Menu
Publications
2025
2024
2023
2022
2021
2020
2019
2018
2017
2016
2015
2014
2013
2012
2011
2010
2009
2008
2007
2006
2005
2004
2003
2002
2001
Editor-in-Chief
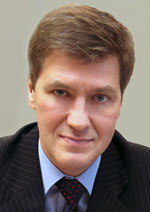
Nikiforov
Vladimir O.
D.Sc., Prof.
Partners
Summaries of the Issue
OPTICAL ENGINEERING
Multispectral optoelectronic system
Grigoriev Alexey V. , Demin Anatoliy V., Evgenii N. Sechak 1
Aerial photography equipment and space systems for remote sensing of the Earth’s surface make it possible to solve various problems in conditions of rapidly changing optical and physical parameters and flight dynamics. Despite its advantages, aerial photography has a number of disadvantages that limit its application in real conditions: the need for a high level of technology for obtaining an aerial photograph, a relatively long period of processing photographic materials in conditions of rapidly changing man-made processes in the monitoring zone. This article discusses the urgent task of creating a multispectral optical-electronic system (complex) for remote sensing of the Earth, which allows obtaining information about the characteristics of the surface in different spectral ranges, primarily in the visible and infrared. The main advantage of multispectral optical and optical-electronic complexes is the ability to work at any time of day or night and at any time of year. The article discusses the principle of constructing aviation integrated multispectral optical- electronic systems operating at an altitude of up to the stratosphere, and its main components. From a modern perspective, the possibilities and prospects for using such systems in various fields, including monitoring and control, are shown. A structural and functional diagram of the device is proposed, including independent channels for collecting, storing and transmitting information. The functional purpose of the experimental sample is to search for and detect objects below the clouds in the infrared range. The visible range channel performs the function of orienting the operator’s visual perception in space and obtaining an image of the object. A laser communication channel is provided for transmitting the collected information. Studies of the experimental sample of the aviation two-channel optical-electronic complex, structurally implemented as an integrated technical system with independent channels and operating in the visible and infrared spectral regions, showed high accuracy and efficiency of the system. The accuracy of the stabilization system was about 7·10–9 s–1, the range in the infrared range is at least 150 km, the required exposure time is no more than 2 s. The results of the work can be used for further development and improvement of multispectral optical-electronic systems for remote sensing of the Earth.
Study of the influence of laser wavelength on the dichroism effect in ZnO:Ag films
Gresko Vladislav R., Sergeev Maxim M. , Dolgopolov Arthur D. , Sokura Liliia A. , Grigoryev Evgeniy A. 9
ZnO:Ag films are used as photoabsorbing layers in plasmonic photodetectors. The use of laser radiation in the manufacture of photodetectors allows one to control the parameters of the plasmon resonance peak and change the range of spectral sensitivity of the device. Known studies on laser action on similar photoabsorbing films with nanoparticles pay little attention to the dichroism effect arising as a result of laser action. In the presence of dichroism, the efficiency of a plasmonic photodetector depends on the polarization of the detected radiation. The aim of this work is to study the dichroism effect arising in ZnO:Ag films under the action of femtosecond laser radiation with wavelengths near the plasmon resonance of nanoparticles and far from it. To obtain the dichroism effect in the films, laser pulses with a wavelength near the plasmon resonance of nanoparticles (515 ± 5 nm) and far from it (1030 ± 5 nm) were used. Linearly polarized pulses of 224 ± 15 fs duration and 200 kHz repetition rate were used. Transmission spectra of linearly polarized light by areas of ZnO:Ag films modified by laser radiation were obtained using a spectrophotometer microscope. The size, concentration, shape and arrangement of nanoparticles in the films, and the surface morphology of zinc oxide (ZnO) films were studied using electron microscopy methods. It was shown that laser radiation with a wavelength near the plasmon resonance of nanoparticles with an energy density in a pulse