Меню
Публикации
2025
2024
2023
2022
2021
2020
2019
2018
2017
2016
2015
2014
2013
2012
2011
2010
2009
2008
2007
2006
2005
2004
2003
2002
2001
Главный редактор
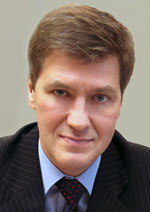
НИКИФОРОВ
Владимир Олегович
д.т.н., профессор
Партнеры
doi: 10.17586/2226-1494-2023-23-4-720-733
УДК 004.056
Атаки на основе вредоносных возмущений на системы обработки изображений и методы защиты от них
Читать статью полностью

Язык статьи - русский
Ссылка для цитирования:
Аннотация
Ссылка для цитирования:
Есипов Д.А., Бучаев А.Я., Керимбай А., Пузикова Я.В., Сайдумаров С.К., Сулименко Н.С., Попов И.Ю., Кармановский Н.С. Атаки на основе вредоносных возмущений на системы обработки изображений и методы защиты от них // Научно-технический вестник информационных технологий, механики и оптики. 2023. Т. 23, № 4. С. 720–733. doi: 10.17586/2226-1494-2023-23-4-720-733
Аннотация
Системы, реализующие технологии искусственного интеллекта, получили широкое распространение благодаря их эффективности в решении прикладных задач, включая компьютерное зрение. Обработка изображений посредством нейронных сетей применяется в критически важных для безопасности системах. В то же время использование искусственного интеллекта сопряжено с характерными угрозами, к которым относится и нарушение работы моделей машинного обучения. Феномен провокации некорректного отклика нейронной сети посредством внесения визуально незаметных человеку искажений впервые описан и привлек внимание исследователей в 2013 году. Методы атак на нейронные сети на основе вредоносных возмущений непрерывно совершенствовались, были предложены способы нарушения работы нейронных сетей при обработке различных типов данных и задач целевой модели. Угрозы нарушения функционирования нейронных сетей посредством указанных атак стала значимой проблемой для систем, реализующих технологии искусственного интеллекта. Таким образом, исследования в области противодействия атакам на основе вредоносных возмущений являются весьма актуальными. В данной статье представлено описание актуальных атак, приведен обзор и сравнительный анализ таких атак на системы обработки изображений с использованием искусственного интеллекта. Сформулированы подходы к классификации атак на основе вредоносных возмущений. Рассмотрены методы защиты от подобных атак, выявлены их недостатки. Показаны ограничения применяемых методов защиты, снижающие эффективность противодействия атакам. Предложены подходы по обнаружению и устранению вредоносных возмущений.
Ключевые слова: искусственный интеллект, искусственная нейронная сеть, обработка изображений, состязательная атака, встраивание бэкдора, вредоносное возмущение, состязательное обучение, защитная дистилляция, сжатие параметров, сертификационная защита, предобработка данных
Список литературы
Список литературы
- Goldberg Y. A primer on neural network models for natural language processing // Journal of Artificial Intelligence Research. 2016. V. 57. P. 345–420. https://doi.org/10.1613/jair.4992
- Nassif A.B., Shahin I., Attili I., Azzeh M., Shaalan K. Speech recognition using deep neural networks: A systematic review // IEEE Access. 2019. V. 7. P. 19143–19165. https://doi.org/10.1109/access.2019.2896880
- Almabdy S., Elrefaei L. Deep convolutional neural network-based approaches for face recognition // Applied Sciences. 2019. V. 9. N 20. P. 4397. https://doi.org/10.3390/app9204397
- Khan M.Z., Harous S., Hassan S. U., Khan M. U. G., Iqbal R., Mumtaz S. Deep unified model for face recognition based on convolution neural network and edge computing // IEEE Access. 2019. V. 7. P. 72622–72633. https://doi.org/10.1109/access.2019.2918275
- Zhang Y., Shi D., Zhan X., Cao D., Zhu K., Li Z. Slim-ResCNN: A deep residual convolutional neural network for fingerprint liveness detection // IEEE Access. 2019. V. 7. P. 91476–91487. https://doi.org/10.1109/access.2019.2927357
- Sarvamangala D.R., Kulkarni R.V. Convolutional neural networks in medical image understanding: a survey //Evolutionary Intelligence. 2022. V. 15. N 1. P. 1–22. https://doi.org/10.1007/s12065-020-00540-3
