Menu
Publications
2025
2024
2023
2022
2021
2020
2019
2018
2017
2016
2015
2014
2013
2012
2011
2010
2009
2008
2007
2006
2005
2004
2003
2002
2001
Editor-in-Chief
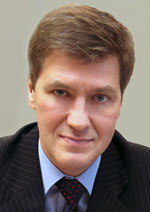
Nikiforov
Vladimir O.
D.Sc., Prof.
Partners
doi: 10.17586/2226-1494-2024-24-5-824-833
Classification of multiple sclerosis lesion through Deep Learning analysis of MRI images
Read the full article

Article in English
For citation:
Abstract
For citation:
Divya M., Dhilipan J., Saravanan A. Classification of multiple sclerosis lesion through Deep Learning analysis of MRI images. Scientific and Technical Journal of Information Technologies, Mechanics and Optics, 2024, vol. 24, no. 5, pp. 824–833. doi: 10.17586/2226-1494-2024-24-5-824-833
Abstract
Multiple Sclerosis (MS) is a progressive autoimmune disease affecting the central nervous system, causing communication disruptions between the brain and the body. Early and accurate detection of MS lesions in brain Magnetic Resonance Imaging (MRI) scans is crucial for effective treatment. This paper proposes MSNet, a deep learning-based approach for automatic detection and diagnosis of MS lesions from MRI images, leveraging Convolutional Neural Networks (CNNs) for precise lesion identification and classification. Our methodology involves a comprehensive analysis of MRI datasets, including preprocessing steps such as normalization and lesion segmentation. We propose a novel CNN architecture tailored for MS lesion detection, achieving an accuracy rate of 98.2 % on the test dataset. By incorporating advanced image recognition techniques, our system classifies MS lesions from diverse brain pathologies present in MRI images. The model also highlights MS lesions within the MRI images, aiding neuroradiologists in accurate diagnosis and treatment planning. This study contributes significantly to improving MS diagnosis by providing a reliable and automated tool for lesion detection and classification.
Keywords: multiple sclerosis, machine learning, MRI
References
References
- López-Dorado A., Pérez J., Rodrigo M.J., Miguel-Jiménez J.M., Ortiz M., de Santiago L., López-Guillén E., Blanco R., Cavalliere C., Morla E.M.S., Boquete L., Garcia-Martin E. Diagnosis of multiple sclerosis using multifocal ERG data feature fusion. Information Fusion, 2021, vol. 76, pp. 157–167. https://doi.org/10.1016/j.inffus.2021.05.006
- Mohseni E., Moghaddasi S.M. A hybrid approach for MS diagnosis through nonlinear EEG descriptors and metaheuristic optimized classification learning. Computational Intelligence and Neuroscience, 2022, vol. 2022, pp. 5430528. https://doi.org/10.1155/2022/5430528
- Ahmadi A., Davoudi S., Daliri M.R. Computer Aided Diagnosis System for multiple sclerosis disease based on phase to amplitude coupling in covert visual attention. Computer Methods and Programs in Biomedicine, 2019, vol. 169, pp. 9–18. https://doi.org/10.1016/j.cmpb.2018.11.006
- Karaca B.K., Akşahin M.F., Öcal R. Detection of multiple sclerosis from photic stimulation EEG signals. Biomedical Signal Processing and Control, 2021, vol. 67, pp. 102571. https://doi.org/10.1016/j.bspc.2021.102571
- De Santiago L., Morla E.M.S., Ortiz M., López E., Usanos C.A., Alonso-Rodríguez M.C., Barea R., Cavaliere-Ballesta C., Fernández A., Boquete L. A computer-aided diagnosis of multiple sclerosis based on mfVEP recordings. PLoS ONE, 2019, vol. 14, no. 4, pp. e0214662. https://doi.org/10.1371/journal.pone.0214662
- Yperman J., Becker T., Valkenborg D., Popescu V., Hellings N., Van Wijmeersch B., Peeters L.M. Machine learning analysis of motor evoked potential time series to predict disability progression in multiple sclerosis. BMC Neurology, 2020, vol. 20, no. 1, pp. 105. https://doi.org/10.1186/s12883-020-01672-w
- Solana E., Martinez-Heras E., Casas-Roma J., Calvet L., Lopez-Soley E., Sepulveda M., Sola-Valls N., Montejo C., Blanco Y., Pulido-Valdeolivas I., Andorra M., Saiz A., Prados F., Llufriu S. Modified connectivity of vulnerable brain nodes in multiple sclerosis, their impact on cognition and their discriminative value. Scientific Reports, 2019, vol. 9, pp. 20172. https://doi.org/10.1038/s41598-019-56806-z
- Kawahara J. Spinal Cord Segmentation and Disability Prediction in Multiple Sclerosis Using Novel Optimization and Machine Learning Methods: Ph.D. Dissertation. Vancouver Island University, Nanaimo, BC, Canada. 2013, 57 p.
