Menu
Publications
2025
2024
2023
2022
2021
2020
2019
2018
2017
2016
2015
2014
2013
2012
2011
2010
2009
2008
2007
2006
2005
2004
2003
2002
2001
Editor-in-Chief
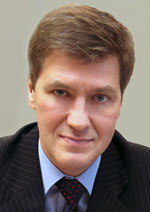
Nikiforov
Vladimir O.
D.Sc., Prof.
Partners
doi: 10.17586/2226-1494-2024-24-5-726-737
Algorithm for navigation on the terrain of unmanned aerial vehicles with machine vision
Read the full article

Article in Russian
For citation:
Abstract
For citation:
Zikratov I.A., Belyaev P.U., Neverov E.A. Algorithm for navigation on the terrain of unmanned aerial vehicles with machine vision. Scientific and Technical Journal of Information Technologies, Mechanics and Optics, 2024, vol. 24, no. 5, pp. 726–737 (in Russian). doi: 10.17586/2226-1494-2024-24-5-726-737
Abstract
One of the problems solved by developers of autonomously controlled unmanned aerial vehicles is the task of determining by the drone its exact position over the terrain without the help of global satellite navigation systems. The existing mass-dimensional and energy limitations for small-sized drones lead to the necessity of using relatively simple algorithms in drone computing devices. The paper considers methods of navigation of unmanned aerial vehicles using computer vision implemented by on-board optical and computing devices. Machine vision implemented by on-board computing devices provides autonomy of small-sized aircraft in the absence or unstable communication channel with the control center and/or satellite navigation system. The proposed algorithm solves the problem of identifying an area of terrain observed from a drone with a terrain image stored in the memory of the drone control system. The drone location is determined by the minimum (maximum) value of the discrepancy between the observed current image and the image of the terrain area stored in the drone memory device. The solution of the identification problem is based on the concept of immunocomputing using singular value decomposition of the feature matrix of the identified objects. This approach allows providing high quality indicators of identification due to decomposition of the feature matrix into three simple transformations for transition to a new feature space which is not identifiable, but whose components are statistically significant. The quality indicators of the developed algorithm were evaluated in comparison with the known method of image identification by calculating the correlation function between two arrays of features. A series of tests were carried out in which the probability of correct location determination and the speed of the algorithms were evaluated for the same initial data. It is shown that when pre-preparing a “reference” image stored in the drone memory device, the speed of the developed method exceeds the speed of the method based on the calculation of the correlation function of the compared images by an order of magnitude. The mean absolute error of correct positioning using the proposed method ranges from 0.109 to 0.153. The proposed algorithm can be used by developers of navigation systems for small-sized unmanned aerial vehicles due to its low resource requirements while maintaining a level of accuracy sufficient in the context of solving problems of orientation on the terrain. Devices realizing the proposed orientation algorithm have better energy and mass-size characteristics.
Keywords: image identification, singular matrix decomposition, immunocomputing, drone navigation
References
References
- Kabaldin Iu.G., Kiselev A.V., Shatagin D.A. The concept of developing a route for an unmanned vehicle in the Arctic conditions. Eurasian Union of Scientists, 2016, no. 31-1, pp. 63–66. (in Russian)
- Wells D., Beck N. Guide to GPS positioning. Canadian GPS Assoc, 1987, 600 p.