higher than 43 ± 0.5 mJ/cm2 leads to the appearance of a dichroism effect in the films. The occurrence of this effect is associated with the reorientation of nanoparticles. Laser action leads to a reorientation of the initially chaotic arrangement of nanoparticles in the direction parallel to the polarization vector of the laser radiation. The highest value of the linear dichroism is achieved in the region of plasmon resonance wavelengths of 515 ± 5 nm at a radiation energy density of 66 ± 0.5 mJ/cm2. A further increase in the energy density leads to a decrease in dichroism due to the return of chaotic orientation. The effect of radiation with a wavelength far from the plasmon resonance of 1030 ± 5 nm with equivalent energy densities does not lead to a reorientation of nanoparticles and, as a consequence, the change in the linear dichroism value is significantly lower. According to the proposed hypothesis, the differences between the results of laser exposure are associated with different mechanisms of radiation absorption in the material. Radiation with a wavelength of 515 ± 5 nm is absorbed by nanoparticles. In the case of linear polarization of radiation, ionization of nanoparticles and their reorientation parallel to the polarization vector occur. At a wavelength of 1030 ± 5 nm, radiation is absorbed by the ZnO matrix. This leads to heating of the film, heat transfer to the nanoparticles, as a result of which the process of reorientation of nanoparticles parallel to the polarization vector is complicated, and the dichroism effect is much less pronounced. The results of the study can be used in the design and manufacture of photodetectors due to the identified possibility of shifting the peak of plasmon resonance of nanoparticles in the photoabsorbing layer of the photodetector. Control of the dichroism effect allows controlling the sensitivity range of detectors.
Direct laser thermochemical writing on titanium films for rasterized images creation
Pivovarov Artem D., Usynina Ekaterina V. , Dmitry A. Sinev 23
Creating greyscale photomasks is an uncommon technical problem which in some cases can be solved by rasterization. At the same time, existing works on the direct laser thermochemical recording show the possibility of forming local areas of transparency as a result of oxidation of thin metal films, but the final contrast of the transmittance coefficient of the resulting structure turns out to be difficult to predict due to the complexity of the web of influencing factors. In the present work, we propose an experimental approach to combining the methods of greyscale thermochemical recording and rasterizing by creating structures with controlled transparency on titanium films which can form the basis for recording topologies of rasterized photomasks. The samples used in this study were thin (20–40 nm) titanium films which were treated using the Minimarker-2 technological complex based on a fiber ytterbium laser. Direct recording with a scanning focused beam was performed using a built-in system of galvanometric scanners. The optical and geometric characteristics of the recorded structures were analyzed using an optical microscope. The experimentally determined recording modes were confirmed by semi-analytical temperature modeling. It is shown that the formation of contrast structures occurs in the ranges of power densities about 15–140 MW/m2 when scanning at speeds from 0.1 to 1 mm/s, and the change in the contrast of the structures is achieved at power densities of about 50–90 MW/m2. The contrast of the transmission coefficient of the recorded structures relative to the initial value of the film transparency is controlled to vary from 1 to 40 %. In a number of regimes, the formation of periodic structures with a period of about 0.71 μm was revealed, leading to diffraction effects observed in reflected light. The paper presents theoretically modeled and experimentally confirmed modes of recording structures under the influence of nanosecond radiation. It is shown that varying the parameters of the effect allows localizing oxidation regions, which leads to a change in the contrast of the transmitted light and allows creating halftone rasterized images with specified values of grayscale in the transmitted light. The practical significance of the obtained results is demonstrated by the example of recording an optical element such as a halftone rasterized photomask with a specified geometry and contrast values.
