Menu
Publications
2024
2023
2022
2021
2020
2019
2018
2017
2016
2015
2014
2013
2012
2011
2010
2009
2008
2007
2006
2005
2004
2003
2002
2001
Editor-in-Chief
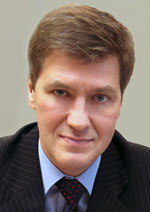
Nikiforov
Vladimir O.
D.Sc., Prof.
Partners
Summaries of the Issue
REVIEW PAPERS
669
A review of modern methods and technologies for automatic machine translation for the deaf and hard of hearing is presented, including recognition and synthesis of both spoken and sign languages. These methods aim to facilitate effective communication between deaf/hard-of-hearing and hearing individuals. The proposed solutions have potential applications in contemporary human-machine interaction interfaces. Key aspects of new technologies are examined, including methods for sign language recognition and synthesis, audiovisual speech recognition and synthesis, existing corpora for training neural network models, and current systems for automatic machine translation. Current neural network approaches are presented, including the use of deep learning methods such as convolutional and recurrent neural networks as well as transformers. An analysis of existing corpora for training recognition and synthesis systems is provided, along with an evaluation of the challenges and limitations of existing machine translation systems. The main shortcomings and specific problems of current automatic machine translation technologies are identified, and promising solutions are proposed. Special attention is given to the applicability of automatic machine translation systems in real-world scenarios. The need for further research in data collection and annotation, development of new methods and neural network models, and creation of innovative technologies for processing audio and video data to enhance the quality and efficiency of the existing automatic machine translation systems is highlighted.
687
This paper examines routing algorithms for networks on a chip (NoC). An analysis of existing routing algorithms is provided; their limitations and areas of application are highlighted. The algorithms were evaluated taking into account the requirements of specific applications and architecture features. The results of comparing the performance of the considered algorithms are presented. Analysis and comparison of various routing algorithms for NoC are carried out taking into account critical characteristics. The main attention is paid to such routing algorithms as the deterministic XY algorithm, the model rotation algorithm, congestion-aware routing, fault-tolerant routing, Quality of Service routing, and the ant colony algorithm. It is shown that the choice of routing algorithm should be based on the specific requirements and conditions of use of the network. The importance of adapting to a variety of conditions and tasks that NoC users and developers may encounter is shown. Based on data from existing studies, an analysis of algorithms was carried out based on several key indicators: latency, throughput, adaptability, fault tolerance and implementation complexity. The strengths and weaknesses of each algorithm are identified in various use scenarios and under different network loads. It is shown that the choice of a routing algorithm should be based on the specific requirements and conditions of use of the network, as well as on the balance between performance, adaptability, fault tolerance and implementation complexity. The study contributes to the understanding of the effectiveness of various routing algorithms in NoC, providing recommendations for their selection depending on the specific application requirements and system architecture. The study contributes to a better understanding of the impact of routing algorithms on the overall performance of NoC, suggesting directions for further improvements in this area. The results of the work can be applied in the design and development of highperformance multiprocessor systems on a chip where efficient data routing between various systems components is a key factor in ensuring high performance. The importance of developing fault-tolerant routing algorithms that can ensure the continuity of system operation in the event of failures of individual components or units is emphasized. This is especially important for mission-critical applications where service continuity and reducing the risk of data loss are top priorities.
OPTICAL ENGINEERING
699
The paper presents the results of an experimental study of the possibility of using a narrow-band semiconductor distributed feedback laser diode used as a source and detector of optical radiation to detect the spectral response from a fiber Bragg grating. The DFB laser “LDI-1550-DFB-2.5G-20/70” from the company “Laserscom”, mass-produced on the Russian market and having standard characteristics, was chosen as the laser diode under study. To sweep the central wavelength of a semiconductor distributed feedback laser diode in the range 1549.5–1552 nm, direct pulse current modulation was used with a frequency of 100 kHz, a duty cycle of 40, and a current value of 1 A per pulse. The radiation reflected from the fiber Bragg grating corresponding to the central Bragg wavelength was recorded as a change in voltage at the anode and cathode of the laser diode due to the photoelectric effect in the laser diode. An experimental assessment of the optoelectronic parameters of a laser diode in photovoltaic and short-circuit modes was carried out: dark current, bandwidth and spectral sensitivity. The evaluation was carried out at a temperature of 25 °C. A measuring circuit has been created to detect the response from a fiber Bragg grating based on direct pulse current modulation and the photovoltaic mode of a semiconductor distributed feedback laser diode. It is shown that the photovoltaic mode of the laser diode is applicable to problems of recording optical radiation. The amplitude-frequency characteristic of a laser diode in the photovoltaic mode was experimentally obtained depending on the forward bias voltage. It is experimentally found that the –3 dB bandwidth is 300 MHz and the maximum sensitivity is 0.1 A/W in short-circuit mode, and the amplitude response is linear in the wavelength range from 1540 to 1560 nm. For the laser diode under study, the reverse branch of the current-voltage characteristic was experimentally obtained and the dark current at zero bias of the laser diode is 12.5 pA. The demonstrated method of FBG interrogation can be used for miniaturization and simplification of optical devices for fiber Bragg grating interrogation. The obtained results may be useful to specialists in fiber optic sensors, system for interrogation and processing signals from fiber optic sensors.
709
717
726
Nikulin I.N., Rofer Yu.I.
Development of a fiber-optic system for monitoring geotechnical structures
Development of a fiber-optic system for monitoring geotechnical structures
738
AUTOMATIC CONTROL AND ROBOTICS
MATERIAL SCIENCE AND NANOTECHNOLOGIES
COMPUTER SCIENCE
758
770
779
788
797
806
815
824
834
843
849