Menu
Publications
2025
2024
2023
2022
2021
2020
2019
2018
2017
2016
2015
2014
2013
2012
2011
2010
2009
2008
2007
2006
2005
2004
2003
2002
2001
Editor-in-Chief
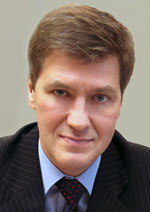
Nikiforov
Vladimir O.
D.Sc., Prof.
Partners
doi: 10.17586/2226-1494-2022-22-5-941-950
An enforced non-negative matrix factorization based approach towards community detection in dynamic networks
Read the full article

Article in English
For citation:
Abstract
For citation:
Bashir S., Chachoo M.A. An enforced non-negative matrix factorization based approach towards community detection in dynamic networks. Scientific and Technical Journal of Information Technologies, Mechanics and Optics, 2022, vol. 22, no. 5, pp. 941–950. doi: 10.17586/2226-1494-2022-22-5-941-950
Abstract
Identifying community structures within network dynamics is important for analysing the latent structure of the network, understanding the functions of the network, predicting the evolution of the network as well as detecting unusual events of the network. From various perspectives, a diversity of approaches towards dynamic community detection has been advised. However, owing to the difficulty in parameter adjustment, high temporal complexity and detection accuracy is diminishing as time slice rises; and recognizing the community composition in dynamic networks gets extremely complex. The basic models, principles, qualities, and techniques of latent factor models, as well as their various modifications, generalizations and extensions, are summed up systematically in this study which focuses on both theoretical and experimental research into latent factor models across the latest ten years. Latent factor model like non-negative matrix factorization is considered one of the most successful models for community identification which aims to uncover distributed lower dimension representation so as to reveal community node membership. These models are mostly centred on reconstructing the network from node representations while requiring the representation to have special desirable qualities (non-negativity). The purpose of this work is to provide an experimental as well as theoretical comparative analysis of the latent factor approaches employed to detect communities within dynamic networks. Parallelly we have devised the generic and improved non-negative matrix factorization-based model which will help in producing robust community detection results in dynamic networks. The results have been calculated from the experiments done in Python. Moreover our models methodology focuses on information dynamics so as to quantify the information propagation among the involved nodes unlike existing methods that considers networks first-order topological information described by its adjacency matrix without considering the information propagation between the nodes. In addition, this paper intends to create a unified, state of the art framework meant for non-negative matrix factorization conception which could be useful for future study.
Keywords: community detection, principal component analysis, orthogonality, non-negative matrix factorization, singular value decomposition, social network analysis
References
References
-
Girvan M., Newman M.E.J. Community structure in social and biological networks. Proceedings of the National Academy of Sciences of the United States of America, 2002, vol. 99, no. 12, pp. 7821–7826. https://doi.org/10.1073/pnas.122653799
-
Wang Y., Zhang Y. Nonnegative Matrix Factorization: A comprehensive review. IEEE Transactions on Knowledge and Data Engineering, 2013, vol. 25, no. 6, pp. 1336–1353. https://doi.org/10.1109/TKDE.2012.51
-
Yang J., Leskovec J. Overlapping community detection at scale: A nonnegative matrix factorization approach. Proc. of the 6th ACM International Conference on Web Search and Data Mining (WSDM), 2013, pp. 587–596. https://doi.org/10.1145/2433396.2433471
-
Wang F., Li T., Wang X., Zhu S., Ding C. Community discovery using nonnegative matrix factorization. Data Mining and Knowledge Discovery, 2011, vol. 22, no. 3, pp. 493–521. https://doi.org/10.1007/s10618-010-0181-y
-
Gao F., Yuan L., Wang W. Dynamic community detection using nonnegative matrix factorization. Proc. of the International Conference on Computing Intelligence and Information System (CIIS), 2017, pp. 39–45. https://doi.org/10.1109/CIIS.2017.56
-
Ye Z.,Zhang H.,Feng L., Shan Z. CDCN: A new NMF-based community detection method with community structures and node attributes.Wireless Communications and Mobile Computing, 2021, pp. 5517204. https://doi.org/10.1155/2021/5517204
-
Yang K., Guo Q., Liu J.Q. Community detection via measuring the strength between nodes for dynamic networks. Physica A: Statistical Mechanics and its Applications, 2018, vol. 509, pp. 256–264. https://doi.org/10.1016/j.physa.2018.06.038
-
Lin Y.R., Chi Y., Zhu S., Sundaram H., Tseng B.L. Facetnet: A framework for analyzing communities and their evolutions in dynamic networks. Proc. of the 17th International Conference on World Wide Web, 2008, pp. 685–694. https://doi.org/10.1145/1367497.1367590
-
Lu H., Sang X., Zhao Q., Lu J. Community detection algorithm based on nonnegative matrix factorization and pairwise constraints. Physica A: Statistical Mechanics and its Applications, 2020, vol. 545, pp. 123491. https://doi.org/10.1016/j.physa.2019.123491
-
Lee D., Seung H.S. Learning the parts of objects with non-negative matrix factorization. Nature, 1999, vol. 401, no. 6755, pp. 788–791. https://doi.org/10.1038/44565
-
Cai D., He X., Han J., Huang T.S. Graph regularized nonnegative matrix factorization for data representation. IEEE Transactions on Pattern Analysis and Machine Intelligence, 2011, vol. 33, no. 8, pp. 1548–1560. https://doi.org/10.1109/TPAMI.2010.231
-
Chung F.R.K. Spectral Graph Theory. Published for the Conference Board of the mathematical sciences by the American Mathematical Society, 1997, 108 p.