- Mahmood M., Al-Khateeb B., Alwash W. A review on neural networks approach on classifying cancers // IAES International Journal of Artificial Intelligence. 2020. V. 9. N 2. P. 317–326. http://doi.org/10.11591/ijai.v9.i2.pp317-326
- Singh V., Singh S., Gupta P. Real-time anomaly recognition through CCTV using neural networks // Procedia Computer Science. 2020. V. 173. P. 254–263. https://doi.org/10.1016/j.procs.2020.06.030
- Severino A., Curto S., Barberi S., Arena F., Pau G. Autonomous vehicles: an analysis both on their distinctiveness and the potential impact on urban transport systems // Applied Sciences. 2021. V. 11. N 8. P. 3604. https://doi.org/10.3390/app11083604
- Wang L., Fan X., Chen J., Cheng J., Tan J., Ma X. 3D object detection based on sparse convolution neural network and feature fusion for autonomous driving in smart cities // Sustainable Cities and Society. 2020. V. 54. P. 102002. https://doi.org/10.1016/j.scs.2019.102002
- Chen L., Lin S., Lu X., Cao D., Wu H., Guo C., Wang F. Y. Deep neural network based vehicle and pedestrian detection for autonomous driving: A survey // IEEE Transactions on Intelligent Transportation Systems. 2021. V. 22. N 6. P. 3234–3246. https://doi.org/10.1109/tits.2020.2993926
- Chen P. Y., Liu S. Holistic adversarial robustness of deep learning models // Proceedings of the AAAI Conference on Artificial Intelligence. 2023. V. 37. N 13. P. 15411–15420. https://doi.org/10.1609/aaai.v37i13.26797
- Huang X., Kroening D., Ruan W., Sharp J., Sun Y., Thamo E., Min W., Yi X. A survey of safety and trustworthiness of deep neural networks: Verification, testing, adversarial attack and defence, and interpretability // Computer Science Review. 2020. V. 37. P. 100270. https://doi.org/10.1016/j.cosrev.2020.100270
- Szegedy C., Zaremba W., Sutskever I., Bruna J., Erhan D., Goodfellow I., Fergus R. Intriguing properties of neural networks // arXiv. 2013. arXiv:1312.6199. https://doi.org/10.48550/arXiv.1312.6199
- Song Y., Shu R., Kushman N., Ermon S. Constructing unrestricted adversarial examples with generative models // Advances in Neural Information Processing Systems. 2018. V. 31.
- Sayghe A., Zhao J., Konstantinou C. Evasion attacks with adversarial deep learning against power system state estimation // Proc. of the 2020 IEEE Power & Energy Society General Meeting (PESGM). 2020. P. 1–5. https://doi.org/10.1109/pesgm41954.2020.9281719
- Goodfellow I.J., Shlens J., Szegedy C. Explaining and harnessing adversarial examples // arXiv. 2014. arXiv:1412.6572. https://doi.org/10.48550/arXiv.1412.6572
- Paul R., Schabath M., Gillies R., Hall L., Goldgof D. Mitigating adversarial attacks on medical image understanding systems // Proc. of the 2020 IEEE 17th International Symposium on Biomedical Imaging (ISBI). 2020. P. 1517–1521. https://doi.org/10.1109/isbi45749.2020.9098740
- Rozsa A., Rudd E.M., Boult T.E. Adversarial diversity and hard positive generation // Proc. of the IEEE Conference on Computer Vision and Pattern Recognition Workshops (CVPRW). 2016. P. 25–32. https://doi.org/10.1109/cvprw.2016.58
- Dong Y., Liao F., Pang T., Su H., Zhu J., Hu X., Li J. Boosting adversarial attacks with momentum // Proc. of the IEEE/CVF Conference on Computer Vision and Pattern Recognition. 2018. P. 9185–9193. https://doi.org/10.1109/cvpr.2018.00957
- Miyato T., Maeda S.I., Koyama M., Ishii S. Virtual adversarial training: a regularization method for supervised and semi-supervised learning // IEEE Transactions on Pattern Analysis and Machine Intelligence. 2019. V. 41. N 8. P. 1979–1993. https://doi.org/10.1109/tpami.2018.2858821
- Kurakin A., Goodfellow I.J., Bengio S. Adversarial examples in the physical world // Artificial Intelligence Safety and Security. Chapman and Hall/CRC, 2018. P. 99–112. https://doi.org/10.1201/9781351251389-8
- Mądry A., Makelov A., Schmidt L., Tsipras D., Vladu A. Towards deep learning models resistant to adversarial attacks // Stat. 2017. V. 1050. P. 9.
