Menu
Publications
2024
2023
2022
2021
2020
2019
2018
2017
2016
2015
2014
2013
2012
2011
2010
2009
2008
2007
2006
2005
2004
2003
2002
2001
Editor-in-Chief
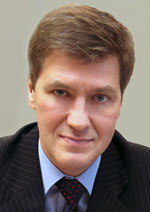
Nikiforov
Vladimir O.
D.Sc., Prof.
Partners
doi: 10.17586/2226-1494-2023-23-1-97-104
Multiobjective evolutionary discovery of equation-based analytical models for dynamical systems
Read the full article

Article in English
For citation:
Abstract
For citation:
Maslyaev M.A., Hvatov A.A. Multiobjective evolutionary discovery of equation-based analytical models for dynamical systems. Scientific and Technical Journal of Information Technologies, Mechanics and Optics, 2023, vol. 23, no. 1, pp. 97–104. doi: 10.17586/2226-1494-2023-23-1-97-104
Abstract
In this article, an approach to modeling dynamical systems in case of unknown governing physical laws has been introduced. The systems of differential equations obtained by means of a data-driven algorithm are taken as the desired models. In this case, the problem of predicting the state of the process is solved by integrating the resulting differential equations. In contrast to classical data-driven approaches to dynamical systems representation, based on the general machine learning methods, the proposed approach is based on the principles, comparable to the analytical equation-based modeling. Models in forms of systems of differential equations, composed as combinations of elementary functions and operation with the structure, were determined by adapted multi-objective evolutionary optimization algorithm. Time-series describing the state of each element of the dynamic system are used as input data for the algorithm. To ensure the correct operation of the algorithm on data characterizing real-world processes, noise reduction mechanisms are introduced in the algorithm. The use of multicriteria optimization, held in the space of complexity and quality criteria for individual equations of the differential equation system, makes it possible to improve the diversity of proposed candidate solutions and, therefore, to improve the convergence of the algorithm to a model that best represents the dynamics of the process. The output of the algorithm is a set of Pareto-optimal solutions of the optimization problem where each individual of the set corresponds to one system of differential equations. In the course of the work, a library of data-driven modeling of dynamic systems based on differential equation systems was created. The behavior of the algorithm was studied on a synthetic validation dataset describing the state of the hunter-prey dynamic system given by the Lotka-Volterra equations. Finally, a toolset based on the solution of the generated equations was integrated into the algorithm for predicting future system states. The method is applicable to data-driven modeling of arbitrary dynamical systems (e.g. hydrometeorological systems) in cases where the processes can be described using differential equations. Models generated by the algorithm can be used as components of more complex composite models, or in an ensemble of methods as an interpretable component.
Keywords: differential equation discovery, evolutionary optimization, multi-objective optimization, differential equations system, symbolic regression
Acknowledgements. This research is financially supported by the Russian Scientific Foundation, Agreement No. 21-71-00128.
References
Acknowledgements. This research is financially supported by the Russian Scientific Foundation, Agreement No. 21-71-00128.
References
-
Bubnova A.V., Deeva I., Kalyuzhnaya A.V. MIxBN: library for learning Bayesian networks from mixed data. Procedia Computer Science, 2021, vol. 193, pp. 494–503. https://doi.org/10.1016/j.procs.2021.10.051
-
Maslyaev M., Hvatov A. Solver-based fitness function for the data-driven evolutionary discovery of partial differential equations. Proc. of the 2022 IEEE Congress on Evolutionary Computation (CEC), 2022. https://doi.org/10.1109/cec55065.2022.9870370
-
Brunton S.L., Brunton B.W., Proctor J.L., Kaiser E., Kutz J.N. Chaos as an intermittently forced linear system. Nature Communications, 2017, vol. 8, no. 1, pp. 19. https://doi.org/10.1038/s41467-017-00030-8
-
Schmid P.J., Sesterhenn J. Dynamic mode decomposition of numerical and experimental data. Proc. of the 61st Annual Meeting of the APS Division of Fluid Dynamics. American Physical Society, November 2008.
-
Kondrashov D., Chekroun M.D., Ghil M. Data-driven non-Markovian closure models. Physica D: Nonlinear Phenomena, 2015, vol. 297, pp. 33–55. https://doi.org/10.1016/j.physd.2014.12.005
-
Schmidt M., Lipson H. Distilling free-form natural laws from experimental data.Science, 2009, vol. 324, no. 5923, pp. 81–85. https://doi.org/10.1126/science.1165893
-
Kaheman K., Kutz J.N., Brunton S.L. SINDy-PI: a robust algorithm for parallel implicit sparse identification of nonlinear dynamics. Proceedings of the Royal Society A: Mathematical, Physical and Engineering Sciences, 2020, vol. 476, no. 2242, pp. 20200279. https://doi.org/10.1098/rspa.2020.0279
-
Berg J., Nyström K. Data-driven discovery of PDEs in complex datasets. Journal of Computational Physics, 2019, vol. 384, pp. 239–252. https://doi.org/10.1016/j.jcp.2019.01.036
-
Han G., Zahr M.J., Wang J.-X. Physics-informed graph neural Galerkin networks: A unified framework for solving PDE-governed forward and inverse problems. Computer Methods in Applied Mechanics and Engineering, 2022, vol. 390, pp. 114502. https://doi.org/10.1016/j.cma.2021.114502
-
Long Z., Lu Y., Ma X., Dong B. PDE-Net: learning PDEs from data. Proceedings of Machine Learning Research, 2018, vol. 80, pp. 3208–3216.
-
Raissi M. Deep hidden physics models: Deep learning of nonlinear partial differential equations. Journal of Machine Learning Research, 2018, vol. 19, pp. 1–24.
-
Zhang J., Ma W. Data-driven discovery of governing equations for fluid dynamics based on molecular simulation. Journal of Fluid Mechanics, 2020, vol. 892, pp. A5. https://doi.org/10.1017/jfm.2020.184
-
Van Breugel F., Kutz J.N., Brunton B.W. Numerical differentiation of noisy data: A unifying multi-objective optimization framework. IEEE Access, 2020, vol. 8, pp. 196865–196877. https://doi.org/10.1109/access.2020.3034077
-
Maslyaev M., Hvatov A., Kalyuzhnaya A. Partial differential equations discovery with EPDE framework: Application for real and synthetic data. Journal of Computational Science, 2021, vol. 53, pp. 101345. https://doi.org/10.1016/j.jocs.2021.101345
-
Li K., Deb K., Zhang Q., Kwong S. An evolutionary many-objective optimization algorithm based on dominance and decomposition. IEEE Transactions on Evolutionary Computation, 2015, vol. 19, no. 5, pp. 694–716. https://doi.org/10.1109/TEVC.2014.2373386
-
Das I., Dennis J.E. Normal-boundary intersection: A new method for generating the Pareto surface in nonlinear multicriteria optimization problems. SIAM Journal on Optimization, 1998, vol. 8, no. 3, pp. 631–657. https://doi.org/10.1137/s1052623496307510