1996, vol. 63, no. 1, pp. 85–105. https://doi.org/10.1080/00207179608921833
-
Kozlov D.S., Tiumentsev Y.V. Neural network techniques for fault identification of aircraft sensors and actuators. Trudy MAI, 2019, no. 52, pp. 2. (in Russian)
-
Korovkin M.V., Sotnikova M.V. Failure detection and localization in the control systems of marine mobile objects. Sistemy upravlenija i informacionnye tehnologii, 2013, no. 4, pp. 91–94. (in Russian)
-
Oh K.S., Song T.J., Lee J.M., Yoon Y.M., Yi K.S. Sliding mode observer based fault detection and isolation algorithm of sensor for longitudinal autonomous driving using V2V communication. Transactions of the Korean Society of Mechanical Engineers, A, 2019, vol. 43, no. 98, pp. 599–609. (in Korean). https://doi.org/10.3795/KSME-A.2019.43.9.599
-
Zhao R., Wu Y. Intelligent fault detection and diagnosis method for automatic control system based on reinforcement learning. Lecture Notes in Electrical Engineering, 2021, vol. 747, pp. 1459–1467. https://doi.org/10.1007/978-981-16-0115-6_167
-
Zhang Y., Wang K., Qian X., Gendeel M. Robust fault-detection based on residual K–L divergence for wind turbines. IET Renewable Power Generation, 2019, vol. 13, no. 13, pp. 2400–2408. https://doi.org/10.1049/iet-rpg.2018.6190
-
Lim J.K., Park C.G. Satellite fault detection and isolation scheme with modified adaptive fading EKF. Journal of Electrical Engineering and Technology, 2014, vol. 9, no. 4, pp. 1401–1410. https://doi.org/10.5370/jeet.2014.9.4.1401
-
Zakharov N., Kalin S., Klepikov V., Podkhvatilin D. Algorithmic providing of fault tolerance of the distributed controlling systems. Radioelectronic and Computer Systems, 2008, no. 7(34), pp. 43–48. (in Russian)
-
Leibov R.L. Control system actuator failures detection, isolation and concrete definition. Aerospace Instrument-Making, 2012, no. 8, pp. 26–35. (in Russian)
-
Kuznetsova T.A., Augustinovich V.G. The neural network application for fault diagnostics of feedback channels’ sensor in automatic control systems of modern aircraft gas-turbine engines. Scientific and Technical Volga region Bulletin, 2017, no. 4, pp. 131–133. (in Russian). https://doi.org/10.24153/2079-5920-2017-7-4-131-133
-
Adouni A., Abid A., Sbita L. A DC motor fault detection, isolation and identification based on a new architecture Artificial Neural Network. Proc. of the 5th International Conference on Systems and Control (ICSC), 2016, pp. 294–299. https://doi.org/10.1109/ICoSC.2016.7507054
-
Santos L.I., Palhares R., D’Angelo M., Mendes J.B., Veloso R., Ekel P. A new scheme for fault detection and classification applied to dc motor. Trends in Computational and Applied Mathematics, 2018, vol. 19, no. 2, pp. 327–345. https://doi.org/10.5540/tema.2018.019.02.327