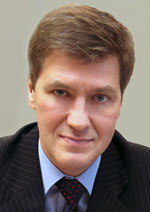
Nikiforov
Vladimir O.
D.Sc., Prof.
Construction of matched distance function for simple Markov channel
Read the full article

For citation:
Abstract
The problem of error correction in communication channel may be solved by finding the most probable error vector in the channel. The equivalent in some cases problem may be formulated as finding the vector of least weight. To perform this, the distance function is needed matched to communication channel. Hamming and Euclid metrics are traditionally used in classical coding theory, but for many channels the correspondent matched distance functions are unknown. Finding such functions would allow decoding error probability decreasing, and it is actual task. In this paper the problem of decoding function development is solved, providing maximum likelihood decoding in simple Markov channel. Analysis of vectors probability in simple Markov channel is performed. The developed function is presented as sum of coefficients from the set depending on channel parameters. The way of coefficient computation is mentioned, providing matching the function with channel. Some approximations of coefficients are given for the case when channel parameters are unknown or uncertain. Affect of this function and its approximations on error probability is estimated experimentally using convolutional code. The decoding rule is proposed providing maximum likelihood decoding in simple Markov channel. Proposed function is matched with the channel for all code lengths, as opposed to known Markov metrics. The selection of coefficients for the decoding rule function is considered, simplifying computations by cost of possible losing the matching property. Error probability of maximum likelihood decoding using proposed function is estimated experimentally for convolutional code in simple Markov channel. The affect of coefficients approximation on decoding error probability increasing is estimated. The comparison with the class of known Markov metrics is performed. Experiments show that both proposed matched function and its simplifications provide significant gain in decoding error probability comparing to Hamming metric, and comparing to known Markov metric in area of low a priori channel bit error probabilities. Usage of quantized values of proposed function practically does not increase the error probability comparing to maximum likelihood decoding. The method based on analysis of error probability in two-state channels may be used to develop decoding functions for more complex Gilbert and Gilbert–Elliott channel models. Such functions would allow significant increasing in data transmission reliability in channels with complicated noise structure and provide maximum likelihood decoding in Markov channel with memory, instead of traditional approach which uses decorrelation of the channel and significantly reduces capacity.
Acknowledgements. The article was prepared within the framework of the Basic Research Program at HSE University, Internet of Things and Cyber-Physical Systems Laboratory, Saint Petersburg School of Physics, Mathematics, and Computer Science.
References
- Krouk Е. А. Combinatorial Decoding of Linear Block Codes. St. Petersburg, SUAI Publ., 2007, 238 p. (in Russian)
- Bogatyrev A.V., Bogatyrev V.A., Bogatyrev S.V. The probability of timeliness of a fully connected exchange in a redundant real-time communication system. Proc. of the Wave Electronics and its Application in Information and Telecommunication Systems (WECONF), 2020, pp. 1–4. https://doi.org/10.1109/weconf48837.2020.9131517
- Bogatyrev V.A., Bogatyrev A.V., Bogatyrev S.V. Multipath transmission of heterogeneous traffic in acceptable delays with packet replication and destruction of expired replicas in the nodes that make up the path. Communications in Computer and Information Science, 2023, vol. 1748, pp. 104–121. https://doi.org/10.1007/978-3-031-30648-8_9
- Bogatyrev V.A., Bogatyrev S.V., Bogatyrev A.V. Control of multipath transmissions in the nodes of switching segments of reserved paths. Proc. of the International Conference on Information, Control, and Communication Technologies (ICCT), 2022, pp. 1–5. https://doi.org/10.1109/icct56057.2022.9976839
- Lin S., Li J. Fundamentals of Classical and Modern Error-Correcting Codes. Cambridge University Press, 2022, 840 p.