- Xie C., Zhang Z., Zhou Y., Bai S., Wang J., Ren Z., Yuille A.L. Improving transferability of adversarial examples with input diversity // Proc. of the IEEE/CVF Conference on Computer Vision and Pattern Recognition. 2019. P. 2730–2739. https://doi.org/10.1109/cvpr.2019.00284
- Dong X., Han J., Chen D., Liu J., Bian H., Ma Z., Li H., Wang X., Zhang W., Yu N. Robust superpixel-guided attentional adversarial attack // Proc. of the IEEE/CVF Conference on Computer Vision and Pattern Recognition. 2020. P. 12895–12904. https://doi.org/10.1109/cvpr42600.2020.01291
- Sriramanan G., Addepalli S., Baburaj A. Guided adversarial attack for evaluating and enhancing adversarial defenses // Advances in Neural Information Processing Systems. 2020. V. 33. P. 20297–20308.
- Rony J., Hafemann L.G., Oliveira L.S., Ayed I.B., Sabourin R., Granger E. Decoupling direction and norm for efficient gradient-based L2 adversarial attacks and defenses // Proc. of the IEEE/CVF Conference on Computer Vision and Pattern Recognition. 2019. P. 4322–4330. https://doi.org/10.1109/cvpr.2019.00445
- Moosavi-Dezfooli S.M., Fawzi A., Frossard P. DeepFool: a simple and accurate method to fool deep neural networks // Proc. of the IEEE Conference on Computer Vision and Pattern Recognition (CVPR). 2016. P. 2574–2582. https://doi.org/10.1109/cvpr.2016.282
- Carlini N., Wagner D. Towards evaluating the robustness of neural networks // Proc. of the IEEE Symposium on Security and Privacy (SP). 2017. P. 39–57. https://doi.org/10.1109/sp.2017.49
- Yao Z., Gholami A., Xu P., Keutzer K., Mahoney M. W. Trust region based adversarial attack on neural networks // Proc. of the IEEE/CVF Conference on Computer Vision and Pattern Recognition (CVPR). 2019. P. 11350–11359. https://doi.org/10.1109/cvpr.2019.01161
- Papernot N., McDaniel P., Jha S., Fredrikson M., Celik Z. B., Swami A. The limitations of deep learning in adversarial settings // Proc. of the 2016 IEEE European Symposium on Security and Privacy (EuroS&P). 2016. P. 372–387. https://doi.org/10.1109/eurosp.2016.36
- Su J., Vargas D.V., Sakurai K. One pixel attack for fooling deep neural networks // IEEE Transactions on Evolutionary Computation. 2019. V. 23. N 5. P. 828–841. https://doi.org/10.1109/tevc.2019.2890858
- Moosavi-Dezfooli S.M., Fawzi A., Fawzi O., Frossard P. Universal adversarial perturbations // Proc. of the IEEE Conference on Computer Vision and Pattern Recognition (CVPR). 2017. P. 1765–1773. https://doi.org/10.1109/cvpr.2017.17
- Brendel W., Rauber J., Bethge M. Decision-based adversarial attacks: Reliable attacks against black-box machine learning models // Advances in Reliably Evaluating and Improving Adversarial Robustness. 2021. P. 77.
