Меню
Публикации
2025
2024
2023
2022
2021
2020
2019
2018
2017
2016
2015
2014
2013
2012
2011
2010
2009
2008
2007
2006
2005
2004
2003
2002
2001
Главный редактор
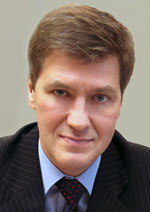
НИКИФОРОВ
Владимир Олегович
д.т.н., профессор
Партнеры
doi: 10.17586/2226-1494-2025-25-1-42-52
УДК 004.056
Большие языковые модели в информационной безопасности и тестировании на проникновение: систематический обзор возможностей применения
Читать статью полностью

Язык статьи - русский
Ссылка для цитирования:
Аннотация
Ссылка для цитирования:
Конев А.А., Паюсова Т.И. Большие языковые модели в информационной безопасности и тестировании на проникновение: систематический обзор возможностей применения // Научно- технический вестник информационных технологий, механики и оптики. 2025. Т. 25, № 1. С. 42–52. doi: 10.17586/2226-1494-2025-25-1-42-52
Аннотация
Введение. Развитие технологий искусственного интеллекта, в частности, больших языковых моделей (Large Learning Model, LLM), привело к изменениям во многих сферах жизни и деятельности человека. Информационная безопасность также претерпела существенные изменения. Тестирование на проникновение (пентест) позволяет оценить систему защиты на практике в «боевых» условиях. LLM могут вывести практический анализ защищенности на качественно новый уровень с точки зрения автоматизации и возможности генерации нестандартных шаблонов атаки. Представленный в работе систематический обзор направлен на определение уже известных способов применения LLM в кибербезопасности, а также на выявление «белых пятен» в развитии технологии. Метод. Отбор исследуемых научных работ осуществлялся в соответствии с многоступенчатым руководством PRISMA на основании анализа аннотаций и ключевых слов публикаций. Полученная выборка была дополнена с помощью метода «снежного кома» и ручного поиска статей. Суммарное количество публикаций составило 50 работ с января 2023 г. по март 2024 г. Основные результаты. В работе выполнен анализ способов применения LLM в области информационной безопасности (поддержка целеполагания и принятия решений, автоматизация пентеста, анализ защищенности моделей LLM и программного кода). Определены архитектуры LLM (GPT-4, GPT-3.5, Bard, LLaMA, LLaMA 2, BERT, Mixtral 8×7B Instruct, FLAN, Bloom) и программные решения на базе LLM (GAIL-PT, AutoAttacker, NetSecGame, Cyber Sentinel, Microsoft Counterfit, GARD project, GPTFUZZER, VuRLE), применяемые в области информационной безопасности. Установлены ограничения (конечное «время жизни» данных для обучения LLM, недостаточные когнитивные способности языковых моделей, отсутствие самостоятельного целеполагания и сложности при адаптации LLM к новым параметрам задачи). Выявлены потенциальные точки роста и развития технологии в контексте киберзащиты (исключение «галлюцинаций» моделей и обеспечение защиты LLM от джейлбрейков, осуществление интеграции известных разрозненных решений и программная автоматизация выполнения задач в области информационной безопасности с помощью LLM). Обсуждение. Полученные результаты могут быть полезны при разработке собственных теоретических и практических решений, обучающих и тренировочных наборов данных, программных комплексов и инструментов для проведения тестирования на проникновение. Исследование поможет в реализации новых подходов к построению LLM и повышению их когнитивных способностей, учитывающих аспекты работы с джейлбрейками и «галлюцинациями», а также для самостоятельного дальнейшего многостороннего изучения вопроса.
Ключевые слова: обработка естественного языка, компьютерная лингвистика, ChatGPT, пентест, искусственный интеллект, машинное обучение, наступательная безопасность, моделирование атак, автоматизация, Red Teaming, джейлбрейк
Благодарности. Работа выполнена при финансовой поддержке Министерства науки и высшего образования РФ в рамках базовой части государственного задания ТУСУРа на 2023–2025 гг. (проект № FEWM-2023-0015).