AUTOMATIC CONTROL AND ROBOTICS
Algorithms of direct output-feedback adaptive control of a linear system with finite time tuning
Gerasimov Dmitriy N, Podoshkin Dmitry L. , Nikiforov Vladimir O. 33
A problem of direct model reference adaptive control of parametrically uncertain systems with inaccessible for measurement state is considered in this paper. With the purpose of adaptive tuning of the controller parameters, a modification of the gradient adaptation algorithm with finite time convergence is proposed. The modification is implemented by periodic recalculation of the adjustable parameters and further their replacement in the integrators of the gradient adaptation algorithm. Preliminary calculation is accomplished under condition of interval excitation based on prediction of the adaptation algorithm dynamics; hence the controller parameters are identified precisely. The control problem is solved with the use of augmented error approach and certainty equivalence principle. Analysis of the closed-loop system is made using the Lyapunov functions method. The modification ensures parametric convergence under interval excitation condition which is weaker than the persistent excitation one, sensitive to variations of the unknown parameters, and, in comparison with the variety of analogous solutions, does not require the dynamic order increasing. The other distinguishing feature of the algorithm is opportunity of its use in schemes of both indirect and direct adaptation.
COMPUTER SCIENCE
Large language models in information security and penetration testing: a systematic review of application possibilities
Konev Anton A., Payusova Tatyana I. 42
The development of artificial intelligence technologies, in particular, large language models (LLM), has led to changes in many areas of human life and activity. Information security (IS) has also undergone significant changes. Penetration testing (pentest) allows evaluating the security system in practice in “combat” conditions. LLMs can take practical security analysis to a qualitatively new level in terms of automation and the ability to generate non-standard attack patterns. The presented systematic review is aimed at determining the already known ways of applying LLM in cybersecurity, as well as identifying “blank spots” in the development of technology. The selection of literature sources was carried out in accordance with the multi-stage PRISMA guidelines based on the analysis of abstracts and keywords of publications. The resulting sample was supplemented using the “snowball” method and manual search of articles. The total number of publications was 50 works from January 2023 to March 2024. The conducted research allowed to analyze the ways of using LLM in the field of information security (goal setting and decision-making support, pentest automation, security analysis of LLM models and program code), determine the LLM architectures (GPT-4, GPT-3.5, Bard, LLaMA, LLaMA 2, BERT, Mixtral 8×7B Instruct, FLAN, Bloom) and software solutions based on LLM used in the field of information security (GAIL-PT, AutoAttacker, NetSecGame, Cyber Sentinel, Microsoft Counterfit, GARD project, GPTFUZZER, VuRLE), to establish limitations (finite “lifetime” of data for LLM training, insufficient cognitive abilities of language models, lack of independent goal setting and difficulties in adapting LLM to new task parameters), identify potential growth points and development of technology in the context of cyber defense (elimination of “hallucinations” of models and ensuring protection of LLM from jailbreaks, implementation of integration of known disparate solutions and software automation of tasks in the field of information security using LLM). The presented results can be useful in developing theoretical and practical solutions, educational and training datasets, software packages and tools for penetration testing, new approaches to building LLM and improving their cognitive abilities, taking into account aspects of working with jailbreaks and “hallucinations”, as well as for independent further multilateral study of the issue.
Usage of polar codes for fixed and random length error bursts correction
Andrei A. Ovchinnikov 53
Error correction during data storage, processing, and transmission allows for ensuring data integrity. Channel coding techniques are used to counteract these errors. Noise in real systems is often correlated, whereas traditional coding and decoding approaches are based on decorrelation which in turn reduces the performance limits of channel coding. Polar codes, adopted as a coding scheme in the modern fifth-generation communication standard, demonstrate low error probabilities during decoding in memoryless channels. The current task is to investigate the suitability of polar codes for channels with memory, analyze their burst error-correcting capabilities, and compare them with known error- correcting coding methods. To evaluate burst error-correcting capability, the method of calculating the ranks of each submatrix of the parity-check matrix of a fixed-size polar code is used. The burst error-correcting capability of polar codes can be improved through a proposed interleaving procedure. The analysis of the