Меню
Публикации
2024
2023
2022
2021
2020
2019
2018
2017
2016
2015
2014
2013
2012
2011
2010
2009
2008
2007
2006
2005
2004
2003
2002
2001
Главный редактор
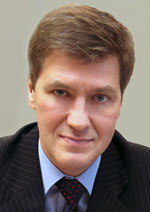
НИКИФОРОВ
Владимир Олегович
д.т.н., профессор
Партнеры
doi: 10.17586/2226-1494-2023-23-2-340-351
УДК 004.94
Выделение ролей в сетях общественного транспорта с атрибутами узлов: описание модели
Читать статью полностью

Язык статьи - английский
Ссылка для цитирования:
Аннотация
Ссылка для цитирования:
Лыткин Ю.В., Чунаев П.В., Градов Т.А., Бойцов А.А., Саитов И.А. Выделение ролей в сетях общественного транспорта с атрибутами узлов: описание модели // Научно-технический вестник информационных технологий, механики и оптики. 2023. Т. 23, № 2. С. 340–351 (на англ. яз.). doi: 10.17586/2226-1494-2023-23-2-340-351
Аннотация
Предмет исследования. Моделирование систем общественного транспорта с позиций теории сложных сетей имеет большое значение для повышения их эффективности и надежности. При этом важной задачей является анализ ролей узлов и взвешенных связей в сети, моделирующих группы остановок общественного транспорта и связывающие их маршруты. В настоящее время данная задача решена на основе только топологической и геопространственной информации о наличии маршрутов между остановками и их географическом положении. Такое ограничение приводит к проблеме неинтерпретируемости выделенных ролей. Для решения проблемы определения ролей в сетях в предложенной модели транспорта дополнительно учтена информация о социальной инфраструктуре вокруг остановок, а также осуществлено выделение совместно топологических, геопространственных и инфраструктурных ролей. Метод. Система общественного транспорта смоделирована с помощью специальной взвешенной сети — с атрибутами узлов. При этом узлы — непересекающиеся группы остановок, объединенные по геопространственному положению, атрибуты узлов — векторы, содержащие сведения о социальной инфраструктуре вокруг остановок, а взвешенные связи интегрируют информацию о расстоянии и количестве пересадок в маршрутах между остановками. Для идентификации модели достаточно использовать только открытые городские данные о системе общественного транспорта. Выделение ролей остановок выполнено путем кластеризации узлов сети в соответствии с их топологическими и атрибутивными признаками. Основные результаты. Предложена обобщенная модель системы общественного транспорта. Представлен новый подход решения задачи выделения ролей остановок, обеспечивающий интерпретируемость с топологической, геопространственной и инфраструктурной точек зрения. Модель идентифицирована на открытых данных Санкт-Петербурга об остановках подземного, троллейбусного и автобусного транспорта, а также организациях и предприятиях вокруг остановок. На основе данных найдены сбалансированные параметры объединения остановок, назначения весов связей и построения векторов атрибутов для последующего использования в задаче выделения ролей. Практическая значимость. Результаты исследования могут быть использованы для определения транспортных и инфраструктурных недостатков реальных систем общественного транспорта, которые следует учитывать для улучшения функционирования этих систем в будущем.
Ключевые слова: сеть с атрибутами узлов, сеть общественного транспорта, выделение ролей, классификация узлов сети, топология сети, социальная инфраструктура
Благодарности. Исследование выполнено за счет гранта Российского научного фонда (проект № 17-71-30029) при софинансировании ПАО «Банк Санкт-Петербург».
Список литературы
Благодарности. Исследование выполнено за счет гранта Российского научного фонда (проект № 17-71-30029) при софинансировании ПАО «Банк Санкт-Петербург».
