Меню
Публикации
2025
2024
2023
2022
2021
2020
2019
2018
2017
2016
2015
2014
2013
2012
2011
2010
2009
2008
2007
2006
2005
2004
2003
2002
2001
Главный редактор
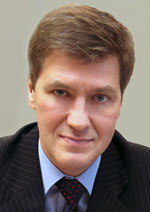
НИКИФОРОВ
Владимир Олегович
д.т.н., профессор
Партнеры
doi: 10.17586/2226-1494-2022-22-2-308-316
УДК 004.021
Создание корпуса иракского арабского диалекта в Твиттере для анализа настроений
Читать статью полностью

Язык статьи - английский
Ссылка для цитирования:
Аннотация
Ссылка для цитирования:
Хассун Аль-Джавад М.М., Альхарби Х., Альмухтар А.Ф., Алнавас А.А. Создание корпуса иракского арабского диалекта в Твиттере для анализа настроений // Научно-технический вестник информационных технологий, механики и оптики. 2022. Т. 22, № 2. С. 308–316 (на англ. яз.). doi:10.17586/2226-1494-2022-22-2-308-316
Аннотация
За последние годы количество пользователей Twitter в Ираке значительно увеличилось. Крупные события и политическая обстановка в стране оказывают значительное влияние на информацию в Twitter и затрагивают сообщения иракских пользователей. Создание корпуса иракских арабских диалектов Corpus of Iraqi Arabic Dialect (CIAD) имеет решающее значение для анализа настроений и изучения такой информации. Представлено исследование CIAD, который был разработан и описан другими исследователями. Корпус проверен с использованием восьми различных комбинаций четырех подходов к выбору признаков и двух версий алгоритма метода опорных векторов. Рассчитаны различные показатели эффективности. Полученная точность составила 78 %, что указывает на многообещающее
Ключевые слова: анализ настроений, интеллектуальный анализ данных, метод опорных векторов, поведение пользователей, интеллектуальный анализ социальных сетей
Список литературы
Список литературы
-
Stone M.L. et al. BIg data for Media // Reuters Institute for the Study of Journalism. 2014. November.
-
Badaro G., Baly R., Hajj H., El-Hajj W., Shaban K.B., Habash N., Al-Sallab A., Hamdi A.A survey of opinion mining in Arabic: A comprehensive system perspective covering challenges and advances in tools, resources, models, applications, and visualizations // ACM Transactions on Asian and Low-Resource Language Information Processing. 2019. V. 18.N 3.P. 27. https://doi.org/10.1145/3295662
-
Zaidan O.F., Callison-Burch C. Arabic dialect identification // Computational Linguistics.2014. V. 40.N 1. P. 171–202.https://doi.org/10.1162/COLI_a_00169
-
Habash N.Y. Introduction to Arabic natural language processing // Synthesis Lectures on Human Language Technologies. 2010. V. 3.N 1.https://doi.org/10.2200/S00277ED1V01Y201008HLT010
-
Alnawas A., AriciN. The corpus based approach to sentiment analysis in modern standard Arabic and Arabic dialects: A literature review // Journal of Polytechnic-Politeknik Dergisi. 2018.V. 21. N 2. P. 461–470. https://doi.org/10.2339/politeknik.403975
-
Alshutayri A., Atwell E. Classifying Arabic dialect text in the Social Media Arabic Dialect Corpus (SMADC) // Proc.of the 3rd Workshop on Arabic Corpus Linguistics. 2019. P. 51–59.
-
Abo M.E.M., Raj R.G., Qazi A. A review on Arabic sentiment analysis: State-of-The-Art, taxonomy and open research challenges // IEEE Access. 2019. V. 7.P. 162008–162024. https://doi.org/10.1109/ACCESS.2019.2951530
-
Kumar A., Jaiswal A. Systematic literature review of sentiment analysis on Twitter using soft computing techniques // Concurrency and Computation: Practice and Experience. 2020. V. 32.N 1.P. e5107. https://doi.org/10.1002/cpe.5107
-
Cieliebak M., Deriu J., Egger D., Uzdilli F.A Twitter corpus and benchmark resources for German sentiment analysis// Proc. of the 5th International Workshop on Natural Language Processing for Social Media (SocialNLP).2017.P. 45–51. https://doi.org/10.18653/v1/W17-1106
-
Nabil M., Aly M., Atiya A.F. ASTD: Arabic sentiment tweets dataset // Proc. of the Conference on Empirical Methods in Natural Language Processing (EMNLP). 2015.P. 2515–2519. https://doi.org/10.18653/v1/D15-1299
-
Alahmary R.M., Al-Dossari H.Z., Emam A.Z. Sentiment analysis of Saudi dialect using deep learning techniques // Proc. of the 18th International Conference on Electronics, Information, and Communication (ICEIC). 2019.P. 8706408. https://doi.org/10.23919/ELINFOCOM.2019.8706408
-
Alnawas A., Arici N. Sentiment analysis of Iraqi Arabic dialect on Facebook based on distributed representations of documents // ACM Transactions on Asian and Low-Resource Language Information Processing. 2019. V. 18.N 3.P. a20. https://doi.org/10.1145/3278605
-
Kwaik K.A., Saad M., Chatzikyriakidis S., Dobnik S.Shami: A corpus of levantine Arabic dialects // Proc. of the 11th International Conference on Language Resources and Evaluation. (LREC-2018). 2019.P. 3645–3652.
-
Oussous A., Lahcen A.A., Belfkih S. Impact of text pre-processing and ensemble learning on Arabic sentiment analysis // ACM International Conference Proceeding Series. 2019. V. Part F148154.P. 65. https://doi.org/10.1145/3320326.3320399
-
El Abdouli A., Hassouni L., Anoun H. Sentiment analysis of moroccan tweets using naive bayes algorithm // International Journal of Computer Science and Information Security. 2017. V. 15.N 12.P. 191–200.
-
Bouazizi M., Ohtsuki T. Sentiment analysis: From binary to multi-class classification: A pattern-based approach for multi-class sentiment analysis in Twitter // Proc. of the IEEE International Conference on Communications(ICC). 2016.P. 7511392. https://doi.org/10.1109/ICC.2016.7511392
-
Altamimi M., Alruwaili O., Teahan W.J. BTAC: A twitter corpus for Arabic dialect identification // Proc. of the 6th Conference on Computer-Mediated Communication (CMC) and Social Media Corpora (CMC-corpora 2018). 2018. P. 5.
-
Al-Yasiri E.K., Al-Azawei A. Improving Arabic sentiment analysis on social media: A comparative study on applying different pre-processing techniques // Compusoft. 2019. V. 8.N 6.P. 3150–3157.
-
Platt J.C. Sequential Minimal Optimization: A fast algorithm for training support vector machines // CiteSeerX. 1998. V. 10.N 1.43. P. 4376.