burst error-correcting capability is carried out for short-length polar codes. An analysis of the burst error-correcting capability of polar codes has been performed. A comparison of burst error-correcting capabilities of polar codes with codes defined by random generator matrix, Gilbert codes and low-density parity-check codes was conducted. An analysis of the decoding error probability shows that standard polar code decoding algorithms do not achieve low error probabilities. The same decoding error probability 0.01 as for Gilbert channel is achieved by polar code in binary symmetric channel with an unconditional error probability two times as high. From the analysis, it can be concluded that the burst error-correcting capability of standard polar codes is low. The proposed interleaving approach improves the burst error-correcting capability and allows achieving values close to the Reiger bound. Further research directions may include developing decoding algorithms for polar codes adapted for channels with variable packet lengths
Efficient sparse retrieval through embedding-based inverted index construction
Dobrynin Viacheslav Yu. , Abramovich Roman K. , Alexey V. Platonov 61
Modern search engines use a two-stage architecture for efficient and high-quality search over large volumes of data. In the first stage, simple and fast algorithms like BM25 are applied, while in the second stage, more precise but resource- intensive methods methods, such as deep neural networks, are employed. Although this approach yields good results, it is fundamentally limited in quality due to the vocabulary mismatch problem inherent in the simple algorithms of the first stage. To address this issue, we propose an algorithm for constructing an inverted index using vector representations combining the advantages of both stages: the efficiency of the inverted index and the high search quality of vector models. In our work, we suggest creating a vector index that preserves the various semantic meanings of vocabulary tokens. For each token, we identify the documents in which it is used, and then cluster its contextualized embeddings. The centroids of the resulting clusters represent different semantic meanings of the tokens. This process forms an extended vocabulary which is used to build the inverted index. During index construction, similarity scores between each semantic meaning of a token and documents are calculated which are then used in the search process. This approach reduces the number of computations required for similarity estimation in real-time. Searching the inverted index first requires finding keys in the vector index, helping to solve the vocabulary mismatch problem. The operation of the algorithm is demonstrated on a search task within the SciFact dataset. It is shown that the proposed method achieves high search quality with low memory requirements. The proposed algorithm demonstrates high search quality, while maintaining a compact vector index whose size remains constant and depends only on the size of the vocabulary. The main drawback of the algorithm is the need to use a deep neural network to generate vector representations of queries during the search process which slows down this stage. Finding ways to address this issue and accelerate the search process represents a direction for future research.
Method of semantic segmentation of airborne laser scanning data of water protection zones
Sergey V. Sai , Alexey V. Zinkevich 68
This article presents an evaluation of the efficiency of a neural network method for the semantic segmentation of three-dimensional point clouds obtained using the Geoscan 401 Lidar UAV. The proposed implementation of the neural network is based on the PointNet++ deep learning model which directly processes point clouds. A technique has been developed for acquiring and preparing a dataset with four classes: land, vegetation, vehicles, and construction objects. To increase the accuracy of the evaluation, a technique based on augmentation and redistribution of the datasets has been proposed. The neural network model consists of hierarchically constructed blocks that perform sampling, grouping, and feature extraction. Adjusting the number of blocks and setting the search radius for local features affects both the accuracy of segmentation and computational costs. The efficiency of the method for semantic segmentation of three-dimensional point clouds obtained using the Geoscan 401 Lidar UAV has been evaluated. The augmentation and redistribution technique improved the average Intersection over Union (IoU) value by at least 35 %. For the obtained data, the optimal radius in the grouping layer was determined, ensuring a balance between detail and sensitivity. It was found that an increase in the number of points in the dataset does not lead to a significant improvement in accuracy; however, the diversity of the datasets used enhances the method efficiency. The developed dataset increases the effectiveness of the applied approach, including when training other models. The results of this study indicate the potential for using the proposed methods and algorithms in constructing digital models of the Amur River and its main tributaries.