- Chen J., Jordan M.I., Wainwright M.J. HopSkipJumpAttack: A query-efficient decision-based attack // Proc. of the 2020 IEEE Symposium on Security and Privacy (SP). 2020. P. 1277–1294. https://doi.org/10.1109/sp40000.2020.00045
- Liu Y., Moosavi-Dezfooli S.M., Frossard P. A geometry-inspired decision-based attack // Proc. of the IEEE/CVF International Conference on Computer Vision (ICCV). 2019. P. 4890–4898. https://doi.org/10.1109/iccv.2019.00499
- Rahmati A., Moosavi-Dezfooli, S.M., Frossard P., Dai H. GeoDA: a geometric framework for black-box adversarial attacks // Proc. of the IEEE/CVF Conference on Computer Vision and Pattern Recognition (CVPR). 2020. P. 8446–8455. https://doi.org/10.1109/cvpr42600.2020.00847
- Du J., Zhang H., Zhou J.T., Yang Y., Feng J. Query-efficient meta attack to deep neural networks // Proc. of the International Conference on Learning Representations. 2020.
- Li J., Ji R., Liu H., Liu J., Zhong B., Deng C., Tian Q. Projection & probability-driven black-box attack // Proc. of the IEEE/CVF Conference on Computer Vision and Pattern Recognition (CVPR). 2020. P. 362–371. https://doi.org/10.1109/cvpr42600.2020.00044
- Li H., Xu X., Zhang X., Yang S., Li B. QEBA: Query-efficient boundary-based blackbox attack // Proc. of the IEEE/CVF Conference on Computer Vision and Pattern Recognition (CVPR). 2020. P. 1221–1230. https://doi.org/10.1109/cvpr42600.2020.00130
- Cheng M., Singh S., Chen P., Chen P.Y., Liu S., Hsieh C.J. Sign-OPT: A query-efficient hard-label adversarial attack // Proc. of the International Conference on Learning Representations. 2020.
- Brunner T., Diehl F., Le M.T., Knoll A. Guessing smart: Biased sampling for efficient black-box adversarial attacks // Proc. of the IEEE/CVF International Conference on Computer Vision (ICCV). 2019. P. 4958–4966. https://doi.org/10.1109/iccv.2019.00506
- Maho T., Furon T., Le Merrer E. SurFree: a fast surrogate-free black-box attack // Proc. of the IEEE/CVF Conference on Computer Vision and Pattern Recognition (CVPR). 2021. P. 10430–10439. https://doi.org/10.1109/cvpr46437.2021.01029
- Shi Y., Han Y., Tian Q. Polishing decision-based adversarial noise with a customized sampling // Proc. of the IEEE/CVF Conference on Computer Vision and Pattern Recognition (CVPR). 2020. P. 1030–1038. https://doi.org/10.1109/cvpr42600.2020.00111
- Huang Z., Zhang T. Black-box adversarial attack with transferable model-based embedding // Proc. of the International Conference on Learning Representations. 2020.
- Zhou M., Wu J., Liu Y., Liu S., Zhu C. DaST: Data-free substitute training for adversarial attacks // Proc. of the IEEE/CVF Conference on Computer Vision and Pattern Recognition (CVPR). 2020. P. 234–243. https://doi.org/10.1109/cvpr42600.2020.00031
- Zou J., Pan Z., Qiu J., Liu X., Rui T., Li W. Improving the transferability of adversarial examples with resized-diverse-inputs, diversity-ensemble and region fitting // Lecture Notes in Computer Science. 2020. V. 12367.P. 563–579. https://doi.org/10.1007/978-3-030-58542-6_34
- Wang X., He K. Enhancing the transferability of adversarial attacks through variance tuning // Proc. of the IEEE/CVF Conference on Computer Vision and Pattern Recognition (CVPR). 2021. P. 1924–1933. https://doi.org/10.1109/cvpr46437.2021.00196
- Wu W., Su Y., Lyu M.R., King I. Improving the transferability of adversarial samples with adversarial transformations // Proc. of the IEEE/CVF Conference on Computer Vision and Pattern Recognition (CVPR). 2021. P. 9024–9033. https://doi.org/10.1109/cvpr46437.2021.00891
- Hosseini H., Poovendran R. Semantic adversarial examples // Proc. of the IEEE/CVF Conference on Computer Vision and Pattern Recognition Workshops (CVPRW). 2018. P. 1614–1619. https://doi.org/10.1109/cvprw.2018.00212
- Engstrom L., Tran B., Tsipras D., Schmidt L., Madry A. A rotation and a translation suffice: Fooling cnns with simple transformations [Электронный ресурс]. URL: https://openreview.net/forum?id=BJfvknCqFQ (дата обращения: 29.05.2023).
