Menu
Publications
2025
2024
2023
2022
2021
2020
2019
2018
2017
2016
2015
2014
2013
2012
2011
2010
2009
2008
2007
2006
2005
2004
2003
2002
2001
Editor-in-Chief
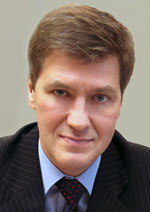
Nikiforov
Vladimir O.
D.Sc., Prof.
Partners
doi: 10.17586/2226-1494-2024-24-3-424-430
Improving the algorithm for processing data from multisensor system in tasks of determining quality parameters in vegetable oils
Read the full article

Article in English
For citation:
Abstract
For citation:
Semenov V.V. Improving the algorithm for processing data from multisensor system in tasks of determining quality parameters in vegetable oils. Scientific and Technical Journal of Information Technologies, Mechanics and Optics, 2024, vol. 24, no. 3, pp. 424–430. doi: 10.17586/2226-1494-2024-24-3-424-430
Abstract
The effective functioning of modern production systems is impossible without using of methods for processing and analyzing data continuously generated during operation. Limitations imposed on the speed and precision of determining the required indicators lead to the need of optimizing the algorithms used. Multisensor systems, as a rule, have an excessive number of cross-sensitive sensors, and their signals can be used to determine various indicators of a similar physical nature. The purpose of the study is to improve the algorithm for processing multidimensional data from multisensor systems. Principal component analysis was applied as part of the developed algorithm for the formation of informative features. Partial least squares regression was used to build regression models. The data set for approbation of proposed approach was obtained through potentiometric measurements using a digital mV-meter. An experiment is described using a multisensor system called “electronic tongue”, consisting of 12 cross-sensitive potentiometric sensors. In the experiment, real samples of vegetable oils acted as analyzed objects. Regression models were built to determine three quality indicators of vegetable oils: peroxide value, para-anisidine value and total tocopherol concentrations. The results of the study were compared with known scientific works. A comparative analysis allowed us to conclude that using of the most informative sources selected according to the proposed algorithm can significantly reduce the root mean square error of prediction. The results obtained can be used both in systems for identifying deviations in production processes in “Industry 4.0” enterprises, and for expressly identifying counterfeit products.
Keywords: quantitative analysis, quality control, vegetable oils, potentiometric sensors, multisensor system, time series, principal component analysis
References
References
- Caruana L., Francalanza E. A Safety 4.0 approach for collaborative robotics in the factories of the future. Procedia Computer Science, 2023, vol. 217, pp. 17847–1793. https://doi.org/10.1016/j.procs.2022.12.378
- Zegzhda D.P., Vasilev U.S., Poltavtseva M.A., Kefele I.F., Borovkov A.I. Advanced production technologies security in the era of digital transformation. Voprosy kiberbezopasnosti, 2018, no. 2(26), pp. 2–15. (in Russian). https://doi.org/10.21681/2311-3456-2018-2-2-15
- Alulema D., Criado J., Iribarne L., Fernández-García A.J., Ayala R. SI4IoT: A methodology based on models and services for the integration of IoT systems. Future Generation Computer Systems, 2023, vol. 143, pp. 132–151. https://doi.org/10.1016/j.future.2023.01.023
- Kivrak H., Karakusak M.Z., Watson S., Lennox B. Cyber–physical system architecture of autonomous robot ecosystem for industrial asset monitoring. Computer Communications, 2024, vol. 218, pp. 72–84. https://doi.org/10.1016/j.comcom.2024.02.013
- Semenov V.V. Method for monitoring the state of elements of cyber-physical systems based on time series analysis. Scientific and Technical Journal of Information Technologies, Mechanics and Optics, 2022, vol. 22. no. 6, pp. 1150–1158. (in Russian). https://doi.org/10.17586/2226-1494-2022-22-6-1150-1158
- Wold S., Esbensen K., Geladi P. Principal component analysis. Chemometrics and Intelligent Laboratory Systems, 1987, vol. 2, no. 1–3, pp. 37–52. https://doi.org/10.1016/0169-7439(87)80084-9
- Yuan S., Yang M., Reniers G. Integrated process safety and process security risk assessment of industrial cyber-physical systems in chemical plants. Computers in Industry, 2024, vol. 155, pp. 104056. https://doi.org/10.1016/j.compind.2023.104056
- Tonin F., Tao Q., Patrinos P., Suykens J. Deep Kernel Principal Component Analysis for multi-level feature learning. Neural Networks, 2024, vol. 170, pp. 578–595. https://doi.org/10.1016/j.neunet.2023.11.045
- Yamaguchi T., Yamashita Y. Multi-target regression via target combinations using principal component analysis. Computers & Chemical Engineering, 2024, vol. 181, pp. 108510. https://doi.org/10.1016/j.compchemeng.2023.108510
- Cangelosi R., Goriely A. Component retention in principal component analysis with application to cDNA microarray data. Biology Direct, 2007, vol. 2, pp. 2. https://doi.org/10.1186/1745-6150-2-2
- Vlasov Yu.G., Bychkov E.A., Legin A.V. Chalcogenide glass chemical sensors: Research and analytical applications. Talanta, 1994, vol. 41, no. 6, pp. 1059–1063. https://doi.org/10.1016/0039-9140(94)00124-3
- Semenov V., Volkov S., Khaydukova M., Fedorov A., Lisitsyna I., Kirsanov D., Legin A. Determination of three quality parameters in vegetable oils using potentiometric e-tongue. Journal of Food Composition and Analysis, 2019, vol. 75, pp. 75–80. https://doi.org/10.1016/j.jfca.2018.09.015
- Gromadzka J., Wardencki W. Trends in edible vegetable oils analysis. Part A. Determination of different components of edible oils - a review. Polish Journal of Food and Nutrition Sciences, 2011, vol. 61, no. 1, pp. 33–43. https://doi.org/10.2478/v10222-011-0002-z
- Martinez-Velasco J.D., Filomena-Ambrosio A., Garzon-Castro C.L. Technological tools for the measurement of sensory characteristics in food: A review. F1000Research, 2024, no. 12, pp. 340. https://doi.org/10.12688/f1000research.131914.2
- Geladi P., Kowalski B.R. Partial least-squares regression: a tutorial. Analytica Chimica Acta, 1986, vol. 185, pp. 1–17. https://doi.org/10.1016/0003-2670(86)80028-9
- Pattern Recognition and Machine Learning. Ed. by C.M. Bishop. Springer-Verlag New York, 2006, XX, 778 p. https://doi.org/10.1007/978-0-387-45528-0