Список литературы
-
Sen P., Dasgupta S., Chatterjee A., Sreeram P.A., Mukherjee G., Manna S.S. Small-world properties of the indian railway network // Physical Review E. 2003. V. 67. N 3. P. 036106. https://doi.org/10.1103/physreve.67.036106
-
Sienkiewicz J., Hołyst J. Statistical analysis of 22 public transport networks in Poland // Physical Review E. 2005. V. 72. N 4. P. 046127. https://doi.org/10.1103/physreve.72.046127
-
Haznagy A., Fi I., London A., Nemeth T. Complex network analysis of public transportation networks: A comprehensive study // Proc. of the 2015 International Conference on Models and Technologies for Intelligent Transportation Systems (MT-ITS). 2015. P. 371–378. https://doi.org/10.1109/mtits.2015.7223282
-
Yang X.-H., Chen G., Chen S.-Y., Wang W.-L., Wang L. Study on some bus transport networks in china with considering spatial characteristics // Transportation Research Part A: Policy and Practice. 2014. V. 69. N 1. P. 1–10.https://doi.org/10.1016/j.tra.2014.08.004
-
Zhang J., Zhao M., Liu H., Xu X. Networked characteristics of the urban rail transit networks // Physica A: Statistical Mechanics and its Applications. 2013. V. 392. N 6. P. 1538–1546. https://doi.org/10.1016/j.physa.2012.11.036
-
Wang L.-N., Wang K., Shen J.-L. Weighted complex networks in urban public transportation: Modeling and testing //Physica A: Statistical Mechanics and its Applications. 2020. V. 545. P. 123498. https://doi.org/10.1016/j.physa.2019.123498
-
Shanmukhappa T., Ho I.W.-H., Chi K.T. Spatial analysis of bus transport networks using network theory // Physica A: Statistical Mechanics and its Applications. 2018. V. 502. P. 295–314. https://doi.org/10.1016/j.physa.2018.02.111
-
Wang Y., Deng Y., Ren F., Zhu R., Wang P., Du T., Du Q. Analysing the spatial configuration of urban bus networks based on the geospatial network analysis method // Cities. 2020. V. 96. P. 102406. https://doi.org/10.1016/j.cities.2019.102406
-
Lantseva A., Ivanov S. Modeling transport accessibility with open data: Case study of st. Petersburg // Procedia Computer Science. 2016. V. 101. P. 197–206. https://doi.org/10.1016/j.procs.2016.11.024
-
Bothorel C., Cruz J., Magnani M., Micenková B. Clustering attributed graphs: Models, measures and methods // Network Science. 2015. V. 3. N 3. P. 408–444. https://doi.org/10.1017/nws.2015.9
-
Chunaev P. Community detection in node-attributed social networks: A survey // Computer Science Review. 2020. V. 37. P. 100286. https://doi.org/10.1016/j.cosrev.2020.100286
-
Atzmueller M., Günnemann S., Zimmermann A. Mining communities and their descriptions on attributed graphs: a survey // Data Mining and Knowledge Discovery. 2021. V. 35. N 3. P. 661–687. https://doi.org/10.1007/s10618-021-00741-z
-
Rossi R.A., Ahmed N.K. Role discovery in networks // IEEE Transactions on Knowledge and Data Engineering. 2015. V. 27. N 4. P. 1112–1131. https://doi.org/10.1109/tkde.2014.2349913
-
Ahmed N.K., Rossi R.A., Willke T.L., Zhou R. Revisiting role discovery in networks: From node to edge roles // ArXiv. 2016. arXiv:1610.00844. https://doi.org/10.48550/arXiv.1610.00844
-
Martínez V., Berzal F., Cubero J.-C. An automorphic distance metric and its application to node embedding for role mining // Complexity. 2021. V. 2021. P. 1–17. https://doi.org/10.1155/2021/5571006
-
Gupte P.V., Ravindran B., Parthasarathy S. Role discovery in graphs using global features: Algorithms, applications and a novel evaluation strategy // Proc. of the IEEE 33rd International Conference on Data Engineering (ICDE). 2017. P. 771–782. https://doi.org/10.1109/icde.2017.128
-
Revelle M., Domeniconi C., Johri A. Persistent roles in online social networks // Lecture Notes in Computer Science. 2016. V. 9852. P. 47–62.