- Joshi A., Mukherjee A., Sarkar S., Hegde C. Semantic adversarial attacks: Parametric transformations that fool deep classifiers // Proc. of the IEEE/CVF International Conference on Computer Vision (ICCV). 2019. P. 4773–4783. https://doi.org/10.1109/iccv.2019.00487
- Liu A., Wang J., Liu X., Cao B., Zhang C., Yu H. Bias-based universal adversarial patch attack for automatic check-out // Lecture Notes in Computer Science. 2020. V. 12358.P. 395–410. https://doi.org/10.1007/978-3-030-58601-0_24
- Swathi P., Sk S. DeepFake creation and detection: A survey // Proc. of the 2021 Third International Conference on Inventive Research in Computing Applications (ICIRCA). 2021. P. 584–588. https://doi.org/10.1109/icirca51532.2021.9544522
- Chadha A., Kumar V., Kashyap S., Gupta M. Deepfake: An Overview // Lecture Notes in Networks and Systems. 2021. V. 203. P. 557–566. https://doi.org/10.1007/978-981-16-0733-2_39
- Nakka K.K., Salzmann M. Indirect local attacks for context-aware semantic segmentation networks // Lecture Notes in Computer Science. 2020. V. 12350.P. 611–628. https://doi.org/10.1007/978-3-030-58558-7_36
- He Y., Rahimian S., Schiele B., Fritz M. Segmentations-leak: Membership inference attacks and defenses in semantic image segmentation // Lecture Notes in Computer Science. 2020. V. 12368.P. 519–535. https://doi.org/10.1007/978-3-030-58592-1_31
- Choi J.H. Zhang H., Kim J.H., Hsieh C.J., Lee J.S. Evaluating robustness of deep image super-resolution against adversarial attacks // Proc. of the IEEE/CVF International Conference on Computer Vision (ICCV). 2019. P. 303–311. https://doi.org/10.1109/iccv.2019.00039
- Jiang L., Ma X., Chen S., Bailey J., Jiang Y.G. Black-box adversarial attacks on video recognition models // Proc. of the 27th ACM International Conference on Multimedia. 2019. P. 864–872. https://doi.org/10.1145/3343031.3351088
- Li S., Aich A., Zhu S., Asif S., Song C., Roy-Chowdhury A., Krishnamurthy S. Adversarial attacks on black box video classifiers: Leveraging the power of geometric transformations // Advances in Neural Information Processing Systems. 2021. V. 34. P. 2085–2096.
- Chen X., Li S., Huang H. Adversarial attack and defense on deep neural network-based voice processing systems: An overview // Applied Sciences. 2021. V. 11. N 18. P. 8450. https://doi.org/10.3390/app11188450
- Kwon H., Kim Y., Yoon H., Choi D. Selective audio adversarial example in evasion attack on speech recognition system // IEEE Transactions on Information Forensics and Security. 2020. V. 15. P. 526–538. https://doi.org/10.1109/tifs.2019.2925452
- Usama M., Qayyum A., Qadir J., Al-Fuqaha A. Black-box adversarial machine learning attack on network traffic classification // Proc. of the 15th International Wireless Communications & Mobile Computing Conference (IWCMC). 2019. P. 84–89. https://doi.org/10.1109/iwcmc.2019.8766505
- Imam N.H., Vassilakis V.G. A survey of attacks against twitter spam detectors in an adversarial environment // Robotics. 2019. V. 8. N 3. P. 50. https://doi.org/10.3390/robotics8030050
- Zhong H., Liao C., Squicciarini A.C., Zhu S., Miller D. Backdoor embedding in convolutional neural network models via invisible perturbation // Proc. of the Tenth ACM Conference on Data and Application Security and Privacy. 2020. P. 97–108. https://doi.org/10.1145/3374664.3375751
- Liu X., Yang H., Liu Z., Song L., Li H., Chen Y. Dpatch: An adversarial patch attack on object detectors // arXiv. 2018. arXiv:1806.02299. https://doi.org/10.48550/arXiv.1806.02299
- Liu Y., Ma X., Bailey J., Lu F. Reflection backdoor: A natural backdoor attack on deep neural networks // Lecture Notes in Computer Science. 2020. V. 12355. P. 182–199. https://doi.org/10.1007/978-3-030-58607-2_11
- Nguyen A., Tran A. WaNet - imperceptible warping-based backdoor attack // Proc. of the International Conference on Learning Representations. 2021.