Directional variance-based algorithm for digital image smoothing
Al-Ameen Zohair 78
Image smoothing is vital in image processing as it attenuates the texture and unnecessary high-frequency components and provides a smooth image with a preserved structure to facilitate subsequent operations or analysis. Smoothed images are required in many image processing applications, such as details boost, sharpening, High Dynamic Range imaging, edge detection, stylization, abstraction, etc. Still, not all existing smoothing methods are successful in this task, as some undesirable problems may be introduced, such as removing significant details, introducing excessive blurring, processing flaws, halos, and other artifacts. Thus, the opportunity still stands to provide a new algorithm that smooths an image efficiently. This study concisely explores smoothing via the Directional Variances (DV) concept. The proposed algorithm leverages the DV concept to minimize energy, seeking a balance between essential structural preservation and smoothness. The proposed algorithm iteratively smooths the image using DV, diffusion, regularization, and energy minimization. A thorough evaluation is conducted on diverse images, showcasing the effectiveness of the developed algorithm. The results demonstrate that the developed DV-based algorithm has superb abilities in smoothing different images while preserving structural details, making it a valuable tool for various applications in digital image processing.
87
A new method of generating model sets of Distributed Acoustic Sensing (DAS) signals of different classes is proposed. Statistical characteristics of model signals are quite similar to real DAS-signals of corresponding classes and can be used for sharp improvement of DAS-signals processing quality by machine learning methods. The proposed method is a modification of the Generative Adversarial Network (GAN) technique. The novelty of the approach lies in the introduction of an additional external control loop for the performance of the generative network which includes a classifier trained on an available (small) corpus of real DAS signals. A method for generating model sets of DAS signals based on GAN technology is proposed, and it differs from the classical technology by the presence of an additional external quality control loop. An optimality criterion for the generating system is formulated, the optimum of which is achieved by step-by-step reconfiguration of the GAN neural network structure. Reconfiguration is based on the Nelder- Mead optimization method. A software implementation of the proposed solution architecture on the Python platform is developed and tested on real data. Results are presented proving the practical efficiency of the proposed method. In particular, the proposed method allowed to increase the capacity of the training dataset and, thus, to increase the resulting reliability of the classification of target DAS signals. The developed approach is promising for use in cases where the capacity of the datasets provided for training is insufficient to ensure highly reliable classification.
Multidimensional trajectory planning algorithm for a 5D printer slicer
Afanasiev Maksim Ya , Solovev Michael R. , Krylova Anastasiia A., Shorokhov Sergey A., Fedosov Yuri V. 95
The article presents a trajectory planning algorithm for a 5D printer to solve problems that arise in traditional 3D printing. Standard 3D printing methods using layer-by-layer material deposition lead to anisotropy of mechanical properties, where the object strength depends on the direction of the layer application. This limits the ability to create isotropic- strength parts, especially those with complex geometry. The goal of the study is to develop an algorithm that enables uniform distribution of the mechanical properties of the object by optimizing the printing trajectories. The proposed algorithm is based on constructing trajectories using spherical spiral layers. The algorithm considers changing printing parameters, such as layer height and line thickness, and adapts to various geometric shapes of the object. A key feature is ensuring isotropy of the part properties by evenly distributing the material along the trajectories. The algorithm also includes the construction of normals at each point of the curve to accurately direct the movement of the printing head. This approach avoids the standard limitations typical of 3D printing. The algorithm was tested on various models, including simple and complex geometric shapes with high curvature. During computer modeling, experiments were conducted with different layer heights and line thicknesses, which allowed for the assessment of the influence of these parameters. The algorithm demonstrated high convergence under various input conditions, ensuring accurate trajectory execution regardless of initial parameters. The trajectories and normals were visualized, confirming the correct print direction and even material deposition. For further work convenience, an intermediate trajectory representation format was developed which is easily converted into G-codes. This allows data to be prepared for future physical experiments that will be conducted to assess the algorithm effectiveness in real printing conditions. The multidimensional trajectory planning algorithm opens up new possibilities for additive manufacturing, enabling the creation of complex objects with improved mechanical properties without the need for additional supports. The practical significance of the algorithm lies in its application in areas, such as aerospace, automotive, and medicine, where both complex geometric shapes and high part strength are important. Further research may focus on expanding the algorithm capabilities to work with various materials and adjusting printing parameters to improve the performance and quality of printed parts.
