Меню
Публикации
2025
2024
2023
2022
2021
2020
2019
2018
2017
2016
2015
2014
2013
2012
2011
2010
2009
2008
2007
2006
2005
2004
2003
2002
2001
Главный редактор
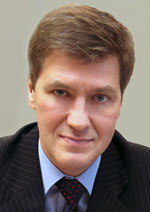
НИКИФОРОВ
Владимир Олегович
д.т.н., профессор
Партнеры
doi: 10.17586/2226-1494-2022-22-3-415-432
УДК 004.932.2
Методы аудиовизуального распознавания людей в масках
Читать статью полностью

Язык статьи - русский
Ссылка для цитирования:
Аннотация
Ссылка для цитирования:
Косулин К.Э., Карпов А.А. Методы аудиовизуального распознавания людей в масках // Научно-технический вестник информационных технологий, механики и оптики. 2022. Т. 22, № 3. С. 415–432. doi: 10.17586/2226-1494-2022-22-3-415-432
Аннотация
Предмет исследования. В современном мире очень распространены случаи ношения людьми различных масок, респираторов и одежды на лице. Начавшаяся в 2019 году пандемия новой коронавирусной инфекции существенно увеличила применимость масок в общественных местах. Наиболее эффективными способами бесконтактного распознания личности являются методы идентификации и верификации человека по изображению лица и по записи голоса. Автоматические системы распознавания личности столкнулись с новыми проблемами из-за перекрытия большей части лица маской. Наличие данной проблемы определяет актуальность исследований в области распознания лиц в масках. Предмет исследования работы — системы и корпусы данных для распознавания личности людей в масках. Метод. Рассмотрены и проанализированы основные современные подходы и методы распознавания личности людей в масках, использующие изображения лица, записи голоса человека и аудиовизуальные методы. Приведен сравнительный анализ существующих корпусов данных, содержащих изображения и записи голосов людей, необходимые для создания систем распознавания личности. Основные результаты. Результаты анализа показали, что среди методов, использующих изображения лиц, наиболее эффективными являются методы, построенные на основе сверточных нейронных сетей, которые применяют область маски для извлечения признаков о геометрии лица. Популярные методы на основе x-векторов показали незначительное падение эффективности, что позволяет сделать вывод об их применимости в задачах распознавания личности говорящего в маске. Практическая значимость. На основании полученных выводов сформулированы требования к перспективным системам распознавания личности и определены актуальные направления для дальнейших исследований в данной области.
Ключевые слова: распознавание личности, лицевая биометрия, голосовая биометрия, медицинские маски, средства индивидуальной защиты, аудиовизуальные характеристики, объединение информации
Благодарности. Работа выполнена при поддержке фонда РФФИ (проект № 20-04-60529), Совета по грантам Президента РФ (грант № НШ-17.2022.1.6), а также в рамках бюджетной темы (№ 0073-2019-0005).
Список литературы
Благодарности. Работа выполнена при поддержке фонда РФФИ (проект № 20-04-60529), Совета по грантам Президента РФ (грант № НШ-17.2022.1.6), а также в рамках бюджетной темы (№ 0073-2019-0005).
Список литературы
1. Chernenkova A. Facial recognition technology in Russia: do the citizens of Russia accept it? / University of Twente. BMS Faculty Department of Communication Science University of Twente. 2021. 78 p.