Список литературы
Благодарности. Работа выполнена при финансовой поддержке Министерства науки и высшего образования РФ в рамках базовой части государственного задания ТУСУРа на 2023–2025 гг. (проект № FEWM-2023-0015).
Список литературы
- Konev A., Shelupanov A., Kataev M., Ageeva V., Nabieva A. A survey on threat-modeling techniques: protected objects and classification of threats // Symmetry. 2022. V. 14. N3. P. 549. https://doi.org/10.3390/sym14030549
- Shelupanov A., Evsyutin O., Konev A., Kostyuchenko E., Kruchinin D., Nikiforov D. Information security methods–Modern research directions // Symmetry. 2019. V. 11. N 2. P. 150. https://doi.org/10.3390/sym11020150
- Yao Y., Duan J., Xu K., Cai Y., Sun Z., Zhang Y. A survey on large language model (LLM) security and privacy: The Good, the Bad, and the Ugly // High-Confidence Computing. 2024. V. 4. N 2. P. 100211. https://doi.org/10.1016/j.hcc.2024.100211
- da Silva G.J.C., Westphall C.B. A Survey of Large Language Models in Cybersecurity // arXiv. 2024. arXiv:2402.16968. https://doi.org/10.48550/arXiv.2402.16968
- Wang L., Ma C., Feng X., Zhang Z., Yang H., Zhang J., Chen Z., Tang J., Chen X., Lin Y., Zhao W., Wei Z., Wen J. A survey on large language model based autonomous agents // Frontiers of Computer Science. 2024. V. 18. N 6. P. 186345. https://doi.org/10.1007/s11704-024-40231-1
- Gupta M., Akiri C., Aryal K., Parker E., Praharaj L. From ChatGPT to ThreatGPT: Impact of generative ai in cybersecurity and privacy // IEEE Access. 2023. V. 11. P. 80218–80245. https://doi.org/10.1109/ACCESS.2023.3300381
- Happe A., Cito J. Getting pwn’d by ai: Penetration testing with large language models // Proc. of the 31st ACM Joint European Software Engineering Conference and Symposium on the Foundations of Software Engineering. 2023. P. 2082–2086. https://doi.org/10.1145/3611643.3613083
- Chang Y., Wang X., Wang J., Wu Y., Yang L., Zhu K., Chen H., Yi X., Wang C., Wang Y., Ye W., Zhang Y., Chang Y., Yu P., Yang Q., Xie X. A survey on evaluation of Large Language Models // ACM Transactions on Intelligent Systems and Technology. 2024. V. 15. N 3. P. 1–45. https://doi.org/10.1145/3641289
- Li Z., Cao Y., Xu X., Jiang J., Liu X., Teo Y., Lin S., Liu Y. LLMs for Relational Reasoning: How Far are We? // Proc. of the 1st International Workshop on Large Language Models for Code (LLM4Code '24). 2024. P. 119–126. https://doi.org/10.1145/3643795.3648387
- Genevey-Metat C., Bachelot D., Gourmelen T., Quemat A., Satre P.-M., Scotto L., Di Perrotolo, Chaux M., Delesques P., Gesny O. Red Team LLM: towards an adaptive and robust automation solution // Proc. of the Conference on Artificial Intelligence for Defense. 2023. hal-04328468.
