Меню
Публикации
2025
2024
2023
2022
2021
2020
2019
2018
2017
2016
2015
2014
2013
2012
2011
2010
2009
2008
2007
2006
2005
2004
2003
2002
2001
Главный редактор
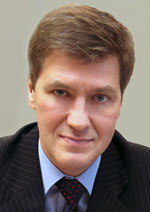
НИКИФОРОВ
Владимир Олегович
д.т.н., профессор
Партнеры
doi: 10.17586/2226-1494-2019-19-3-508-515
УДК 004.021:004.852:004.832.23
АВТОМАТИЧЕСКАЯ НАСТРОЙКА ГИПЕРПАРАМЕТРОВ АЛГОРИТМОВ КЛАСТЕРИЗАЦИИ С ПОМОЩЬЮ ОБУЧЕНИЯ С ПОДКРЕПЛЕНИЕМ
Читать статью полностью

Ссылка для цитирования:
Аннотация
Муравьёв С.Б., Ефимова В.А., Шаламов В.В., Фильченков А.А., Сметанников И.Б. Автоматическая настройка гиперпараметров алгоритмов кластеризации с помощью обучения с подкреплением // Научно-технический вестник информационных технологий, механики и оптики. 2019. Т. 19. № 3. С. 508–515. doi: 10.17586/2226-1494-2019-19-3-508-515
Аннотация
Предмет исследования. Исследованы алгоритмы выбора и настройки модели алгоритма в задачах кластеризации, применяемые в машинном обучении. Подробно рассмотрен метод выбора модели, показана необходимость поиска компромисса между исследованием и эксплуатацией, который производится с помощью сведения задачи к задаче о многоруком бандите. Метод. В работе предложен алгоритм одновременного выбора модели и настройки ее гиперпараметров на основе сведения к задаче о многоруком бандите. Предложены вариации алгоритма, использующие различные способы решения задачи о многоруком бандите, Softmax и UCB1, кроме того, награда определялась разными способами. Основные результаты. Проведенные эксперименты на реальных наборах данных из репозитория UCI позволили подтвердить, что предложенный алгоритм в целом за фиксированное время достигает существенно лучших результатов, чем метод полного перебора, а также позволили определить наиболее успешную вариацию предложенного алгоритма. Практическая значимость. Предложенный алгоритм может быть использован для выбора и настройки модели алгоритма кластеризации, в нем может использоваться любой алгоритм оптимизации гиперпараметров. Соответственно он может быть применен в широком спектре задач кластеризации, например, в биологии, психологии и при обработке изображений.
Ключевые слова: машинное обучение, кластеризация, настройка гиперпараметров, обучение с подкреплением, многорукий бандит
Благодарности. Работа выполнена при финансовой поддержке Правительства Российской Федерации, субсидия 08-08 и РФФИ, грант 16-37-60115-мол_а_дк.
Список литературы
Благодарности. Работа выполнена при финансовой поддержке Правительства Российской Федерации, субсидия 08-08 и РФФИ, грант 16-37-60115-мол_а_дк.
Список литературы
1. Halkidi M., Batistakis Y., Vazirgiannis M. On clustering validation techniques // Journal of Intelligent Information Systems. 2001. V. 17. N 2–3. P. 107–145. doi: 10.1023/a:1012801612483
2. Jain A.K., Murty M.N., Flynn P.J. Data clustering: a review // ACM Computing Surveys. 1999. V. 31. N 3. P. 264–323. doi: 10.1145/331499.331504
3. Mirkin B. Clustering for Data Mining: a Data Recovery Approach. CRC Press, 2005. 296 p. doi: 10.1201/9781420034912
4. Schlee D., Sneath P.H., Sokal R.R., Freman W.H. Numerical taxonomy. The principles and practice of numerical classification // Systematic Zoology. 1975. V. 24. N 2. P. 263–268. doi: 10.2307/2412767
5. Holzinger K.J., Harman H.H. Factor Analysis: A Synthesis of Factorial Methods. Chicago: University of Chicago Press, 1941. 417 p.
6. Chou C.H., Su M.C., Lai E. A new cluster validity measure and its application to image compression // Pattern Analysis and Applications. 2004. V. 7. N 2. P. 205–220. doi: 10.1007/s10044-004-0218-1
7. Luo M., Wang L.N., Zhang H.G. An unsupervised clustering- based intrusion detection method // Acta Electronica Sinica. 2003. V. 31. N 11. P. 1713–1716.
8. Von Luxburg U., Williamson R.C., Guyon I. Clustering: science or art // Proc. ICML Workshop on Unsupervised and Transfer Learning. Bellevue, USA, 2012. V. 27. P. 65–79.