2. Кухарев Г.А., Матвеев Ю.Н., Форчманьски П. Поиск людей по фотороботам: методы, системы и практические решения // Научно-технический вестник информационных технологий, механики и оптики. 2015. Т. 15. № 4. С. 640–653. https://doi.org/10.17586/26-1494-2015-15-224-640-653
3. Grother P., Ngan M. Face recognition vendor test (FRVT): NIST Interagency Report 8009 / US Department of Commerce, National Institute of Standards and Technology. 2014. https://doi.org/10.6028/NIST.IR.8009
4. Двойникова А.А., Маркитантов М.В., Рюмина Е.В., Рюмин Д.А., Карпов А.А. Аналитический обзор аудиовизуальных систем для определения средств индивидуальной защиты на лице человека // Информатика и автоматизация. 2021. Т. 20. № 5. С. 1116–1152. https://doi.org/10.15622/20.5.5
5. Wang Z., Wang G., Huang B., Xiong Z., Hong Q., Wu H., Yi P., Jiang K., Wang N., Pei Y., Chen H., Miao Y., Huang Z., Liang J. Masked face recognition dataset and application // arXiv. 2020. arXiv:2003.09093.. https://doi.org/10.48550/arXiv.2003.09093
6. Geng M., Peng P., Huang Y., Tian Y. Masked face recognition with generative data augmentation and domain constrained ranking // Proc. of the 28th ACM International Conference on Multimedia. 2020. P. 2246–2254. https://doi.org/10.1145/3394171.3413723
7. Din N.U., Javed K., Bae S., Yi J. A novel GAN-based network for unmasking of masked face // IEEE Access. 2020. V. 8. P. 44276–44287. https://doi.org/10.1109/ACCESS.2020.29773868. Din N.U., Javed K., Bae S., Yi J. Effective removal of user-selected foreground object from facial images using a novel GAN-based network // IEEE Access. 2020. V. 8. P. 109648–109661. https://doi.org/10.1109/ACCESS.2020.3001649
9. Deng J., Guo J., Xue N., Zafeiriou S. ArcFace: Additive angular margin loss for deep face recognition // Proc. of the 32nd IEEE/CVF Conference on Computer Vision and Paern Recognition (CVPR). 2019. P. 4685–4694. https://doi.org/10.1109/CVPR.2019.00482
10. Boutros F., Damer N., Kirchbuchner F., Kuijper A. Self-restrained triplet loss for accurate masked face recognition // Pattern Recognition. 2022. V. 124. P. 108473. https://doi.org/10.1016/j.patcog.2021.108473
11. Anwar A., Raychowdhury A. Masked face recognition for secure authentication // arXiv. 2020. arXiv:2008.11104. https://doi.org/10.48550/arXiv.2008.11104
12. Damer N., Grebe J.H., Chen C., Boutros F., Kirchbuchner F., Kuijper A. The effect of wearing a mask on face recognition performance: an exploratory study // Proc. of the 19th International Conference of the Biometrics Special Interest Group (BIOSIG). 2020. P. 9210999.
13. Huang G.B., Ramesh M., Berg T., Learned-Miller E. Labeled faces in the wild: A database for studying face recognition in unconstrained environments // Workshop on Faces in'RealLife'Images: Detection, Alignment, and Recognition. 2008.