- Костюмов В.В. Обзор ис истематизация атак уклонением на модели компьютерного зрения // International Journal of Open Information Technologies. 2022. Т. 10. № 10. С. 11–20.
- Papernot N., McDaniel P., Wu X., Jha S., Swami A. Distillation as a defense to adversarial perturbations against deep neural networks // Proc. of the 2016 IEEE Symposium on Security and Privacy (SP). 2016. P. 582–597. https://doi.org/10.1109/sp.2016.41
- Steihaug T. The conjugate gradient method and trust regions in large scale optimization // SIAM Journal on Numerical Analysis. 1983. V. 20. N 3. P. 626–637. https://doi.org/10.1137/0720042
- Curtis A.R., Powell M.J.D., Reid J.K. On the estimation of sparse Jacobian matrices // IMA Journal of Applied Mathematics. 1974. V. 13. N 1. P. 117–120. https://doi.org/10.1093/imamat/13.1.117
- Niebur E. Saliency map // Scholarpedia. 2007. V. 2. N 8. С. 2675. https://doi.org/10.4249/scholarpedia.2675
- Das S., Suganthan P.N. Differential evolution: A survey of the state-of-the-art // IEEE Transactions on Evolutionary Computation. 2011. V. 15. N 1. P. 4–31. https://doi.org/10.1109/tevc.2010.2059031
- Badrinarayanan V., Kendall A., Cipolla R. SegNet: A deep convolutional encoder-decoder architecture for image segmentation // IEEE Transactions on Pattern Analysis and Machine Intelligence. 2017. V. 39. N 12. P. 2481–2495. https://doi.org/10.1109/tpami.2016.2644615
- Lowd D., Meek C. Adversarial learning // Proc. of the Eleventh ACM SIGKDD International Conference on Knowledge Discovery in Data Mining. 2005. P. 641–647. https://doi.org/10.1145/1081870.1081950
- Xu W., Evans D., Qi Y. Feature squeezing: Detecting adversarial examples in deep neural networks // Proc. of the 2018 Network and Distributed System Security Symposium (NDSS). 2018.
- Liao F., Liang M., Dong Y., Pang T., Hu X., Zhu J. Defense against adversarial attacks using high-level representation guided denoiser // Proc. of the 2018 IEEE/CVF Conference on Computer Vision and Pattern Recognition. 2018. P. 1778–1787. https://doi.org/10.1109/cvpr.2018.00191
- Zhang D., Ye M., Gong C., Zhu Z., Liu Q. Black-box certification with randomized smoothing: A functional optimization based framework // Advances in Neural Information Processing Systems. 2020. V. 33. P. 2316–2326.
- Fischer M., Baader M., Vechev M. Certified defense to image transformations via randomized smoothing // Advances in Neural Information Processing Systems. 2020. V. 33. P. 8404–8417.
- Yang R., Chen X.Q., Cao T.J. APE-GAN++: An improved APE-GAN to eliminate adversarial perturbations // IAENG International Journal of Computer Science. 2021. V. 48. N 3. P. 827–844.
- Glenn T.C., Zare A., Gader P.D. Bayesian fuzzy clustering // IEEE Transactions on Fuzzy Systems. 2015. V. 23. N 5. P. 1545–1561. https://doi.org/10.1109/tfuzz.2014.2370676
- Plackett R.L. Karl Pearson and the chi-squared test // International Statistical Review / Revue Internationale de Statistique. 1983. V. 51. N 1. P. 59–72. https://doi.org/10.2307/1402731
- McLachlan G.J. Mahalanobis distance // Resonance. 1999. V. 4. N 6. P. 20–26. https://doi.org/10.1007/BF02834632