-
Rossi R.A., Gallagher B., Neville J., Henderson K. Modeling dynamic behavior in large evolving graphs // Proc. of the Sixth ACM International Conference on Web Search and Data Mining (WSDM’13). 2013. P. 667–676. https://doi.org/10.1145/2433396.2433479
-
Vega D., Meseguer R., Freitag F., Magnani M. Role and position detection in networks: Reloaded // Proc. of the 2015 IEEE/ACM International Conference on Advances in Social Networks Analysis and Mining (ASONAM). 2015. P. 320–325. https://doi.org/10.1145/2808797.2809412
-
Yang Z., Algesheimer R., Tessone C.J. A comparative analysis of community detection algorithms on artificial networks // Scientific Reports. 2016. V. 6. N 1. P. 30750. https://doi.org/10.1038/srep30750
-
Fortunato S. Community detection in graphs // Physics Reports. 2010. V. 486. N 3-5. P. 75–174. https://doi.org/10.1016/j.physrep.2009.11.002
-
Souravlas S., Sifaleras A., Tsintogianni M., Katsavounis S. A classification of community detection methods in social networks: a survey // International Journal of General Systems. 2021. V. 50. N 1. P. 63–91. https://doi.org/10.1080/03081079.2020.1863394
-
Bartal A., Ravid G. Member behavior in dynamic online communities: Role affiliation frequency model // IEEE Transactions on Knowledge and Data Engineering. 2020. V. 32. N 9. P. 1773–1784. https://doi.org/10.1109/tkde.2019.2911067
-
Ni W., Guo H., Liu T., Zeng Q. Automatic role identification for research teams with ranking multi-view machines // Knowledge and Information Systems. 2020. V. 62. N 12. P. 4681–4716. https://doi.org/10.1007/s10115-020-01504-w
-
Liu S., Toriumi F., Nishiguchi M., Usui S. Multiple role discovery in complex networks // Studies in Computational Intelligence. 2022. V. 1016. P. 415–427. https://doi.org/10.1007/978-3-030-93413-2_35
-
Liu Y., Du F., Sun J., Silva T., Jiang Y., Zhu T. Identifying social roles using heterogeneous features in online social networks // Journal of the Association for Information Science and Technology. 2019. V. 70. N 7. P. 660–674. https://doi.org/10.1002/asi.24160
-
Zhang L., Lu J., Fu B., Li S. A review and prospect for the complexity and resilience of urban public transit network based on complex network theory // Complexity. 2018. V. 2018. P. 1–36. https://doi.org/10.1155/2018/2156309
-
Shanmukhappa T., Ho I.W.-H., Tse C.K., Leung K.K. Recent development in public transport network analysis from the complex network perspective // IEEE Circuits and Systems Magazine. 2019. V. 19. N 4. P. 39–65. https://doi.org/10.1109/mcas.2019.2945211
-
Xu Q., Mao B., Bai Y. Network structure of subway passenger flows // Journal of Statistical Mechanics: Theory and Experiment. 2016. V. 2016. N 3. P. 033404. https://doi.org/10.1088/1742-5468/2016/03/033404
-
Feng J., Li X., Mao B., Xu Q., Bai Y. Weighted complex network analysis of the beijing subway system: Train and passenger flows // Physica A: Statistical Mechanics and its Applications. 2017. V. 474. P. 213–223. https://doi.org/10.1016/j.physa.2017.01.085
-
Faust K., Wasserman S. Blockmodels: Interpretation and evaluation // Social Networks. 1992. V. 14. N 1. P. 5–61. https://doi.org/10.1016/0378-8733(92)90013-w
-
Batagelj V., Mrvar A., Ferligoj A., Doreian P. Generalized blockmodeling with Pajek // Metodološki zvezki. 2004. V. 1. N 2. P. 455–467. https://doi.org/10.51936/ofaw1880
-
Luczkovich J., Borgatti S., Johnson J.C., Everett M.G. Defining and measuring trophic role similarity in food webs using regular equivalence // Journal of theoretical biology. 2003. V. 220. N 3. P. 303–21. https://doi.org/10.1006/jtbi.2003.3147
-
Ma H., Zhou D., Liu C., Lyu M.R., King I. Recommender systems with social regularization // Proc. of the Fourth ACM International Conference on Web Search and Data Mining (WSDM’11). 2011. P. 287–296. https://doi.org/10.1145/1935826.1935877
-
Golder S.A., Donath J. Social roles in electronic communities // Internet Research. 2004. V. 5.
-
Yue H., Guan Q., Pan Y., Chen L., Lv J., Yao Y. Detecting clusters over intercity transportation networks using k-shortest paths and hierarchical clustering: a case study of mainland China // International Journal of Geographical Information Science. 2019. V. 33. N 5. P. 1082–1105. https://doi.org/10.1080/13658816.2019.1566551
-
Bereznyi D., Qutbuddin A., Her Y., Yang K. Node-attributed spatial graph partitioning // Proc. of the 28th International Conference on Advances in Geographic Information Systems (SIGSPATIAL’20). 2020. P. 58–67. https://doi.org/10.1145/3397536.3422198
-
MacQueen J. Some methods for classification and analysis of multivariate observations // Proc. of the Fifth Berkeley Symposium on Mathematical Statistics and Probability. V. 1. Statistics. 1967. P. 281–297.