- Franco M.F., Rodrigues B., Scheid E., Jacobs A., Killer C., Granville L., Stiller B. SecBot: a business-driven conversational agent for cybersecurity planning and management // Proc. of the 16th International Conference on Network and Service management (CNSM). 2020. P. 1–7. https://doi.org/10.23919/cnsm50824.2020.9269037
- Chamberlain D., Casey E. Capture the Flag with ChatGPT: Security Testing with AI ChatBots // Proc. of the 19th International Conference on Cyber Warfare and Security (ICCWS). 2024. V. 19. N 1. P. 43–54. https://doi.org/10.34190/iccws.19.1.2171
- Timmins J., Knight S., Lachine B. Offensive cyber security trainer for platform management systems // Proc. of the IEEE International Systems Conference (SysCon). 2021. P. 1–8. https://doi.org/10.1109/syscon48628.2021.9447060
- Raman R., Calyam P., Achuthan K. ChatGPT or Bard: Who is a better Certified Ethical Hacker? // Computers & Security. 2024. V. 140. P. 103804. https://doi.org/10.1016/j.cose.2024.103804
- Abualhaija S., Arora C., Sleimi A., Briand L. Automated question answering for improved understanding of compliance requirements: A multi-document study // Proc. of the IEEE 30th International Requirements Engineering Conference (RE). 2022. P. 39–50. https://doi.org/10.1109/re54965.2022.00011
- Renaud K., Warkentin M., Westerman G. From ChatGPT to HackGPT: Meeting the cybersecurity threat of generative AI // MIT Sloan Management Review. 2023.
- Yamin M.M., Hashmi E., Ullah M., Katt B. Applications of LLMs for generating cyber security exercise scenarios // IEEE Access. 2024. V. 12. P. 143806–143822. https://doi.org/10.1109/access.2024.3468914
- Heim M.P., Starckjohann N., Torgersen M. The Convergence of AI and Cybersecurity: An Examination of ChatGPT's Role in Penetration Testing and its Ethical and Legal Implications. BS thesis. NTNU, 2023.
- Chen J., Hu S., Zheng H., Xing C., Zhang G. GAIL-PT: An intelligent penetration testing framework with generative adversarial imitation learning // Computers & Security. 2023. V. 126. P. 103055. https://doi.org/10.1016/j.cose.2022.103055
- Ананьев В.А., Человечкова А.В. ChatGPT в сфере информационной безопасности // Информационная безопасность цифровой экономики. 2023. С. 77–83.
- Прохоров А.И. Киберполигон как современный инструмент обеспечения информационной безопасности // Информатизация в цифровой экономике. 2023. Т. 4. № 4. С. 363–378. https://doi.org/10.18334/ide.4.4.119301
- Chen Y., Yao Y., Wang X., Xu D., Yue C., Liu X., Chen K., Tang H., Liu B. Bookworm game: Automatic discovery of LTE vulnerabilities through documentation analysis // Proc. of the IEEE Symposium on Security and Privacy (SP). 2021. P. 1197–1214. https://doi.org/10.1109/sp40001.2021.00104
- Ren Z., Ju X., Chen X., Shen H. ProRLearn: boosting prompt tuning-based vulnerability detection by reinforcement learning // Automated Software Engineering. 2024. V. 31. N 2. P. 38. https://doi.org/10.1007/s10515-024-00438-9
- Hoffmann J. Simulated penetration testing: from "Dijkstra" to "Turing Test++" // Proc. of the 25th International Conference on Automated Planning and Scheduling. 2015. V. 25. N 1. P. 364–372. https://doi.org/10.1609/icaps.v25i1.13684
- Dube R. Large Language Models in Information Security Research: A January 2024 Survey: preprint. 2024.
