Меню
Публикации
2025
2024
2023
2022
2021
2020
2019
2018
2017
2016
2015
2014
2013
2012
2011
2010
2009
2008
2007
2006
2005
2004
2003
2002
2001
Главный редактор
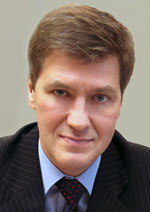
НИКИФОРОВ
Владимир Олегович
д.т.н., профессор
Партнеры
doi: 10.17586/2226-1494-2025-25-1-114-127
УДК 004.492.3
Улучшение и расширение CatBoost для точного обнаружения и классификации подтипов DoS и DDoS атак в сетевом трафике
Читать статью полностью

Язык статьи - английский
Ссылка для цитирования:
Аннотация
Ссылка для цитирования:
Хажжуз А., Авксентьева Е.Ю. Улучшение и расширение CatBoost для точного обнаружения и классификации подтипов DoS и DDoS атак в сетевом трафике // Научно-технический вестник информационных технологий, механики и оптики. 2025. Т. 25, № 1. С. 114–127 (на англ. яз.). doi: 10.17586/2226-1494-2025-25-1-114-127
Аннотация
В постоянно меняющемся цифровом мире рост сложных киберугроз, особенно атак DoS (отказ в обслуживании) и DDoS (распределенный отказ в обслуживании), представляет собой серьезную проблему для информационной безопасности. В работе рассматривается задача классификации вредоносного и безопасного сетевого трафика с спользованием применением классификатора CatBoost — алгоритма машинного обучения, оптимизированного для категориальных данных и несбалансированных наборов данных. Использованы наборы данных CIC- IDS2017 и CSE-CIC-IDS2018, которые имитируют различные сценарии кибератак. оптимизация классификатора CatBoost для распознавания конкретных подтипов атак DoS и DDoS, включая Hulk, SlowHTTPTest, GoldenEye, Slowloris, HOIC, LOIC-UDP-HTTP, LOIT. Разработана методика работы CatBoost для подготовки данных, отбора признаков и настройки модели, нормализации выбросов, корректировки отрицательных значений и улучшения структуры наборов данных. Стратифицированная выборка обеспечила сбалансированное представление классов в обучающих, валидационных и тестовых наборах. Разработанная модель CatBoost продемонстрировала отличные результаты с общей точностью 0,999922, высокой полнотой и значениями F1-меры по всем категориям и способностью обрабатывать более 3,4 млн образцов в секунду. Эти результаты показывают, что модель является надежной и подходит для обнаружения вторжений в реальном времени. Классификация конкретных типов атак улучшает точность системы обнаружения вторжений (Intrusion Detection Systems, IDS) и позволяет целенаправленно реагировать на различные угрозы. Существенное повышение точности обнаружения решает проблему несбалансированных наборов данных и необходимость детектирования различных типов атак. CatBoost рекомендуется к использованию в передовых рамках информационной безопасности для критической инфраструктуры, облачных сервисов и корпоративных сетей для защиты от цифровых угроз. Данная работа предлагает быстрое, точное и масштабируемое решение для сетевой IDS и подчеркивает важность использования кастомизированных моделей машинного обучения в информационной безопасности. В дальнейшем предполагается изучить применение CatBoost на большем количестве наборов данных и его интеграцию с другими методами машинного обучения для повышения устойчивости и точности обнаружения.