14. Ding F., Peng P., Huang Y., Geng M., Tian Y. Masked face recognition with latent part detection // Proc. of the 28th ACM international Conference on Multimedia. 2020. P. 2281–2289. https://doi.org/10.1145/3394171.3413731
15. Li S., Yi D., Lei Z., Liao S. The CASIA NIR-VIS 2.0 face database // Proc. of the IEEE Conference on Computer Vision and Pattern Recognition Workshops. 2013. P. 348–353. https://doi.org/10.1109/CVPRW.2013.59
16. Deng H., Feng Z., Qian G., Lv X., Li H., Li G. MFCosface: a masked-face recognition algorithm based on large margin cosine loss // Applied Sciences. 2021. V. 11. N 16. P. 7310. https://doi.org/10.3390/app11167310
17. Yi D., Lei Z., Liao S., Li S.Z. Learning face representation from scratch // arXiv. 2014. arXiv:1411.7923. https://doi.org/10.48550/arXiv.1411.7923
18. Hong J.H., Kim H., Kim M., Nam G.P., Cho J., Ko H.-S., Kim I.-J. A 3D model-based approach for fitting masks to faces in the wild // Proc. of the IEEE International Conference on Image Processing (ICIP). 2021. P. 235–239. https://doi.org/10.1109/ICIP42928.2021.9506069
19. Hariri W. Efficient masked face recognition method during the COVID-19 pandemic // Signal, Image and Video Processing. 2022. V. 16. N 3. P. 605–612. https://doi.org/10.1007/s11760-021-02050-w
20. Maharani D.A., MacHbub C., Rusmin P.H., Yulianti L. Improving the capability of real-time face masked recognition using cosine distance // Proc. of the 6th International Conference on Interactive Digital Media (ICIDM). 2020. P. 9339677. https://doi.org/10.1109/ICIDM51048.2020.9339677
21. Montero D., Nieto M., Leskovsky P., Aginako N. Boosting masked face recognition with multi-task arcface // arXiv. 2021. arXiv:2104.09874. https://doi.org/10.48550/arXiv.2104.09874
22. Golwalkar R., Mehendale N. Masked Face Recognition Using Deep Metric Learning and FaceMaskNet21 // SSRN Electronic Journal. 2020. P. 3731223. http://dx.doi.org/10.2139/ssrn.3731223
23. Li C., Ge S., Zhang D., Li J. Look through masks: Towards masked face recognition with de-occlusion distillation // Proc. of the 28th ACM International Conference on Multimedia. 2020. P. 3016–3024. https://doi.org/10.1145/3394171.3413960
24. Hong Q., Wang Z., He Z., Wang N., Tian X., Lu T. Masked face recognition with identification association // Proc. of the IEEE 32nd International Conference on Tools with Artificial Intelligence (ICTAI). 2020. P. 731–735. https://doi.org/10.1109/ICTAI50040.2020.00116
25. Mandal B., Okeukwu A., Theis Y. Masked face recognition using ResNet-50 // arXiv. 2021. arXiv:2104.08997. https://doi.org/10.48550/arXiv.2104.08997
26. Du H., Shi H., Liu Y., Zeng D., Mei T. Towards NIR-VIS masked face recognition // IEEE Signal Processing Letters. 2021. V. 28. P. 768–772. https://doi.org/10.1109/LSP.2021.3071663
27. Wu G.L. Masked face recognition algorithm for a contactless distribution cabinet // Mathematical Problem in Engineering. 2021. V. 2021. P. 5591020. https://doi.org/10.1155/2021/5591020
28. Li Y., Guo K., Lu Y., Liu L. Cropping and attention based approach for masked face recognition // Applied Intelligence. 2021. V. 51. N 5. P. 3012–3025. https://doi.org/10.1007/s10489-020-02100-9
29. Gover A.R., Harper S.B., Langton L. Anti-Asian hate crime during the COVID-19 pandemic: Exploring the reproduction of inequality // American Journal of Criminal Justice. 2020. V. 45. N 4. P. 647–667. https://doi.org/10.1007/s12103-020-09545-1
30. Saeidi R., Niemi T., Karppelin H., Pohjalainen J., Kinnunen T., Alku P. Speaker recognition for speech under face cover // Proc. of the Interspeech. 2015. P. 1012–1016. https://doi.org/10.21437/Interspeech.2015-275
31. Zhang C., Tan T. Voice disguise and automatic speaker recognition // Forensic Science International. 2008. V. 175. N 2-3. P. 118–122. https://doi.org/10.1016/j.forsciint.2007.05.019
32. Fecher N. The "audio-visual face cover corpus": investigations into audio-visual speech and speaker recognition when the speaker's face is occluded by facewear // Proc. of the 13th Annual Conference of the International Speech Communication Association (INTERSPEECH). 2012. P. 2250–2253. https://doi.org/10.21437/Interspeech.2012-133
33. Iszatt T., Malkoc E., Kelly F., Alexander A. Exploring the impact of face coverings on x-vector speaker recognition using VOCALISE // Poc. of the Conference: International Association of Forensic Phonetics and Acoustics. 2021.