9. Aggarwal C.C., Reddy C.K. Data Clustering: Algorithms and Applications. CRC press, 2013. 674 p. doi: 10.1201/b15410
10. Fraley C., Raftery A.E. How many clusters? Which clustering method? Answers via model-based cluster analysis // The Computer Journal. 1998. V. 41. N 8. P. 578–588. doi 10.1093/comjnl/41.8.578
11. Hennig C. What are the true clusters? // Pattern Recognition Letters. 2015. V. 64. P. 53–62. doi: 10.1016/j.patrec.2015.04.009
12. Pal N.R., Biswas J. Cluster validation using graph theoretic concepts // Pattern Recognition. 1997. V. 30. N 6. P. 847–857. doi: 10.1016/s0031-3203(96)00127-6
13. Ferrari D.G., De Castro L.N. Clustering algorithm selection by meta-learning systems: A new distance-based problem characterization and ranking combination methods // Information Sciences. 2015. V. 301. P. 181–194. doi: 10.1016/j.ins.2014.12.044
14. Tibshirani R., Walther G., Hastie T. Estimating the number of clusters in a data set via the gap statistic // Journal of the Royal Statistical Society: Series B (Statistical Methodology). 2001. V. 63. N 2. P. 411–423. doi: 10.1111/1467-9868.00293
15. Sugar C.A., James G.M. Finding the number of clusters in a dataset // Journal of the American Statistical Association. 2003. V. 98. N 463. P. 750–763. doi: 10.1198/016214503000000666
16. Pelleg D., Moore A.W. X-means: Extending k-means with efficient estimation of the number of clusters // Proc. 17th Int. Conf. on Machine Learning. Stanford, USA, 2000. Part 1. P. 727– 734.
17. Thornton C., Hutter F., Hoos H.H., Leyton-Brown K. Auto-WEKA: Combined selection and hyperparameter optimization of classification algorithms // Proc. 19th ACM SIGKDD Int. Conf. on Knowledge Discovery and Data Mining. Chicago, USA, 2012. P. 847–855. doi: 10.1145/2487575.2487629
18. Olson R.S., Bartley N., Urbanowicz R.J., Moore J.H. Evaluation of a tree-based pipeline optimization tool for automating data science // Proc. Genetic and Evolutionary Computation Conference. Denver, USA, 2016. P. 485–492. doi: 10.1145/2908812.2908918
19. Feurer M., Klein A., Eggensperger K., Springenberg J., Blum M., Hutter F. Efficient and robust automated machine learning // Advances in Neural Information Processing Systems. 2015. V. 2. P. 2962–2970.
20. Ефимова В.А., Фильченков А.А., Шалыто А.А. Применение обучения с подкреплением для одновременного выбора модели алгоритма классификации и ее структурных параметров // Машинное обучение и анализ данных. 2016. № 2. С. 244–254.
21. Kotthoff L., Thornton C., Hoos H.H., Hutter F., Leyton-Brown K. Auto-WEKA 2.0: Automatic model selection and hyperparameter optimization in WEKA // The Journal of Machine Learning Research. 2017. V. 18. N 1. P. 826–830.
22. Sutton R.S., Barto A.G. Introduction to Reinforcement Learning. Cambridge: MIT press, 1998. 322 p.
23. Hutter F., Hoos H.H., Leyton-Brown K. Sequential model-based optimization for general algorithm configuration // Lecture Notes in Computer Science. 2011. V. 6683. P. 507–523. doi: 10.1007/978-3-642-25566-3_40
24. Arbelaitz O., Gurrutxaga I., Muguerza J., Perez J. M., Perona I. An extensive comparative study of cluster validity indices // Pattern Recognition. 2003. V. 46. N 1. P. 243–256. doi: 10.1016/j.patcog.2012.07.021
25. Filchenkov A., Muravyov S., Parfenov V. Towards cluster validity index evaluation and selection // Proc. IEEE Artificial Intelligence and Natural Language Conference. St. Petersburg, Russia, 2016. P. 1–8.
2. Jain A.K., Murty M.N., Flynn P.J. Data clustering: a review // ACM Computing Surveys. 1999. V. 31. N 3. P. 264–323. doi: 10.1145/331499.331504
3. Mirkin B. Clustering for Data Mining: a Data Recovery Approach. CRC Press, 2005. 296 p. doi: 10.1201/9781420034912
4. Schlee D., Sneath P.H., Sokal R.R., Freman W.H. Numerical taxonomy. The principles and practice of numerical classification // Systematic Zoology. 1975. V. 24. N 2. P. 263–268. doi: 10.2307/2412767
5. Holzinger K.J., Harman H.H. Factor Analysis: A Synthesis of Factorial Methods. Chicago: University of Chicago Press, 1941. 417 p.