- Gabidulin E. A brief survey of metrics in coding theory. Proc. of the Mathematics of Distances and Applications, 2012, pp. 66–84
- Firer M., Walker J.L. Matched metrics and channels. IEEE Transactions on Information Theory. 2016, vol. 62, no. 3, pp. 1150–1156. https://doi.org/10.1109/TIT.2015.2512596
- D’Oliveira R., Firer M. Channel metrization. European Journal of Combinatorics, 2019, vol. 80, pp. 107–119. https://doi.org/10.1016/j.ejc.2018.02.026
- Qureshi C.M. Matched metrics to the binary asymmetric channels. IEEE Transactions on Information Theory, 2019, vol. 65, no. 2, pp. 1106–1112. https://doi.org/10.1109/TIT.2018.2885782
- Miyamoto G.A., Firer M. Obtaining binary perfect codes out of tilings. IEEE Transactions on Information Theory, 2020, vol. 66, no. 10, pp. 6121–6132. https://doi.org/10.1109/TIT.2020.2988865
- Cotardo G., Ravagnani A. Parameters of codes for the binary asymmetric channel. IEEE Transactions on Information Theory, 2022, vol. 68, no. 5, pp. 2941–2950. https://doi.org/10.1109/TIT.2022.3147593
- Zhang A., Jing X., Feng K. Optimal combinatorial neural codes with matched metric δr: characterization and constructions. IEEE Transactions on Information Theory, 2023, vol. 69, no. 8, pp. 5440–5448. https://doi.org/10.1109/TIT.2023.3266010
- Xu Y., Kan H., Han G. MacWilliams extension property with respect to weighted poset metric. IEEE Transactions on Information Theory, 2024, vol. 70, no. 2, pp. 995–1007. https://doi.org/10.1109/TIT.2023.3328262
- Xiao X., Vasic B., Lin S., Li J., Abdel–Ghaffar K. Quasi-cyclic LDPC codes with parity-check matrices of column weight two or more for correcting phased bursts of erasures. IEEE Transactions on Communications, 2021, vol. 69, no. 5, pp. 2812–2823. https://doi.org/10.1109/tcomm.2021.3059001
- Li L., Lv J., Li Y., Dai X., Wang X. Burst Error Identification Method for LDPC Coded Systems. IEEE Communications Letters, 2024, vol. 28, no. 7, pp. 1489–1493. https://doi.org/10.1109/lcomm.2024.3391826
- Ovchinnikov А.А., Veresova А.М., Fominykh А.А. Decoding of linear codes for single error bursts correction based on the determination of certain events. Informatsionno-upravliaiushchie sistemy [Information and Control Systems], 2022, no. 6, pp. 41–52. https://doi.org/10.31799/1684-8853-2022-6-41-52
- Isaeva M.N., Ovchinnikov A.A. Correction of single error bursts beyond the code correction capability using information sets. Scientific and Technical Journal of Information Technologies, Mechanics and Optics, 2024, vol. 24, no. 1, pp. 70–80. (in Russian). https://doi.org/10.17586/2226-1494-2024-24-1-70-80
- Alaroni Z., Huleihel B., Pfister H.D., Permuter H.H. Data-Driven polar codes for unknown channels with and without memory. Proc. of the IEEE International Symposium on Information Theory (ISIT), 2023, pp. 1–6. https://doi.org/10.1109/isit54713.2023.10206663
- Fang Y., Chen J. Decoding polar codes for a generalized Gilbert-Elliott channel with unknown parameter. IEEE Transactions on Communications, 2021, vol. 69, no. 10, pp. 6455–6468. https://doi.org/10.1109/TCOMM.2021.3095195
- Veresova A., Fominykh A., Ovchinnikov A. About usage of metrics in decoding of LDPC codes in Two-State channels with memory. Proc. of the XVII International Symposium Problems of Redundancy in Information and Control Systems (REDUNDANCY). 2021, pp. 1–6. https://doi.org/10.1109/REDUNDANCY52534.2021.9606474
- Veresova A., Ovchinnikov A. Usage of Markov Metric in decoding of convolutional codes in Two-State channels. Proc. of the IEEE 3rd International Conference on Problems of Informatics, Electronics and Radio Engineering (PIERE), 2024, pp. 1–5. https://doi.org/10.1109/piere62470.2024.10805044