34. Schuller B.W., Batliner A., Bergler C., Messner E.-M., Hamilton A., Amiriparian S., Baird A., Rizos G., Schmitt M., Stappen L., Baumeister H., MacIntyre D.A., Hantke S. The interspeech 2020 computational paralinguistics challenge: Elderly emotion, breathing & masks // Proc. of the Interspeech. 2020. P. 2042–2046. https://doi.org/10.21437/Interspeech.2020-32
35. Montacié C., Caraty M. J. Phonetic, frame clustering and intelligibility analyses for the INTERSPEECH 2020 ComParE Challenge // Proc. of the 21st Annual Conference of the International Speech Communication Association (INTERSPEECH). 2020. P. 2062–2066. https://doi.org/10.21437/Interspeech.2020-2243
36. Рюмина Е.В., Рюмин Д.А., Маркитантов М.В., Карпов А.А. Метод генерации обучающих данных для компьютерной системы обнаружения защитных масок на лицах людей // Компьютерная оптика. 2022. Т. 46. в печати.
37. Przybocki M.A., Martin A.F., Le A.N. NIST speaker recognition evaluations utilizing the Mixer corpora–2004, 2005, 2006 // IEEE Transactions on Audio, Speech, and Language Processing. 2007. V. 15. N 7. P. 1951–1959. https://doi.org/10.1109/TASL.2007.902489
38. Alam M.R., Bennamoun M., Togneri R., Sohel F. An efficient reliability estimation technique for audio-visual person identification // Proc. of the IEEE 8th Conference on Industrial Electronics and Applications (ICIEA). 2013. P. 1631–1635. https://doi.org/10.1109/ICIEA.2013.6566630
39. Zhao X., Evans N., Dugelay J.-L. Multi-view semi-supervised discriminant analysis: A new approach to audio-visual person recognition // Proc. of the 20th European Signal Processing Conference (EUSIPCO). 2012. P. 31–35.
40. Nishino T., Kajikawa Y., Muneyasu M. Multimodal person authentication system using features of utterance // Proc. of the 20th International Symposium on Intelligent Signal Processing and Communications Systems (ISPACS). 2012. P. 43–47. https://doi.org/10.1109/ISPACS.2012.6473450
41. Alam M.R., Bennamoun M., Togneri R., Sohel F. A joint deep Boltzmann machine (jDBM) model for person identification using mobile phone data // IEEE Transactions on Multimedia. 2017. V. 19. N 2. P. 317–326. https://doi.org/10.1109/TMM.2016.2615524
42. Gofman M., Sandico N., Mitra S., Suo E., Muhi S., Vu T. Multimodal biometrics via discriminant correlation analysis on mobile devices // Proc. of the International Conference on Security and Management (SAM). The Steering Committee of The World Congress in Computer Science, Computer Engineering and Applied Computing (WorldComp). 2018. P. 174–181.
43. Shenoy R.V. Hidden Markov Models for Analysis of Multimodal Biomedical Images: A dissertation submitted in partial satisfaction of the requirements for the degree Doctor of Philosophy in Electrical and Computer Engineering / University of California, Santa Barbara, 2016. 99 p.
44. Chen Y., Yang J., Wang C., Liu N. Multimodal biometrics recognition based on local fusion visual features and variational Bayesian extreme learning machine // Expert Systems with Applications. 2016. V. 64. P. 93–103. https://doi.org/10.1016/j.eswa.2016.07.009
45. Garau M., Fraschini M., Didaci L., Marcialis G.L. Experimental results on multi-modal fusion of EEG-based personal verification algorithms // Proc. of the 9th International Conference on Biometrics (ICB). 2016. P. 7550080. https://doi.org/10.1109/ICB.2016.7550080
46. Shen L., Zheng N., Zheng S., Li W. Secure mobile services by face and speech based personal authentication // Proc. of the IEEE International Conference on Intelligent Computing and Intelligent Systems (ICIS). V. 3. 2010. P. 97–100. https://doi.org/10.1109/ICICISYS.2010.5658534
47. Irfan B., Ortiz M.-G., Lyubova N., Belpaeme T. Multi-modal open-set person identification in HRI // Proc. of the 2018 ACM/IEEE International Conference on Human-Robot Interaction Social Robots in the Wild workshop. 2018.