Scheduling distributed computations in non-deterministic systems
Nikolay V. Kolesov, Elizaveta G. Litunenko, Tolmacheva Marina V., Tiulnikov Viktor S. 106
Computation scheduling is very important in the design of distributed information processing and control systems. Effective scheduling algorithms allow developer to find technical solutions that are adequate to the existing constraints. This is especially important for computers located on autonomous carriers, such as unmanned aerial vehicles, autonomous underwater vehicles, and other vehicles. Scheduling algorithms for tasks in the distributed non-deterministic computing system, when the task execution time is known inaccurately and described as time interval, are proposed and researched. The solution of the problem is achieved by reducing it to a known problem of flow shop scheduling with subsequent application of the formalism of solvable classes of distributed computing systems. Authors propose two algorithms for scheduling tasks for a non-deterministic distributed computing system. Algorithms allow the absence of isomorphisms between task graphs and the graph of interprocessor communications for the system, and the presence of many information outputs and branches between tasks. In these conditions, it is impossible to use known algorithms of flow shop scheduling. Proposed algorithms assume preliminary reduction of the considered system to the required form and base on the provisions of interval analysis and the concept of a solvable class of distributed computing systems. Optimality criterion for proposed algorithms is the criterion of minimum average time a task remains in the system. Additionally, the criterion of minimum of maximum deviation from directive terms is used. For the introduced solvable classes of systems, optimal scheduling algorithms of polynomial complexity are proposed. These algorithms allow us to schedule computations in real distributed computing systems when the system deviates from the canonical form and when the durations of the tasks are not precisely known. Proposed algorithms can be applied when scheduling computations in real distributed computing systems and solving tasks with not precisely known durations and also, for example, in scheduling economic processes.
Enhancing and extending CatBoost for accurate detection and classification of DoS and DDoS attack subtypes in network traffic
Hajjouz Abdulkader , Avksentieva Elena Yu. 114
In the ever changing digital world, the rise of sophisticated cyber threats, especially DoS and DDoS attacks, is a big challenge to Information Security. This paper addresses the problem of classifying malicious from benign network traffic using CatBoost classifier, a machine learning algorithm optimized for categorical data and imbalanced datasets. We used CIC-IDS2017 and CSE-CIC-IDS2018 datasets which simulate various cyberattack scenarios, our research optimized CatBoost to identify specific subtypes of DoS and DDoS attacks including Hulk, SlowHTTPTest, GoldenEye, Slowloris, HOIC, LOIC-UDP-HTTP, LOIT. The methodology involved data preparation, feature selection and model configuration, normalizing outliers, correcting negative values, and refining dataset structures. Stratified sampling ensured a balanced representation of classes in training, validation, and testing sets. The CatBoost model performed well with overall accuracy of 0.999922, high precision, recall, and F1-scores across all categories, and it can process over 3.4 million samples per second. These results show the model is robust and reliable for real-time intrusion detection. By classifying specific attack types, our model improves the precision of the Intrusion Detection Systems (IDS) and allows for targeted response to different threats. The big gain in detection accuracy solves the problem of imbalanced datasets and the need for granular attack types detection. Use CatBoost in advanced Information Security frameworks for critical infrastructure, cloud services, and enterprise networks to defend against digital threats. This paper provides a fast, accurate and scalable solution for network IDS and shows the importance of custom machine learning models in Information Security. Future work should explore CatBoost on more datasets and integrate it with other machine learning techniques to improve robustness and detection.
