Menu
Publications
2025
2024
2023
2022
2021
2020
2019
2018
2017
2016
2015
2014
2013
2012
2011
2010
2009
2008
2007
2006
2005
2004
2003
2002
2001
Editor-in-Chief
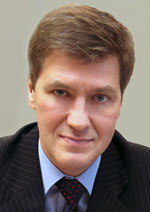
Nikiforov
Vladimir O.
D.Sc., Prof.
Partners
doi: 10.17586/2226-1494-2023-23-1-105-111
Probabilistic criteria for time-series predictability estimation
Read the full article

Article in English
For citation:
Abstract
For citation:
Kovantsev A.N. Probabilistic criteria for time-series predictability estimation. Scientific and Technical Journal of Information Technologies, Mechanics and Optics, 2023, vol. 23, no. 1, pp. 105–111. doi: 10.17586/2226-1494-2023-23-1-105-111
Abstract
Assessing the time series predictability is necessary for forecasting models validating, for classifying series to optimize the choice of the model and its parameters, and for analyzing the results. The difficulties in assessing predictability occur due to large heteroscedasticity of errors obtained when predicting several series of different nature and characteristics. In this work, the internal predictability of predictive modeling objects is investigated. Using the example of time series forecasting, we explore the possibility of quantifying internal predictability in terms of the probability (frequency) of obtaining a forecast with an error greater than some certain level. We also try to determine the relationship of such a measure with the characteristics of the time series themselves. The idea of the proposed method is to estimate the internal predictability by the probability of an error exceeding a predetermined threshold value. The studies were carried out on data from open sources containing more than seven thousand time series of stock market prices. We compare the probability of errors which exceed the allowable value (miss probabilities) for the same series on different forecasting models. We show that these probabilities differ insignificantly for different forecasting models with the same series, and hence, the probability can be a measure of predictability. We also show the relationship of the miss probability values with entropy, the Hurst exponent, and other characteristics of the series according to which the predictability can be estimated. It has been established that the resulting measure makes it possible to compare the predictability of time series with pronounced heteroscedasticity of forecast errors and when using different models. The measure is related to the characteristics of the time series and is interpretable. The results can be generalized to any objects of predictive modeling and forecasting quality scores. It can be useful to developers of predictive modeling algorithms, machine learning specialists in solving practical problems of forecasting.
Keywords: intrinsic predictability, forecasting error, misprediction
Acknowledgements. This research is supported by The Russian Science Foundation, Agreement No. 17-71-30029.
References
Acknowledgements. This research is supported by The Russian Science Foundation, Agreement No. 17-71-30029.
References
-
Lorenz E.N. Predictability - a problem partly solved. Predictability of Weather and Climate. Cambridge University Press, 2006, pp. 40–58. https://doi.org/10.1017/CBO9780511617652.004
-
Rummens S. The roots of the paradox of predictability: A reply to gijsbers. Erkenntnis, 2022. https://doi.org/10.1007/s10670-022-00617-8
-
Gijsbers V. The paradox of predictability. Erkenntnis, 2021. https://doi.org/10.1007/s10670-020-00369-3
-
Pennekamp F., Iles A.C., Garland J., Brennan G., Brose U., Gaedke U., Jacob U., Kratina P., Matthews B., Munch S., Novak M., Palamara G.M., Rall B.C., Rosenbaum B., Tabi A., Ward C., Williams R., Ye H., Petchey O.L. The intrinsic predictability of ecological time series and its potential to guide forecasting. Ecological Monographs, 2019, vol. 89, no. 2, pp. e01359. https://doi.org/10.1002/ecm.1359
-
Kovantsev A., Gladilin P. Analysis of multivariate time series predictability based on their features. Proc. of the IEEE International Conference on Data Mining Workshops (ICDMW), 2020, pp. 348–355. https://doi.org/10.1109/icdmw51313.2020.00055
-
Kovantsev A., Chunaev P., Bochenina K. Evaluating time series predictability via transition graph analysis. Proc. of the IEEE International Conference on Data Mining Workshops (ICDMW), 2021. https://doi.org/10.1109/ICDMW53433.2021.00135
-
Beckage B., Gross L.J., Kauffman S. The limits to prediction in ecological systems. Ecosphere, 2011, vol. 2, no. 11, pp. 1–12. https://doi.org/10.1890/ES11-00211.1
-
Naro D., Rummel C., Schindler K., Andrzejak R.G. Detecting determinism with improved sensitivity in time series: Rank-based nonlinear predictability score. Physical Review E, 2014, vol. 90, no. 3, pp. 032913. https://doi.org/10.1103/PhysRevE.90.032913
-
Mandelbrot B.B., Hudson R.L. The (Mis)behaviour of Markets: A Fractal View of Risk, Ruin and Reward. Basic Books, 2004.
-
Loskutov Y., Kotlyarov O.L., Istomin I.A., Zhuravlev D.I. Problems of nonlinear dynamics: III. Local methods of time series forecasting. Moscow University Physics Bulletin, 2002, vol. 57, no. 6.
-
Mitra S.K. Is Hurst exponent value useful in forecasting financial time series? Asian Social Science, 2012, vol. 8, no. 8, pp. 111–120. https://doi.org/10.5539/ass.v8n8p111
-
May R.M. Simple mathematical models with very complicated dynamics. The Theory of Chaotic Attractors, 2004, pp. 85–93. https://doi.org/10.1007/978-0-387-21830-4_7
-
Chuchueva I.A. The time series extrapolation model based on maximum likeness set. Information technologies. Information Technologies, 2010, no. 12, pp. 43–47. (in Russian)