48. Antipov G., Gengembre N., Le Blouch O., Le Lan G. Automatic quality assessment for audio-visual verification systems. The LOVe submission to NIST SRE challenge 2019 // Proc. of the 21st Annual Conference of the International Speech Communication Association (INTERSPEECH). 2020. P. 2237–2241. https://doi.org/10.21437/Interspeech.2020-1434
49. Estival D., Cassidy S., Cox F., Burnham D. AusTalk: an audio-visual corpus of Australian English // Proc. of the Ninth International Conference on Language Resources and Evaluation (LREC'14). 2014.
50. Khoury E., El Shafey L., McCool C., Günther M., Marcel S. Bi-modal biometric authentication on mobile phones in challenging conditions // Image and Vision Computing. 2014. V. 32. N 12. P. 1147–1160. https://doi.org/10.1016/j.imavis.2013.10.001
51. McCool C., Marcel S. MOBIO database for the ICPR 2010 face and speech competition: Idiap Communication Report. Idiap Research Institute, 2009.
52. Alam M.R., Togneri R., Sohel F., Bennamoun M., Naseem I. Linear regression-based classifier for audio visual person identification // Proc. of the 1st International Conference on Communications, Signal Processing, and their Applications (ICCSPA). 2013. P. 6487281. https://doi.org/10.1109/ICCSPA.2013.6487281
53. Tresadern P., Cootes T.F., Poh N., Matejka P., Hadid A., Lévy C., McCool C., Marcel S. Mobile biometrics: Combined face and voice verification for a mobile platform // IEEE Pervasive Computing. 2013. V. 12. N 1. P. 79–87. https://doi.org/10.1109/MPRV.2012.54
54. Islam R., Sobhan A. BPN based likelihood ratio score fusion for audio-visual speaker identification in response to noise // International Scholarly Research Notices. 2014. V. 2014. P. 737814. https://doi.org/10.1155/2014/737814
55. Primorac R., Togneri R., Bennamoun M., Sohel F. Audio-visual biometric recognition via joint sparse representations // Proc. of the 23rd International Conference on Pattern Recognition (ICPR). 2016. P. 3031–3035. https://doi.org/10.1109/ICPR.2016.7900099
56. Memon Q., AlKassim Z., AlHassan E., Omer M., Alsiddig M. Audio-visual biometric authentication for secured access into personal devices // Proc. of the 6th International Conference on Bioinformatics and Biomedical Science (ICBBS). 2017. P. 85–89. https://doi.org/10.1145/3121138.3121165
57. Gofman M.I., Mitra S., Cheng T.-H.K., Smith N.T. Multimodal biometrics for enhanced mobile device security // Communications of the ACM. 2016. V. 59. N 4. P. 58–65. https://doi.org/10.1145/2818990
58. Sadjadi S.O., Greenberg C., Singer E., Reynolds D., Mason L., Hernandez-Cordero J. The 2019 NIST speaker recognition evaluation CTS challenge // Proc. of the Speaker and Language Recognition Workshop (Odyssey 2020). 2020. P. 266–272. https://doi.org/10.21437/Odyssey.2020-38
59. Yu C., Huang L. Biometric recognition by using audio and visual feature fusion // Proc. of the 2012 International Conference on System Science and Engineering (ICSSE). 2012. P. 173178. https://doi.org/10.1109/ICSSE.2012.6257171