Menu
Publications
2024
2023
2022
2021
2020
2019
2018
2017
2016
2015
2014
2013
2012
2011
2010
2009
2008
2007
2006
2005
2004
2003
2002
2001
Editor-in-Chief
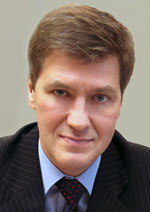
Nikiforov
Vladimir O.
D.Sc., Prof.
Partners
doi: 10.17586/2226-1494-2024-24-1-51-61
An improved performance of RetinaNet model for hand-gun detection in custom dataset and real time surveillance video
Read the full article

Article in English
For citation:
Abstract
For citation:
Khin P.P., Htaik N.M. An improved performance of RetinaNet model for hand-gun detection in custom dataset and real time surveillance video. Scientific and Technical Journal of Information Technologies, Mechanics and Optics, 2024, vol. 24, no. 1, pp. 51–61. doi: 10.17586/2226-1494-2024-24-1-51-61
Abstract
The prevalence of armed robberies has become a significant concern in today’s world, necessitating the development of effective detection systems. While various detection devices exist in the market, they do not possess the capability to automatically detect and alarm the presence of guns during robbery activities. In order to address this issue, a deep learning-based approach using gun detection using RetinaNet model is proposed. The objective is to accurately detect guns and subsequently alert either the police station or the bank owner. RetinaNet, the core of the system, comprises three main components: the Residual Neural Network (ResNet), the Feature Pyramid Network (FPN), and the Fully Convolutional Networks (FCN). These components work together to enable real-time detection of guns without the need for human intervention. Proposed implementation uses a custom robbery detection dataset that consists of gun, no-gun and robbery activity classes. By evaluating the performance of the proposed model on our custom dataset, it is evident that the ResNet50 backbone architecture yields outperforms for the accuracy in robbery detection that reached in 0.92 of Mean Average Precision (mAP). The model effectiveness lies in its ability to accurately identify the presence of guns during robbery activities.
Keywords: robbery activities, RetinaNet, ResNet50, FPN, FCN
References
References
- Dever J., da Vitoria Lobo N., Shah M. Automatic visual recognition of armed robbery. Proc. of the 16th International Conference on Pattern Recognition. V. 1, 2002, pp. 451–455. https://doi.org/10.1109/ICPR.2002.1044755
- Ahmed S., Bhatti M.T., Khan M.G., Lövström B., Shahid M. Development and optimization of deep learning models for weapon detection in surveillance videos. Applied Sciences, 2022, vol. 12, no. 12, pp. 5772. https://doi.org/10.3390/app12125772
- Kakadiya R., Lemos R., Mangalan S., Pillai M. AI based automatic robbery/theft detection using smart surveillance in banks. Proc. of the 3rd International Conference on Electronics, Communication and Aerospace Technology (ICECA), 2019, pp. 201–204. https://doi.org/10.1109/iceca.2019.8822186
- Narejo S., Pandey B., Vargas D.E., Rodriguez C., Anjum M.R. Weapon detection using YOLO V3 for smart surveillance system. Mathematical Problems in Engineering, 2021, vol. 2021, pp. 9975700. https://doi.org/10.1155/2021/9975700
- Salido J., Lomas V., Ruiz-Santaquiteria J., Deniz O. Automatic handgun detection with deep learning in video surveillance images. Applied Sciences, 2021, vol. 11, no. 13, pp. 6085. https://doi.org/10.3390/app11136085
- Zahrawi M., Shaalan K. Improving video surveillance systems in banks using deep learning techniques. Scientific Reports, 2023, vol. 13, pp. 7911. https://doi.org/10.1038/s41598-023-35190-9
- Ineneji C., Kusaf M. Hybrid weapon detection algorithm, using material test and fuzzy logic system. Computers & Electrical Engineering, 2019, vol. 78, pp. 437–448. https://doi.org/10.1016/j.compeleceng.2019.08.005
- Warsi A., Abdullah M., Husen M.N., Yahya M., Jawaid N. Gun detection system using YOLOv3. Proc. of the IEEE International Conference on Smart Instrumentation, Measurement and Application (ICSIMA), 2019, pp. 1–4. https://doi.org/10.1109/ICSIMA47653.2019.9057329
- Bhatti M.T., Khan M.G., Aslam M., Fiaz M.J. Weapon detection in real-time CCTV videos using deep learning. IEEE Access, 2021, vol. 9, pp. 34366–4382. https://doi.org/10.1109/ACCESS.2021.3059170
- Hashmi T.S.S., Haq N.U., Fraz M.M., Shahzad M. Application of deep learning for weapons detection in surveillance videos. Proc. of the 2021 International Conference on Digital Futures and Transformative Technologies (ICoDT2), 2021. https://doi.org/10.1109/ICoDT252288.2021.9441523
- Alaqil R.M., Alsuhaibani J.A., Alhumaidi B.A., Alnasser R.A., Alotaibi R.D., Benhidour H. Automatic gun detection from images using Faster R-CNN. Proc. of the IEEE International Conference of Smart Systems and Emerging Technologies (SMARTTECH), 2020, pp. 149–154. https://doi.org/10.1109/SMART-TECH49988.2020.00045
- Yang M., Xiao X., Liu Z., Sun L., Guo W., Cui L., Sun D., Zhang P., Yang G. Deep RetinaNet for dynamic left ventricle detection in multiview echocardiography classification. Scientific Programming, 2020, vol. 2020, pp. 7025403. https://doi.org/10.1155/2020/7025403
- Bhabad D., Kadam S., Malode T., Shinde G., Bage D. Object detection for night vision using deep learning algorithms. International Journal of Computer Trends and Technology, 2023, vol. 71, no. 2, pp. 87–92. https://doi.org/10.14445/22312803/ijctt-v71i2p113
- Rani E.E., Baulkani S. Construction of deep learning model using ResNet 50 for schizophrenia prediction from rsFMRI images. Research Square, 2022. https://doi.org/10.21203/rs.3.rs-2106170/v1
- Guan Q., Wang Y., Ping B., Li D., Du J., Qin Y., Lu H., Wan X., Xiang J. Deep convolutional neural network VGG-16 model for differential diagnosing of papillary thyroid carcinomas in cytological images: A pilot study. Journal of Cancer, 2019, vol. 10, no. 20, pp. 4876–4882. https://doi.org/10.7150/jca.28769