- Ai Z., Luktarhan N., Zhou A., Lv D. Webshell attack detection based on a deep super learner // Symmetry. 2020. V. 12. N 9. P. 1406. https://doi.org/10.3390/sym12091406
- Esmradi A., Yip D.W., Chan C.F. A Comprehensive Survey of Attack Techniques, Implementation, and Mitigation Strategies in Large Language Models // Communications in Computer and Information Science. 2024. V. 2034. P. 76–95. https://doi.org/10.1007/978-981-97-1274-8_6
- Gabrys R., Bilinski M., Fugate S., Silva D. Using natural language processing tools to infer adversary techniques and tactics under the Mitre ATT&CK framework // Proc. of the IEEE 14th Annual Computing and Communication Workshop and Conference. 2024. P. 541–547. https://doi.org/10.1109/CCWC60891.2024.10427746
- Ebert C., Beck M. Generative Artificial Intelligence for Automotive Cybersecurity // ATZelectronics worldwide. 2024. V. 19. N 1. P. 50–54. https://doi.org/10.1007/s38314-023-1564-3
- Kanakogi K., Washizaki H., Fukazawa Y., Ogata S., Okubo T., Kato T., Kanuka H., Hazeyama A., Yoshioka N. Tracing CVE Vulnerability Information to CAPEC Attack Patterns Using Natural Language Processing Techniques // Information. 2021. V. 12. N 8. P. 298. https://doi.org/10.3390/info12080298
- Radanliev P., De Roure D., Santos O. Red Teaming Generative AI/NLP, the BB84 Quantum Cryptography Protocol and the NIST-Approved Quantum-Resistant Cryptographic Algorithms // SSRN Electronic Journal. September 17, 2023. URL: https://ssrn.com/abstract=4574446 (дата обращения: 01.10.2024).
- Sun Y., Wu D., Xue Y., Liu H., Wang H., Xu Z., Xie X., Liu Y. GPTScan: Detecting Logic Vulnerabilities in Smart Contracts by Combining GPT with Program Analysis // Proc. of the IEEE/ACM 46th International Conference on Software Engineering (ICSE 2024). 2024. N 166. P. 1–13. https://doi.org/10.1145/3597503.3639117
- Al-Hawawreh M., Aljuhani A., Jararweh Y. Chatgpt for cybersecurity: practical applications, challenges, and future directions // Cluster Computing. 2023. V. 26. N 6. P. 3421–3436. https://doi.org/10.1007/s10586-023-04124-5
- Tsingenopoulos I., Preuveneers D., Joosen W. AutoAttacker: A reinforcement learning approach for black-box adversarial attacks // Proc. of the IEEE European Symposium on Security and Privacy Workshops (EuroS&PW). 2019. P. 229-237. https://doi.org/10.1109/EuroSPW.2019.00032
- Rigaki M., Lukas O., Catania C., Garcia S. Out of the cage: how stochastic parrots win in cyber security environments // Proc. of the 16th International Conference on Agents and Artificial Intelligence. 2024. V. 3. P. 774–781. https://doi.org/10.5220/0012391800003636
- Liu B., Xiao B., Jiang X., Cen S., He X., Dou W. Adversarial attacks on large language model-based system and mitigating strategies: A case study on ChatGPT // Security and Communication Networks. 2023. V. 2023. N 1. P. 8691095 https://doi.org/10.1155/2023/8691095
- Campbell M., Jovanovic M. Disinfecting AI: Mitigating Generative AI’s Top Risks // Computer. 2024. V. 57. N 5. P. 111–116. https://doi.org/10.1109/MC.2024.3374433
- Намиот Д.Е., Зубарева Е.В. О работе AI Red Team // International Journal of Open Information Technologies. 2023. Т. 11. № 10. P. 130–139.