6. Chou C.H., Su M.C., Lai E. A new cluster validity measure and its application to image compression // Pattern Analysis and Applications. 2004. V. 7. N 2. P. 205–220. doi: 10.1007/s10044-004-0218-1
7. Luo M., Wang L.N., Zhang H.G. An unsupervised clustering- based intrusion detection method // Acta Electronica Sinica. 2003. V. 31. N 11. P. 1713–1716.
8. Von Luxburg U., Williamson R.C., Guyon I. Clustering: science or art // Proc. ICML Workshop on Unsupervised and Transfer Learning. Bellevue, USA, 2012. V. 27. P. 65–79.
9. Aggarwal C.C., Reddy C.K. Data Clustering: Algorithms and Applications. CRC press, 2013. 674 p. doi: 10.1201/b15410
10. Fraley C., Raftery A.E. How many clusters? Which clustering method? Answers via model-based cluster analysis // The Computer Journal. 1998. V. 41. N 8. P. 578–588. doi 10.1093/comjnl/41.8.578
11. Hennig C. What are the true clusters? // Pattern Recognition Letters. 2015. V. 64. P. 53–62. doi: 10.1016/j.patrec.2015.04.009
12. Pal N.R., Biswas J. Cluster validation using graph theoretic concepts // Pattern Recognition. 1997. V. 30. N 6. P. 847–857. doi: 10.1016/s0031-3203(96)00127-6
13. Ferrari D.G., De Castro L.N. Clustering algorithm selection by meta-learning systems: A new distance-based problem characterization and ranking combination methods // Information Sciences. 2015. V. 301. P. 181–194. doi: 10.1016/j.ins.2014.12.044
14. Tibshirani R., Walther G., Hastie T. Estimating the number of clusters in a data set via the gap statistic // Journal of the Royal Statistical Society: Series B (Statistical Methodology). 2001. V. 63. N 2. P. 411–423. doi: 10.1111/1467-9868.00293
15. Sugar C.A., James G.M. Finding the number of clusters in a dataset // Journal of the American Statistical Association. 2003. V. 98. N 463. P. 750–763. doi: 10.1198/016214503000000666
16. Pelleg D., Moore A.W. X-means: Extending k-means with efficient estimation of the number of clusters // Proc. 17th Int. Conf. on Machine Learning. Stanford, USA, 2000. Part 1. P. 727– 734.
17. Thornton C., Hutter F., Hoos H.H., Leyton-Brown K. Auto-WEKA: Combined selection and hyperparameter optimization of classification algorithms // Proc. 19th ACM SIGKDD Int. Conf. on Knowledge Discovery and Data Mining. Chicago, USA, 2012. P. 847–855. doi: 10.1145/2487575.2487629
18. Olson R.S., Bartley N., Urbanowicz R.J., Moore J.H. Evaluation of a tree-based pipeline optimization tool for automating data science // Proc. Genetic and Evolutionary Computation Conference. Denver, USA, 2016. P. 485–492. doi: 10.1145/2908812.2908918
19. Feurer M., Klein A., Eggensperger K., Springenberg J., Blum M., Hutter F. Efficient and robust automated machine learning // Advances in Neural Information Processing Systems. 2015. V. 2. P. 2962–2970.
20. Ефимова В.А., Фильченков А.А., Шалыто А.А. Применение обучения с подкреплением для одновременного выбора модели алгоритма классификации и ее структурных параметров // Машинное обучение и анализ данных. 2016. № 2. С. 244–254.
21. Kotthoff L., Thornton C., Hoos H.H., Hutter F., Leyton-Brown K. Auto-WEKA 2.0: Automatic model selection and hyperparameter optimization in WEKA // The Journal of Machine Learning Research. 2017. V. 18. N 1. P. 826–830.
22. Sutton R.S., Barto A.G. Introduction to Reinforcement Learning. Cambridge: MIT press, 1998. 322 p.
23. Hutter F., Hoos H.H., Leyton-Brown K. Sequential model-based optimization for general algorithm configuration // Lecture Notes in Computer Science. 2011. V. 6683. P. 507–523. doi: 10.1007/978-3-642-25566-3_40
24. Arbelaitz O., Gurrutxaga I., Muguerza J., Perez J. M., Perona I. An extensive comparative study of cluster validity indices // Pattern Recognition. 2003. V. 46. N 1. P. 243–256. doi: 10.1016/j.patcog.2012.07.021
25. Filchenkov A., Muravyov S., Parfenov V. Towards cluster validity index evaluation and selection // Proc. IEEE Artificial Intelligence and Natural Language Conference. St. Petersburg, Russia, 2016. P. 1–8.