-
Yu W., Wu H., Jiao P., Wu H., Sun Y., Tang M. Modeling the local and global evolution pattern of community structures for dynamic networks analysis. IEEE Access, 2019, vol. 7, pp. 71350–71360. https://doi.org/10.1109/ACCESS.2019.2920237
-
Shafia, Chachoo M.A. Social network analysis based criminal community identification model with community structures and node attributes. Proc. of the 4th International Conference on Smart Systems and Inventive Technology (ICSSIT), 2022,pp. 334–339. https://doi.org/10.1109/ICSSIT53264.2022.9716286
-
Ma X., Dong D. Evolutionary nonnegative matrix factorization algorithms for community detection in dynamic networks. IEEE Transactions on Knowledge & Data Engineering, 2017, vol. 29, no. 5, pp. 1045–1058. https://doi.org/10.1109/TKDE.2017.2657752
-
Jiao P., Yu W., Wang W., Li X., Sun Y. Exploring temporal community structure and constant evolutionary pattern hiding in dynamic networks. Neurocomputing, 2018, vol. 314, pp. 224–233. https://doi.org/10.1016/j.neucom.2018.03.065
-
Liu H.-F., Yuan L.-M.-Z. Community detection in temporal networks using triple nonnegative matrix factorization. DEStech Transactions on Computer Science and Engineering, 2017. https://doi.org/10.12783/dtcse/mmsta2017/19682
-
Wang T., Liu Y., Xi Y.-Y. Identifying community in bipartite networks using graph regularized-based non-negative matrix factorization. Journal of Electronics and Information Technology, 2015, vol. 37, no. 9, pp. 2238–2245. (in Chinese). https://doi.org/10.11999/JEIT141649
-
Newman M.E.J. Modularity and community structure in networks. Proceedingsof the National Academy of Sciences of the United States of America, 2006, vol. 103, no. 23, pp. 8577–8582. https://doi.org/10.1073/pnas.0601602103
-
Blondel V.D., Guillaume J.L., Lambiotte R; Lefebvre E. Fast unfolding of communities in large networks. Journal of Statistical Mechanics: Theory and Experiment, 2008, pp. P10008. https://doi.org/10.1088/1742-5468/2008/10/P10008
-
Nguyen N.P., Dinh T.N., Tokala S., Thai M.T. Overlapping communities in dynamic networks: their detection and mobile applications. Proc. of the 17th Annual International Conference on Mobile Computing and Networking, MobiCom'11 and Co-Located Workshops, 2011, pp. 85–95. https://doi.org/10.1145/2030613.2030624
-
He D., Jin D., Baquero C., Liu D. Link community detection using generative model and nonnegative matrix factorization. PLoS ONE, 2014, vol. 9, no. 1, pp. e86899. https://doi.org/10.1371/journal.pone.0086899
-
Bashir S., Chachoo M.A. Community detection in online social networks: models and methods, a survey. Gedrag & Organisatie Review, 2020, vol. 33, pp. 1164–1175. https://doi.org/10.37896/gor33.03/498
-
Wang S., Li G., Hu G., Wei H., Pan Y., Pan Z. Community detection in dynamic networks using constraint non-negative matrix factorization. Intelligent Data Analysis, 2020, vol. 24, no. 1, pp. 119–139. https://doi.org/10.3233/IDA-184432
-
Lu H., Zhao Q., Sang X., Lu J. Community detection in complex networks using nonnegative matrix factorization and density-based clustering algorithm. Neural Processing Letters, 2020, vol. 51, no. 2, pp. 1731–1748. https://doi.org/10.1007/s11063-019-10170-1
-
Zachary W.W. An information flow model for conflict and fission in small groups. Journal of Anthropological Research, 1977, vol. 33, no. 4, pp. 452–473. https://doi.org/10.1086/jar.33.4.3629752
-
Lusseau D., Schneider K., Boisseau O.J., Haase P., Slooten E., Dawson S.M. The bottlenose dolphin community of doubtful sound features a large proportion of long-lasting associations. Behavioral Ecology and Sociobiology, 2003, vol. 54, no. 4, pp. 396–405. https://doi.org/10.1007/s00265-003-0651-y
-
McAuley J., Leskovec J. Learning to discover social circles in ego networks. Advances in Neural Information Processing Systems, 2012, vol. 1, pp. 539–547.