Detection of L0-optimized attacks via anomaly scores distribution analysis
Esipov Dmitry A. , Basov Mark I., Kletenkova Alyona D. 128
The spread of artificial intelligence and machine learning is accompanied by an increase in the number of vulnerabilities and threats in systems implementing such technologies. Attacks based on malicious perturbations pose a significant threat to such systems. Various solutions have been developed to protect against them, including an approach to detecting L0- optimized attacks on neural image processing networks using statistical analysis methods and an algorithm for detecting such attacks by threshold clipping. The disadvantage of the threshold clipping algorithm is the need to determine the value of the parameter (cutoff threshold) to detect various attacks and take into account the specifics of the data sets, which makes it difficult to apply in practice. This article describes a method for detecting L0-optimized attacks on neural image processing networks through statistical analysis of the distribution of anomaly scores. To identify the distortion inherent in L0-optimized attacks, deviations from the nearest neighbors and Mahalanobis distances are determined. Based on their values, a matrix of pixel anomaly scores is calculated. It is assumed that the statistical distribution of pixel anomaly scores is different for attacked and non-attacked images and for perturbations embedded in various attacks. In this case, attacks can be detected by analyzing the statistical characteristics of the distribution of anomaly scores. The obtained characteristics are used as predictors for training anomaly detection and image classification models. The method was tested on the CIFAR-10, MNIST and ImageNet datasets. The developed method demonstrated the high quality of attack detection and classification. On the CIFAR-10 dataset, the accuracy of detecting attacks (anomalies) was 98.43 %, while the binary and multiclass classifications were 99.51 % and 99.07 %, respectively. Despite the fact that the accuracy of anomaly detection is lower than that of a multiclass classification, the method allows it to be used to distinguish fundamentally similar attacks that are not contained in the training sample. Only input data is used to detect and classify attacks, as a result of which the proposed method can potentially be used regardless of the architecture of the model or the presence of the target neural network. The method can be applied for detecting images distorted by L0-optimized attacks in a training sample.
MODELING AND SIMULATION
Numerical study of SiO2 particle erosion of an aluminum alloy
Strokach Evgeny A. , Pozhidaev Alexei A. 140
Computational methods used to simulate solid particle erosion have advanced so far being able to estimate partial effect of various processing factors on microlevel, such as particle-surface contact, its particle material, and shape, etc. The published activities taken to study different effects in this aspect for popular aluminium or titanium alloys and steels still have a gap in knowledge addressing some process parameters. The influence of particle rotation and its direction on stress-strain state and wear depth is still understudied. The impact of friction consideration in relatively high-speed contacts should also be studied, as well as the surface layer heating effect which may influence the material strength properties when the temperatures get high. Understanding these effects would increase the predictive ability of the erosion model and its accuracy — which is presented in our 2D simulation study for SiO2 solid particles and a widespread Al6061-T6 alloy. The elastic-plastic and failure properties of the surface material were presented by the Johnson-Cook model. To estimate the influence of multiple impacts on the stress-strain state, three sequential rigid impacts of 250 µm particles at 45° and 155 m/s were modeled. Main attention was driven to the evolution of equivalent von-Mises stresses in the sample after each impact and its dependence on the friction and rotation of particles. It was shown that the effect of friction can be noticed after the first impact, remaining high throughout the simulation. Whereas the influence of rotation direction at 1000 rpm was noticeable after the second impact and tended to increase after the third impact. It is assumed that for other 6000 series aluminium alloys being eroded by spherical SiO2 particles with differing diameters the erosive behavior would keep. However, future studies should be addressed to the analyzing of non-spherical particles rotation, consideration of particle deformation, and to studies of these parameters including more impacts and different contact properties in complex.
An approach to solving the problem of geomagnetic data scarcity in decision-making support
Vorobeva Gulnara R. , Vorobev Andrei V. , Farvaev Emil F. 151
One of the main problems of using data in decision-making support is their scarcity in certain spatial points/areas due to the inability to carry out appropriate measurements. An example is the Earth’s magnetic field data (geomagnetic data) which is used to make decisions to reduce the extreme geophysical events negative impact on objects and systems of the technosphere (power lines, communication systems, railway automation, etc.). An analysis of the existing geomagnetic data collection infrastructure from the standpoint of system analysis made it possible to identify incomplete coverage of the monitoring network, which negatively affects decision-making to ensure technosphere security in the relevant spatial areas. Using the example of geomagnetic data, it was revealed that the known interpolation methods, which do not take into account the features of the spatiotemporal characteristics of the processes described by the data and their dependence on external factors, do not deal effectively with the task. To solve this problem, an approach to adaptive spatial interpolation is proposed the main idea of which is the dynamic selection of interpolation methods that are most effective for various factors. For an example of geomagnetic data two factors were chosen: the affiliation of a spatial point to a certain latitude zone and the index of geomagnetic activity in the time period under consideration. To evaluate the proposed solution, a prototype of a web-based application was developed. The experiment was conducted using geomagnetic information from the SuperMAG project. The proposed approach has proved to be more effective than using any separate interpolation method when comparing the root-mean-square errors. Adaptive interpolation proposed in this paper can be used in systems implementing interpolation of geospatial data, as an alternative to standard interpolation methods, in order to increase the accuracy of data recovery. When working with geomagnetic data, the factors considered in this work (latitudinal zones and geomagnetic activity) can be used, but interpolation of data of a different nature will require preliminary analysis to identify significant factors.
