Menu
Publications
2025
2024
2023
2022
2021
2020
2019
2018
2017
2016
2015
2014
2013
2012
2011
2010
2009
2008
2007
2006
2005
2004
2003
2002
2001
Editor-in-Chief
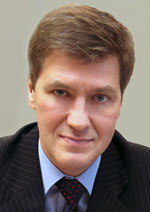
Nikiforov
Vladimir O.
D.Sc., Prof.
Partners
doi: 10.17586/2226-1494-2024-24-5-788-796
Enhanced anomaly detection in network security: a comprehensive ensemble approach
Read the full article

Article in English
For citation:
Abstract
For citation:
Pandey R., Pandey M., Nazarov A.N. Enhanced anomaly detection in network security: a comprehensive ensemble approach. Scientific and Technical Journal of Information Technologies, Mechanics and Optics, 2024, vol. 24, no. 5, pp. 788–796. doi: 10.17586/2226-1494-2024-24-5-788-796
Abstract
Detection and handling of anomalous behavior in the network systems are peremptory efforts to ensure security for vulnerable infrastructures amidst the dynamic context of cybersecurity. In this paper, we propose an ensemble machine learning model architecture that leverages the strengths of XGBoost, Gradient Boosting, Random Forest, and Support Vector Machine models to identify anomalies in the dataset. This method utilizes an ensemble of these models with weighted voting based on accuracy to enhance anomaly detection for robust and adaptive real-world network security. The proposed ensemble learning model is evaluated on standard metrics and demonstrates exceptional efficacy, achieving an impressive accuracy of 99.68 % on NSL KDD dataset. This remarkable performance extends the model prowess in discerning anomalies within network traffic showcasing its potential as a robust tool for enhancing cybersecurity measures against evolving threats.
Keywords: anomaly detection, bagging and boosting, ensemble approach, network security, neural network
References
References
- Reichenbach M. New challenges in electronic payments. Intelligent Enterprises of the 21st Century. Ed. by J.N.D. Gupta, S. Sharma. IGI Global, 2004, pp. 153–162. https://doi.org/10.4018/9781591401605.ch010
- Kebande V.R., Karie N.M., Ikuesan R.A. Real-time monitoring as a supplementary security component of vigilantism in modern network environments. International Journal of Information Technology, 2021, vol. 13, no. 1, pp. 5–17. https://doi.org/10.1007/s41870-020-00585-8
- Hareesh R., Senthil Kumar R.K., Kalluri R., Bindhumadhava B.S. Critical infrastructure asset discovery and monitoring for cyber security. Lecture Notes in Electrical Engineering, 2022, vol. 847, pp. 289–300. https://doi.org/10.1007/978-981-16-9008-2_27
- Savage D., Zhang X., Yu X., Chou P., Wang Q. Anomaly detection in online social networks. Social Networks, 2014, vol. 39, pp. 62–70. https://doi.org/10.1016/j.socnet.2014.05.002
- Benaddi H., Ibrahimi K., Benslimane A. Improving the intrusion detection system for NSL-KDD dataset based on PCA-fuzzy clustering-KNN. Proc. of the 6th International Conference on Wireless Networks and Mobile Communications (WINCOM), 2018, pp. 1–6. https://doi.org/10.1109/wincom.2018.8629718
- Su T., Sun H., Zhu J., Wang S., Li Y. BAT: Deep learning methods on network intrusion detection using NSL-KDD dataset. IEEE Access, 2020, vol. 8, pp. 29575–29585. https://doi.org/10.1109/access.2020.2972627
- Wang C., Zhou H., Hao Z., Hu S., Li J., Zhang X., Jiang B., Chen X. Network traffic analysis over clustering-based collective anomaly detection. Computer Networks, 2022, vol. 205, pp. 108760. https://doi.org/10.1016/j.comnet.2022.108760
- Keim Y., Mohapatra A.K. Cyber threat intelligence framework using advanced malware forensics. International Journal of Information Technology, 2022, vol. 14, no. 1, pp. 521–530. https://doi.org/10.1007/s41870-019-00280-3
- XuB., Jang-Jaccard J., Singh A., Wei Y., Sabrina F. Improving performance of autoencoder-based network anomaly detection on nsl-kdd dataset. IEEE Access,2021, vol. 9, pp. 140136–140146. https://doi.org/10.1109/access.2021.3116612
