Меню
Публикации
2024
2023
2022
2021
2020
2019
2018
2017
2016
2015
2014
2013
2012
2011
2010
2009
2008
2007
2006
2005
2004
2003
2002
2001
Главный редактор
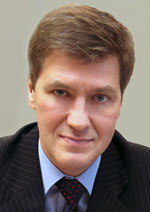
НИКИФОРОВ
Владимир Олегович
д.т.н., профессор
Партнеры
УДК 004.9
Анагностопулос Т., Хоружников С.Э., Грудинин В.А., Скоурлас К.
Читать статью полностью
РАСПОЗНАВАНИЕ И ПРОГНОЗИРОВАНИЕ ДЛИТЕЛЬНЫХ ЭМОЦИЙ В РЕЧИ (на англ. языке)
Читать статью полностью

Язык статьи - английский
Аннотация
Аннотация
Люди действуют рационально, и это их фундаментальное отличие от других видов жизни. Кроме того, в современной психологии подчеркивается, что люди как разумные создания отличаются чувствами и эмоциями. Существует пятнадцать видов универсальных длительных эмоций, плюс нейтральное эмоциональное состояние, такие как гнев, злость, паника, страх, тревога, отчаяние, грусть, восторг, радость, интерес, скука, стыд, гордость, отвращение, презрение и нейтральное отношение. В данном исследовании рассматривается понимание эмоционального состояния человека по анализу речи в процессе общения. Доказано, что на основе достаточного объема акустических данных эмоциональное состояние человека может быть классифицировано набором мажоритарных классификаторов. Предложенный набор классификаторов построен на основе трех базовых классификаторов: kNN, C4.5 и SVMRBFKernel. Этот набор обеспечивает лучшую обработку классификаций эмоций, чем каждый из базовых классификаторов в отдельности. Он сравнивается с двумя другими наборами классификаторов: один-против-всех (OAA) мультиклассовый SVM с гибридными ядрами и с набором классификаторов, состоящим из двух базовых классификаторов С5.0, и нейронная сеть (NeuralNetwork). Предложенный вариант достигает лучшего результата, чем два других набора классификаторов. В настоящей статье осуществляется классификация эмоций набором мажоритарных классификаторов, который состоит из трех определённых базовых классификаторов, имеющих низкую вычислительную сложность. Базовые классификаторы базируются на различных теоретических данных с целью избегания отклонений и избыточности, что дает предложенному набору классификаторов возможность обобщиться в пространство определений эмоций.
Ключевые слова: распознавание эмоций в речи, расчет эмоций, машинное обучение
Благодарности. Исследования проводились при финансовой поддержке Министерства образования и науки Российской Федерации в рамках Соглашения о предоставлении субсидии №14.575.21.0058.
Список литературы
Благодарности. Исследования проводились при финансовой поддержке Министерства образования и науки Российской Федерации в рамках Соглашения о предоставлении субсидии №14.575.21.0058.
Список литературы
1. Matthews G., Zeidner M., Roberts R.D. Emotional Intelligence: Science and Myth. Cambridge, MIT Press,
2003, 697 p.
2. Schacter D.L. Psychology. 2nd ed. NY, Worth Publishers, 2011, 624 p.
3. Gaulin S.J.C., McBurney D.H. Psychology: An Evolutionary Approach. Upper Saddle River, Prentice Hall,
2003.
4. Scherer K.R. Vocal communication of emotion: a review of research paradigms. Speech Communication,
2003, vol. 40, no. 1–2, pp. 227–256. doi: 10.1016/S0167-6393(02)00084-5
5. Thompson E.R. Development and validation of an internationally reliable short-form of the positive and negative
affect schedule (PANAS). Journal of Cross-Cultural Psychology, 2007, vol. 38, no. 2, pp. 227–242.
doi: 10.1177/0022022106297301
6. Parkinson B., Simons G. Worry spreads: interpersonal transfer of problem-related anxiety. Cognition and
Emotion, 2012, vol. 26, no. 3, pp. 462–479. doi: 10.1080/02699931.2011.651101
7. Picard R.W. Affective Computing. Cambridge, MIT Press, 2000, 304 p.
8. Duda R.O., Hart P.E., Stork D.G. Pattern Classification. NY, John Wiley and Sons, 2000, 735 p.
9. Rong J., Chen Y.-P.P. Chowdhury M., Li G. Acoustic features extraction for emotion recognition. Proc. 6th
IEEE/ACIS International Conference on Computer and Information Science, ICIS 2007, 2007, art. 4276418,
pp. 419–424. doi: 10.1109/ICIS.2007.48
10. Meng H., Pittermann J., Pittermann A., Minker W. Combined speech-emotion recognition for spoken human-
computer interfaces. Proc. IEEE International Conference on Signal Processing and Communications,
2007, art. 4728535, pp. 1179–1182. doi: 10.1109/ICSPC.2007.4728535
11. Shami M.T., Kamel M.S. Segment-based approach to the recognition of emotions in speech. Proc. IEEE International
Conference on Multimedia and Expo, ICME 2005, 2005, vol. 2005, art. 1521436, pp. 366–369.
doi: 10.1109/ICME.2005.1521436
12. Sato N., Obuchi Y. Emotion recognition using mel-frequency cepstral coefficients. Journal of Natural Language
Processing, 2007, vol. 14, no. 4, pp. 83–96. doi: 10.5715/jnlp.14.4_83
13. Grimm M., Mower E. Kroschel K., Narayanan S. Combining categorical and primitives-based emotion
recognition. Proc. 14th European Signal Processing Conference. Florence, Italy, 2006, pp. 345–357.