Construction of matched distance function for simple Markov channel
Veresova Alina M. , Andrei A. Ovchinnikov 160
The problem of error correction in communication channel may be solved by finding the most probable error vector in the channel. The equivalent in some cases problem may be formulated as finding the vector of least weight. To perform this, the distance function is needed matched to communication channel. Hamming and Euclid metrics are traditionally used in classical coding theory, but for many channels the correspondent matched distance functions are unknown. Finding such functions would allow decoding error probability decreasing, and it is actual task. In this paper the problem of decoding function development is solved, providing maximum likelihood decoding in simple Markov channel. Analysis of vectors probability in simple Markov channel is performed. The developed function is presented as sum of coefficients from the set depending on channel parameters. The way of coefficient computation is mentioned, providing matching the function with channel. Some approximations of coefficients are given for the case when channel parameters are unknown or uncertain. Affect of this function and its approximations on error probability is estimated experimentally using convolutional code. The decoding rule is proposed providing maximum likelihood decoding in simple Markov channel. Proposed function is matched with the channel for all code lengths, as opposed to known Markov metrics. The selection of coefficients for the decoding rule function is considered, simplifying computations by cost of possible losing the matching property. Error probability of maximum likelihood decoding using proposed function is estimated experimentally for convolutional code in simple Markov channel. The affect of coefficients approximation on decoding error probability increasing is estimated. The comparison with the class of known Markov metrics is performed. Experiments show that both proposed matched function and its simplifications provide significant gain in decoding error probability comparing to Hamming metric, and comparing to known Markov metric in area of low a priori channel bit error probabilities. Usage of quantized values of proposed function practically does not increase the error probability comparing to maximum likelihood decoding. The method based on analysis of error probability in two-state channels may be used to develop decoding functions for more complex Gilbert and Gilbert–Elliott channel models. Such functions would allow significant increasing in data transmission reliability in channels with complicated noise structure and provide maximum likelihood decoding in Markov channel with memory, instead of traditional approach which uses decorrelation of the channel and significantly reduces capacity.
BRIEF PAPERS
Application of the dynamic regressor extension and mixing approach in machine learning on the example of perceptron
Margun Alexei A., Zimenko Konstantin A., Bobtsov Alexey A. 169
This paper explores the application of the Dynamic Regressor Extension and Mixing method to improve the learning speed in machine learning tasks. The proposed approach is demonstrated using a perceptron applied to regression and binary classification problems. The method transforms a multi-parameter optimization problem into a set of independent scalar regressions, significantly accelerating the convergence of the algorithm and reducing computational costs. Results from computer simulations, including comparisons with stochastic gradient descent and Adam methods, confirm the advantages of the proposed approach in terms of convergence speed and computational efficiency.
WaveVRF: post-quantum verifiable random function based on error-correcting codes
Zhan-Mishel N. Dakuo 174
This paper introduces a novel Verifiable Random Function (VRF) based on the syndrome decoding problem and Wave signature, resistant to quantum computer attacks. The primary goal of this work is to present a new VRF scheme that demonstrates the applicability of the syndrome decoding problem for constructing cryptographically robust solutions. The paper describes the core VRF algorithms (KeyGen, VRFEval, VRFVerify) and highlights its essential properties: provability, uniqueness, and pseudo-randomness.