- Shone N., Ngoc T.N., Phai V.D., Shi Q. A deep learning approach to network intrusion detection. IEEE Transactions on Emerging Topics in Computational Intelligence, 2018, vol. 2, no. 1, pp. 41–50. https://doi.org/10.1109/tetci.2017.2772792
- Sharma B., Sharma L., Lal C. Anomaly based network intrusion detection for IoT attacks using convolution neural network. Proc. of the IEEE 7th International Conference for Convergence in Technology (I2CT), 2022, pp. 1–6. https://doi.org/10.1109/i2ct54291.2022.9824229
- KrzemieńW., Jędrasiak K., Nawrat A. Anomaly detection in software defined networks using ensemble learning. Lecture Notes in Networks and Systems,2022, vol. 439, pp. 629–643.https://doi.org/10.1007/978-3-030-98015-3_44
- Staudemeyer R.C. Applying long short-term memory recurrent neural networks to intrusion detection. South African Computer Journal, 2015, vol. 56, pp. 136–154. https://doi.org/10.18489/sacj.v56i1.248
- Kim J., Kim J., Thu H.L.T., Kim H. Long short term memory recurrent neural network classifier for intrusion detection. Proc. of the International Conference on Platform Technology and Service (PlatCon), 2016, pp. 1–5. https://doi.org/10.1109/platcon.2016.7456805
- Liu Z., Thapa N., Shaver A., Roy K., Yuan X., Khorsandroo S. Anomaly detection on IoT network intrusion using machine learning. Proc. of the International Conference on Artificial Intelligence, Big Data, Computing and Data Communication Systems (ICABCD), 2020, pp. 1–5. https://doi.org/10.1109/icabcd49160.2020.9183842
- Khan W., Haroon M. An unsupervised deep learning ensemble model for anomaly detection in static attributed social networks. International Journal of Cognitive Computing in Engineering,2022, vol. 3, pp. 153–160. https://doi.org/10.1016/j.ijcce.2022.08.002
- Gupta K., Sharma D.K., Gupta K.D., Kumar A. A tree classifier based network intrusion detection model for Internet of Medical Things. Computers and Electrical Engineering,2022, vol. 102, pp. 108158. https://doi.org/10.1016/j.compeleceng.2022.108158
- Ma Q., Sun C., Cui B. A novel model for anomaly detection in network traffic based on support vector machine and clustering. Security and Communication Networks, 2021, pp. 170788. https://doi.org/10.1155/2021/2170788
- Iliyasu A.S., Deng H. N-GAN: a novel anomaly-based network intrusion detection with generative adversarial networks. International Journal of Information Technology, 2022, vol. 14, no. 7, pp. 3365–3375. https://doi.org/10.1007/s41870-022-00910-3
- Tavallaee M., Bagheri E., Lu W., Ghorbani A.A. A detailed analysis of the KDD CUP 99 data set. Proc. of the IEEE Symposium on Computational Intelligence for Security and Defense Applications, 2009, pp. 1–6. https://doi.org/10.1109/cisda.2009.5356528
- Panesar A. Evaluating machine learning models. Machine Learning and AI for Healthcare. Apress, Berkeley, CA, 2021, pp. 189–205. https://doi.org/10.1007/978-1-4842-6537-6_7
- Assy A.T., Mostafa Y., Abd El-khaleq A., Mashaly M. Anomaly-based intrusion detection system using one-dimensional convolutional neural network. Procedia Computer Science, 2023, vol. 220, pp. 78–85. https://doi.org/10.1016/j.procs.2023.03.013
- Acharya T., Annamalai A., Chouikha M.F. Efficacy of bidirectional LSTM model for network-based anomaly detection. Proc. of the IEEE 13th Symposium on Computer Applications & Industrial Electronics (ISCAIE), 2023, pp. 336–341. https://doi.org/10.1109/iscaie57739.2023.10165336
- Kavitha S., Uma Maheswari N., Venkatesh R. Network anomaly detection for NSL-KDD dataset using deep learning. Information Technology in Industry, 2021, vol. 9, no. 2, pp. 821–827. https://doi.org/10.17762/itii.v9i2.419
- Gadal S., Mokhtar R., Abdelhaq M., Alsaqour R., Ali E.S., Saeed R. Machine learning-based anomaly detection using K-mean array and sequential minimal optimization. Electronics, 2022, vol. 11, no. 14, pp. 2158. https://doi.org/10.3390/electronics11142158