- Shi Z., Wang Y., Yin F., Chen X., Chang K., Hsieh C. Red teaming language model detectors with language models // Transactions of the Association for Computational Linguistics. 2024. V. 12. P. 174–189. https://doi.org/10.1162/tacl_a_00639
- Alawida M., Mejri S., Mehmood A., Chikhaoui B., Abiodun O. A comprehensive study of ChatGPT: advancements, limitations, and ethical considerations in natural language processing and cybersecurity // Information. 2023. V. 14. N 8. P. 462. https://doi.org/10.3390/info14080462
- Chen Y., Arunasalam A., Celik Z.B. Can large language models provide Security & Privacy advice? measuring the ability of LLms to refute misconceptions // Proc. of the 39th Annual Computer Security Applications Conference. 2023. P. 366–378. https://doi.org/10.1145/3627106.3627196
- Bang Y., Cahyawijaya S., Lee N., Dai W., Su D., Wilie B., Lovenia H., Ji Z., Yu T., Chung W., Do Q.V., Xu Y, Fung P. A Multitask, Multilingual, Multimodal Evaluation of ChatGPT on Reasoning, Hallucination, and Interactivity // Proc. of the 13th International Joint Conference on Natural Language Processing and the 3rd Conference of the Asia-Pacific Chapter of the Association for Computational Linguistics. 2023. V. 1. P. 675–718. https://doi.org/10.18653/v1/2023.ijcnlp-main.45
- Xie Y., Yi J., Shao J., Curl J., Lyu L., Chen Q., Xie X., Wu F. Defending ChatGPT against jailbreak attack via self-reminders // Nature Machine Intelligence. 2023. V. 5. N 12. P. 1486–1496. https://doi.org/10.1038/s42256-023-00765-8
- Deng G., Liu Y., Li Y., Wang K., Zhang Y., Li Z., Wang H., Zhang T., Liu Y. MASTERKEY: Automated jailbreaking of large language model Chatbots // Proc. of the Network and Distributed System Security Symposium. 2024. P. 1–16. https://doi.org/10.14722/ndss.2024.24188
- Schulhoff S., Pinto J., Khan A., Bouchard L., Si C., Anati S., Tagliabue V., Kost A., Carnahan C., Boyd-Graber J. Ignore This Title and HackAPrompt: Exposing Systemic Vulnerabilities of LLMs Through a Global Prompt Hacking Competition // Proc. of the Conference on Empirical Methods in Natural Language Processing. 2023. P. 4945–4977. https://doi.org/10.18653/v1/2023.emnlp-main.302
- Liang H., Li X., Xiao D., Liu J., Zhou Y., Wang A., Li J. Generative Pre-Trained Transformer-Based reinforcement learning for testing Web Application Firewalls // IEEE Transactions on Dependable and Secure Computing. 2024. V. 21. N 1. P. 309–324. https://doi.org/10.1109/TDSC.2023.3252523
- Серобабов Д.С. Безопасность кода, созданного с помощью Chat GPT: анализ и исправление уязвимостей //Фундаментальные и прикладные исследования молодых учёных: сборник материалов VII Международной научно-практической конференции студентов, аспирантов и молодых учёных, приуроченной к 110-летию со дня рождения Т.В. Алексеевой, Омск, 20–21 апреля 2023 года. Омск: Сибирский государственный автомобильно-дорожный университет (СибАДИ). 2023. С. 615–620.
- Gasiba T.E., Oguzhan K., Kessba I., Lechner U., Pinto-Albuquerque M. I'm Sorry Dave, I'm Afraid I Can't Fix Your Code: On ChatGPT, CyberSecurity, and Secure Coding // Proc. of the 4th International Computer Programming Education Conference (ICPEC 2023). 2023.
- Lu G., Ju X., Chen X., Pei W., Cai Z. GRACE: Empowering LLM-based software vulnerability detection with graph structure and in-context learning // Journal of Systems and Software. 2024. V. 212. P. 112031. https://doi.org/10.1016/j.jss.2024.112031
- Wang J., Huang Y., Chen C., Liu Z., Wang S.,Wang Q. Software testing with Large Language Models: Survey, Landscape, and Vision // IEEE Transactions on Software Engineering. 2024. V. 50. N 4. P. 911–936. https://doi.org/10.1109/TSE.2024.3368208
- Chaudhary P.K. AI, ML, and Large Language Models in cybersecurity // International Research Journal of Modernization in Engineering Technology and Science. 2024. V. 6. N 2. P. 2229–2234. https://www.doi.org/10.56726/IRJMETS49546
- Botacin M. GPThreats-3: Is Automatic Malware Generation a Threat? // Proc. of the IEEE Security and Privacy Workshops (SPW). 2023. P. 238–254. https://www.doi.org/10.1109/SPW59333.2023.00027