- Bochkarev A.M. Correlation extreme navigation systems. Zarubezhnaja radiojelektronika, 1981, no. 9, pp. 28–53. (in Russian)
- Beloglazov I.N., Tarasenko V.P. Correlation Extreme Systems. Moscow, Sovetskoe Radio Publ., 1974, 392 p. (in Russian)
- Tarasenko V.P., Timofeev A.V. Confidence estimation of registration precision in correlation-extreme systems. Avtometriya, 1990, no. 4, pp. 106–111. (in Russian)
- Kazmin V.N., Noskov V.P. Detecting geometric and semantic objects in range image for robot navigation and environment reconstruction. Izvestiya SFedU. Engineering Sciences, 2015, no. 10(171), pp. 71–83. (in Russian)
- Marinho L.B., Rebouças Filho P.P., Almeida J.S., Souza J.W.M., Souza Jr A.H., de Albuquerque V.H.C. A novel mobile robot localization approach based on classification with rejection option using computer vision. Computers & Electrical Engineering, 2018, vol. 68, pp. 26–43. https://doi.org/10.1016/j.compeleceng.2018.03.047
- Murray D., Little J.J. Using real-time stereo vision for mobile robot navigation. Autonomous Robots, 2000, vol. 8, no. 2, pp. 161–171. https://doi.org/10.1023/A:1008987612352
- Lulio L.C., Tronco M.L., Porto A.J.V. JSEG-based image segmentation in computer vision for agricultural mobile robot navigation. Proc. of the IEEE International Symposium on Computational Intelligence in Robotics and Automation - (CIRA), 2009, pp. 240–245. https://doi.org/10.1109/cira.2009.5423201
- Hussein A., Marin-Plaza P., Martin D., de la Escalera A., Armingol J.M. Autonomous off-road navigation using stereo-vision and laser-rangefinder fusion for outdoor obstacles detection. Proc. of the IEEE Intelligent Vehicles Symposium (IV), 2016, pp. 104–109. https://doi.org/10.1109/ivs.2016.7535372
- Sozzi M., Kayad A., Marinello F., Taylor J., Tisseyre B. Comparing vineyard imagery acquired from Sentinel-2 and Unmanned Aerial Vehicle (UAV) platform. OENO One, 2020, vol. 54, no. 2, pp. 189–197. https://doi.org/10.20870/oeno-one.2020.54.1.2557
- Pastonchi L., Di Gennaro S.F., Toscano P., Matese A. Comparison between satellite and ground data with UAV-based information to analyse vineyard spatio-temporal variability: This article is pub-lished in cooperation with the XIIIth International Terroir Congress November 17-18, 2020, Adelaide, Australia. Guest editors: Cassandra Collins and Roberta De Bei. OENO One, 2020, vol. 54, no. 4, pp. 919–934. https://doi.org/10.20870/oeno-one.2020.54.4.4028
- Lu Z., Liu F., Lin X. Vision-based localization methods under GPS-denied conditions. arXiv, 2022, arXiv:2211.11988. https://doi.org/10.48550/arXiv.2211.11988
- Lu K., Xu R., Li J., Lv Y., Lin H., Liu Y. A vision-based detection and spatial localization scheme for forest fire inspection from UAV. Forests, 2022, vol. 13, no. 3, pp. 383. https://doi.org/10.3390/f13030383
- Tarakanov A., Skormin V., Sokolova S. Immunocomputing: Principles and Applications. New York, NY, Springer, 2003, 193 p.
- Tarakanov A., Nicosia G. Foundations of immunocomputing. Proc. of the IEEE Symposium on Foundations of Computational Intelligence, 2007, pp. 503–508. https://doi.org/10.1109/foci.2007.371519
- Tarakanov A.O. Mathematical models of intrusion detection by an intelligent immunochip. Communications in Computer and Information Science, 2013, vol. 1, pp. 308–319. https://doi.org/10.1007/978-3-540-73986-9_26
- Solomatin A.Y., Zikratov I.A. Method of person identification by the facial image in video surveillance system using scientific methods of immunocomputing. Information and Space, 2015, no. 2, pp. 47–51. (in Russian)
- Solomatin A.Yu., Lyubert A.S., Zikratov I.A. Moving person identification in video surveillance systems. Scientific and Technical Journal of Information Technologies, Mechanics and Optics, 2014, no. 4(92), pp. 124–131. (in Russian)
- Gubin A., Litvinov V., Filippov F. Use of a principal component analysis for the recognition of the graphic objects. Proceedings of Telecommunication Universities, 2016, vol. 2, no. 3, pp. 27–31. (in Russian)
- Litvinov V.L., Kozlova L.P., Filippov F.V. The use of a matrix decompositions for dimension reduction of training sample. Proc. of the IEEE II International Conference on Control in Technical Systems (CTS), 2017, pp. 282–284. https://doi.org/10.1109/CTSYS.2017.8109546
- Alieva O., Gangan E., Ilyushin E., Kachalin A.Automatic evaluation of recommendation models. Modern information technologies and IT-education, 2020, vol. 16, no. 2, pp. 398–406. (in Russian). https://doi.org/10.25559/SITITO.16.202002.398-406
- Minin A.S. Application of singular value decomposition to reduce dimensionality in data analysis. Tendencii razvitija nauki i obrazovanija, 2023, no. 99-7, pp. 55–58. (in Russian). https://doi.org/10.18411/trnio-07-2023-377
- Studio encoding parameters of digital television for standard 4: 3 and wide-screen 16: 9 aspect ratios. Recommendation ITU-R BT.601-7 (03/2011).
- Timofeev A.V. Detection of a small target object in blurry images affected by affine distortions. Scientific and Technical Journal of Information Technologies, Mechanics and Optics, 2021, vol. 21, no. 2, pp. 206–224. (in Russian). https://doi.org/10.17586/2226-1494-2021-21-2-206-224