- Zhang H., Alberts E., Pongratz V., Mühlau M., Zimmer C., Wiestler B., Eichinger P. Predicting conversion from clinically isolated syndrome to multiple sclerosis–An imaging-based machine learning approach. NeuroImage Clinical, 2019, vol. 21, pp. 101593. https://doi.org/10.1016/j.nicl.2018.11.003
- Rezaee A., Rezaee K., Haddadnia J., Gorji H.T. Supervised meta-heuristic extreme learning machine for multiple sclerosis detection based on multiple feature descriptors in MR images. SN Applied Sciences, 2020, vol. 2, no. 5, pp. 866. https://doi.org/10.1007/s42452-020-2699-y
- Ekşi Z., Özcan E.M., Çakıroğlu M., Öz C., Aralaşmak A. Differentiation of multiple sclerosis lesions and low-grade brain tumors on MRS data: Machine learning approaches. Neurological Sciences, 2021, vol. 42, no. 8, pp. 3389–3395. https://doi.org/10.1007/s10072-020-04950-0
- Peng Y., Zheng Y., Tan Z., Liu J., Xiang Y., Liu H., Dai L., Xie Y., Wang J., Zeng C., Li Y. Prediction of unenhanced lesion evolution in multiple sclerosis using radiomics-based models: A machine learning approach. Multiple Sclerosis and Related Disorders, 2021, vol. 53, pp. 102989. https://doi.org/10.1016/j.msard.2021.102989
- Eshaghi A., Young A.L., Wijeratne P.A., Prados F., Arnold D.L., Narayanan S., Guttmann C.R.G., Barkhof F., Alexander D.C., Thompson A.J., Chard D., Ciccarelli O. Identifying multiple sclerosis subtypes using unsupervised machine learning and MRI data. Nature Communications, 2021, vol. 12, no. 1, pp. 2078. https://doi.org/10.1038/s41467-021-22265-2
- Bonanno L., Mammone N., De Salvo S., Bramanti A., Rifici C., Sessa E., Bramanti P., Marino S., Ciurleo R. Multiple Sclerosis lesions detection by a hybrid Watershed-Clustering algorithm. Clinical Imaging, 2021, vol. 72, pp. 162–167. https://doi.org/10.1016/j.clinimag.2020.11.006
- Iswisi A.F.A., Karan O., Rahebi J. Diagnosis of Multiple Sclerosis Disease in Brain Magnetic Resonance Imaging Based on the Harris Hawks Optimization Algorithm. BioMed Research International, 2021, pp. 3248834. https://doi.org/10.1155/2021/3248834
- Jain S., Rajpal N., Yadav J. Supervised and unsupervised machine learning techniques for multiple sclerosis identification: A performance comparative analysis. Advances in Intelligent Systems and Computing, 2022, vol. 1374, pp. 369–381. https://doi.org/10.1007/978-981-16-3346-1_30
- Garcia-Martin E., Ortiz M., Boquete L., Sánchez-Morla E.M., Barea R., Cavaliere C., Vilades E., Orduna E., Rodrigo M.J. Early diagnosis of multiple sclerosis by OCT analysis using Cohen’s d method and a neural network as classifier. Computers in Biology and Medicine, 2021, vol. 129, pp. 104165. https://doi.org/10.1016/j.compbiomed.2020.104165
- Montolío A., Cegoñino J., Garcia-Martin E., Pérez del Palomar A. Comparison of machine learning methods using spectralis OCT for diagnosis and disability progression prognosis in multiple sclerosis. Annals of Biomedical Engineering, 2022, vol. 50, no. 5, pp. 507–528. https://doi.org/10.1007/s10439-022-02930-3