Menu
Publications
2024
2023
2022
2021
2020
2019
2018
2017
2016
2015
2014
2013
2012
2011
2010
2009
2008
2007
2006
2005
2004
2003
2002
2001
Editor-in-Chief
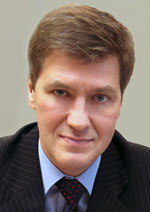
Nikiforov
Vladimir O.
D.Sc., Prof.
Partners
doi: 10.17586/2226-1494-2023-23-4-786-794
Optimization of human tracking systems in virtual reality based on a neural network approach
Read the full article

Article in Russian
For citation:
Abstract
For citation:
Obukhov A.D., Teselkin D.V. Optimization of human tracking systems in virtual reality based on a neural network approach. Scientific and Technical Journal of Information Technologies, Mechanics and Optics, 2023, vol. 23, no. 4, pp. 786–794 (in Russian). doi: 10.17586/2226-1494-2023-23-4-786-794
Abstract
The problem of determining the optimal number and location of tracking points on the human body to ensure the necessary accuracy of reconstruction of kinematic parameters of human movements in virtual space is considered. Optimization of the human tracking system in virtual reality has been performed to reduce the amount of transmitted information, computational load and cost of motion capture systems by reducing the number of physical sensors. The task of optimizing the number and location of tracking points on the human body necessary for the reconstruction of a virtual body model from a limited set of input points using numerical approximation of the regression function is set. An algorithm has been developed for collecting a large amount of data from a human body model in a virtual scene and from a motion capture suit in the real world. The smallest number of human body tracking points and their location were obtained using the proposed algorithm. Various neural network topologies have been trained and tested to approximate the regression relationship between a vector of tracking points limited in size (from 3 to 13) and a vector of 18 virtual points used for the complete reconstruction of the human body model. The necessary accuracy of reconstruction of kinematic parameters of human movements is provided at 5 and 7 input points. The proposed approach made it possible to use 5 or 7 physical sensors to build a model of the human body and restore the kinetic parameters of its movements in virtual reality. The approach can be applied to solving inverse kinematics problems in order to reduce the number of physical sensors placed on the surface of the object under study, to simplify the processing and transmission of information. By combining data from both the motion capture suit and the virtual avatar, the process of collecting information has been significantly accelerated, the volume of the training sample has been expanded and various patterns of user body movements have been modeled.
Keywords: virtual reality, human movement tracking, inverse kinematics, optimization of motion tracking and capture systems, digital representation of a person
Acknowledgements. The research was carried out at the expense of the grant of the Russian Science Foundation (no. 22-71-10057, https:// rscf.ru/project/22-71-10057/).
References
Acknowledgements. The research was carried out at the expense of the grant of the Russian Science Foundation (no. 22-71-10057, https:// rscf.ru/project/22-71-10057/).
References
- Obukhov A.D., Volkov A.A., Vekhteva N.A., Teselkin D.V., Arkhipov A.E. Human motion capture algorithm for creating digital shadows of the movement process. Journal of Physics: Conference Series. IOP Publishing, 2022, vol. 2388, no. 1, pp. 012033. https://doi.org/10.1088/1742-6596/2388/1/012033
- Azarby S., Rice A. Understanding the effects of virtual reality system usage on spatial perception: The potential impacts of immersive virtual reality on spatial design decisions. Sustainability, 2022, vol. 14, no. 16, pp. 10326. https://doi.org/10.3390/su141610326
- Parger M., Mueller J.H., Schmalstieg D., Steinberger M. Human upper-body inverse kinematics for increased embodiment in consumer-grade virtual reality. Proc. of the 24th ACM Symposium on Virtual Reality Software and Technology, 2018, pp. 1–10. https://doi.org/10.1145/3281505.3281529
- Caserman P., Garcia-Agundez A., Konrad R., Göbel S., Steinmetz R. Real-time body tracking in virtual reality using a Vive tracker. Virtual Reality, 2019, vol. 23, no. 2, pp. 155–168. https://doi.org/10.1007/s10055-018-0374-z
- Feigl T., Gruner L., Mutschler C., Roth D. Real-time gait reconstruction for virtual reality using a single sensor. Proc. of the 2020 IEEE International Symposium on Mixed and Augmented Reality Adjunct (ISMAR-Adjunct), 2020, pp. 84–89. https://doi.org/10.1109/ismar-adjunct51615.2020.00037
- Liu H., Zhang Z., Xie X., Zhu Y., Liu Y., Wang Y., Zhu S.-C. High-fidelity grasping in virtual reality using a glove-based system. Proc. of the 2019 International Conference on Robotics and Automation (ICRA), 2019, pp. 5180–5186. https://doi.org/10.1109/icra.2019.8794230
- Liu R., Liu C. Human motion prediction using adaptable recurrent neural networks and inverse kinematics. IEEE Control Systems Letters, 2021, vol. 5, no. 5, pp. 1651–1656. https://doi.org/10.1109/lcsys.2020.3042609
- Li J., Xu C., Chen Z., Bian S., Yang L., Lu C. Hybrik: A hybrid analytical-neural inverse kinematics solution for 3D human pose and shape estimation. Proc. of the IEEE/CVF Conference on Computer Vision and Pattern Recognition (CVPR), 2021, pp. 3383–3392. https://doi.org/10.1109/cvpr46437.2021.00339
- Oyama E., Agah A., MacDorman K.F., Maeda T., Tachi S. A modular neural network architecture for inverse kinematics model learning. Neurocomputing, 2001, vol. 38-40, pp. 797–805. https://doi.org/10.1016/s0925-2312(01)00416-7
- Bai Y., Luo M., Pang F. An algorithm for solving robot inverse kinematics based on FOA optimized BP neural network. Applied Sciences, 2021, vol. 11, no. 15, pp. 7129. https://doi.org/10.3390/app11157129
- Kratzer P., Toussaint M., Mainprice J. Prediction of human full-body movements with motion optimization and recurrent neural networks. Proc. of the 2020 IEEE International Conference on Robotics and Automation (ICRA), 2020, pp. 1792–1798. https://doi.org/10.1109/icra40945.2020.9197290
- Bataineh M., Timothy M., Karim A.-M., Jasbir A. Neural network for dynamic human motion prediction. Expert Systems with Applications, 2016, vol. 48, pp. 26–34. https://doi.org/10.1016/j.eswa.2015.11.020
- Kucherenko T., Beskow J., Kjellström H. A neural network approach to missing marker reconstruction in human motion capture. arXiv, 2018, arXiv:1803.02665. https://doi.org/10.48550/arXiv.1803.02665
- Geigel J., Schweppe M. Motion capture for realtime control of virtual actors in live, distributed, theatrical performances. Proc. of the 2011 IEEE International Conference on Automatic Face & Gesture Recognition (FG), 2011, pp. 774–779. https://doi.org/10.1109/FG.2011.5771347
- Degen R., Tauber A., Nüßgen A., Irmer M., Klein F., Schyr C., Leijon M., Ruschitzka M. Methodical approach to integrate human movement diversity in real-time into a virtual test field for highly automated vehicle systems. Journal of Transportation Technologies, 2022, vol. 12, no. 3, pp. 296–309. https://doi.org/10.4236/jtts.2022.123018
- Sers R., Forrester S., Moss E., Ward S., Ma J., Zecca M. Validity of the Perception Neuron inertial motion capture system for upper body motion analysis. Measurement, 2020, vol. 149, pp. 107024. https://doi.org/10.1016/j.measurement.2019.107024