14. Kim S., Georgiou P.G., Lee S., Narayanan S. Real-time emotion detection system using speech: multi-modal
fusion of different timescale features. Proc. 9th IEEE International Workshop on Multimedia Signal Processing,
MMSP 2007. Chania, Crete, 2007, art. 4412815, pp. 48–51. doi: 10.1109/MMSP.2007.4412815
15. Sethu V., Ambikairaja E., Epps J. Phonetic and speaker variations in automatic emotion classification. Proc.
Annual Conference of the International Speech Communication Association, Interspeech. Brisbane, Australia,
2008, pp. 617–620.
16. Vlasenko B., Schuller B., Wendemuth A., Rigoll G. Frame vs. turn-level: emotion recognition from speech
considering static and dynamic processing. Affective Computing and Intelligent Interaction, 2007, vol. 4738
LNCS, pp. 139–147.
17. Vondra M., Vich R. Recognition of emotions in german speech using gaussian mixture models. Multimodal
Signals: Cognitive and Algorithmic Issues, 2009, vol. 5398 LNAI, pp. 256–263. doi: 10.1007/978-3-642-
00525-1_26
18. Ye C., Liu J., Chen C., Song M., Bu J. Speech emotion classification on a riemannian manifold. Advances in
Multimedia Information Processing – PCM 2008, 2008, vol. 5353 LNCS, pp. 61–69. doi: 10.1007/978-3-
540-89796-5_7
19. Gonen M., Alpaydin E. Multiple kernel learning algorithms. Journal of Machine Learning Research, 2011,
vol. 12, pp. 2211–2268.
20. Bitouk D., Verma R., Nenkova A. Class-level spectral features for emotion recognition. Speech Communication,
2010, vol. 52, no. 7–8, pp. 613–625. doi: 10.1016/j.specom.2010.02.010
21. Yang N., Muraleedharan R., Kohl J., Demirkol I., Heinzelman W., Sturge-Apple M. Speech-based emotion
classification using multiclass SVM with hybrid kernel and thresholding fusion. Proc. 4th IEEE Workshop on
Spoken Language Technology, SLT 2012. Miami, Florida, 2012, art. 6424267, pp. 455–460. doi:
10.1109/SLT.2012.6424267
22. Javidi M.M., Roshan E.F. Speech emotion recognition by using combinations of C5.0, neural network (NN),
and support vectors machines (SVM) classification methods. Journal of Mathematics and Computer Science,
2013, vol. 6, no. 3, pp. 191–200.
23. Anagnostopoulos T., Skourlas C. Ensemble majority voting classifier for speech emotion recognition and
prediction. Journal of Systems and Information Technology, 2014, vol. 16, no. 3, pp. 222–232. doi:
10.1108/JSIT-01-2014-0009
24. Ekman P. An argument for basic emotions. Cognition and Emotion, 1992, pp. 169–200.
25. Douglas-Cowie E., Cowie R., Sneddon I., Cox C., Lowry O., McRorie M., Martin J.-C., Devillers L.,
Abrilian S., Batliner A., Amir N., Karpouzis K. The HUMAINE database: addressing the collection and annotation
of naturalistic and induced emotional data. Proc. 2nd International Conference on Affective Computing
and Intelligent Interaction, ASCII 2007. Lisbon, Portugal, 2007, vol. 4738 LNCS, pp. 488–500.
26. Jury E.I. Theory and Application of the Z-Transform Method. Malabar, Krieger Pub Co, 1973, 330 p.
27. Friedman J., Hastie T., Tibshirani R. The Elements of Statistical Learning. NY, Springer, 2001, 524 p.
28. Alpaydin E. Introduction to Machine Learning. 2nd ed. Cambridge, MIT Press, 2010, 581 p.
29. Basu S., Dasgupta A. The mean, median, and mode of unimodal distributions: a characterization. Theory of
Probability and its Applications, 1997, vol. 41, no. 2, pp. 210–223. doi: 10.1137/S0040585X97975447
30. Seymour G. Predictive Inference. NY, Chapman and Hall, 1993, 240 p.
31. Hall M., Frank E., Holmes G., Pfahringer B., Reutemann P., Witten I.H. The WEKA data mining software:
an update. SIGKDD Explorations, 2009, vol. 11, no. 1, pp. 10–18. doi: 10.1145/1656274.1656278
32. Stehman S.V. Selecting and interpreting measures of thematic classification accuracy. Remote Sensing of
Environment, 1997, vol. 62, no. 1, pp. 77–89. doi: 10.1016/S0034-4257(97)00083-7
33. Vapnik V.N. The Nature of Statistical Learning Theory. 2nd ed. NY, Springer, 2000, 314 p.