Ключевые слова: информационная безопасность, обнаружение сетевых вторжений, атаки DoS, атаки DDoS, машинное обучение, обнаружение в реальном времени, отбор признаков, оптимизация модели
Список литературы
Список литературы
- Hajjouz A., Avksentieva E.Y. An approach to configuring CatBoost for advanced detection of DoS and DDoS attacks in network traffic // Vestnik of Astrakhan State Technical University. Series: Management, computer science and informatics. 2024. V. 2024. N 3. P. 64–74. https://doi.org/10.24143/2072-9502-2024-3-65-74
- Zhou L., Zhu Y., Zong T, Xiang Y. A feature selection-based method for DDoS attack flow classification // Future Generation Computer Systems. 2022. V. 132. P. 67–79. https://doi.org/10.1016/j.future.2022.02.006
- Eliyan L.F., Di Pietro R. DoS and DDoS attacks in Software Defined Networks: A survey of existing solutions and research challenges // Future Generation Computer Systems. 2021. V. 122. P. 149–171. https://doi.org/10.1016/j.future.2021.03.011
- Игнатьев Н.А., Турсунмуротов Д.Х. Цензурирование обучающих выборок с использованием регуляризации отношений связанности объектов классов // Научно-технический вестник информационных технологий, механики и оптики. 2024. Т. 24. N 2. С. 322–329. https://doi.org/10.17586/2226-1494-2024-24-2-322-329
- Alhijawi B., Almajali S., Elgala H., Salameh H.B., Ayyash M. A survey on DoS/DDoS mitigation techniques in SDNs: Classification, comparison, solutions, testing tools and datasets // Computers and Electrical Engineering. 2022. V. 99. P. 107706. https://doi.org/10.1016/j.compeleceng.2022.107706
- Li Y., Liu Q. A comprehensive review study of cyber-attacks and cyber security; Emerging trends and recent developments // Energy Reports. 2021. V. 7. P. 8176–8186. https://doi.org/10.1016/j.egyr.2021.08.126
- Karatas G., Demir O., Sahingoz O.K. Increasing the performance of machine learning-based IDSs on an imbalanced and up-to-date dataset // IEEE Access. 2020. V. 8. P. 32150–32162. https://doi.org/10.1109/ACCESS.2020.2973219
- Kim J., Kim J., Kim H., Shim M., Choi E. CNN-based network intrusion detection against denial-of-service attacks // Electronics. 2020. V. 9. N 6. P. 916. https://doi.org/10.3390/electronics9060916
- Dora V.R.S., Lakshmi V.N. Optimal feature selection with CNN-feature learning for DDoS attack detection using meta-heuristic-based LSTM // International Journal of Intelligent Robotics and Applications. 2022. V. 6 N 2. P. 323–349. https://doi.org/10.1007/s41315-022-00224-4
- Abu Bakar R., Huang X., Javed M.S., Hussain S., Majeed M.F. An intelligent agent-based detection system for DDoS attacks using automatic feature extraction and selection // Sensors. 2023. V. 23. N 6. P. 3333. https://doi.org/10.3390/s23063333
- Farhat S., Abdelkader M., Meddeb-Makhlouf A., Zarai F. Evaluation of DoS/DDoS Attack Detection with ML Techniques on CIC-IDS2017 Dataset // Proc. of the 9th International Conference on Information Systems Security and Privacy ICISSP. 2023. V. 1. P. 287–295. https://doi.org/10.5220/0011605700003405
- Manimurugan S., Al-Mutairi S., Aborokbah M.M., Chilamkurti N., Ganesan S., Patan R. Effective attack detection in internet of medical things smart environment using a deep belief neural network // IEEE Access. 2020. V. 8. P. 77396–77404. https://doi.org/10.1109/ACCESS.2020.2986013
- Rios V.D.M., Inácio P.R., Magoni D., Freire M.M. Detection and mitigation of low-rate denial-of-service attacks: A survey // IEEE Access. 2022. V. 10, P. 76648–76668. https://doi.org/10.1109/ACCESS.2022.3191430
- Faria V.D.S., Gonçalves J.A., Silva C.A.M.D., Vieira G.D.B., Mascarenhas D.M. SDToW: a slowloris detecting tool for WMNs // Information, 2020. V. 11. N 12. P. 544. https://doi.org/10.3390/info11120544
- Mahjabin S. Implementation of DoS and DDoS attacks on cloud servers // Periodicals of Engineering and Natural Sciences. 2018. V. 6 N 2. P. 148–158. https://doi.org/10.21533/pen.v6i2.170
- Kshirsagar D., Kumar S. An ontology approach for proactive detection of HTTP flood DoS attack // International Journal of System Assurance Engineering and Management. 2023. V. 14. Suppl. 3. P. 840–847. https://doi.org/10.1007/s13198-021-01170-3
- Cai Y.X., Chen S.C., Wang C.C. An Implementation of feature selection for detecting LOIC-based DDoS attack // Proc. of the International Conference on Consumer Electronics - Taiwan (ICCE-Taiwan). 2023. P. 607–608. https://doi.org/10.1109/ICCE-Taiwan58799.2023.10226733
- Nayyar S., Arora S., Singh M. Recurrent neural network based intrusion detection system // Proc. of the International Conference on Communication and Signal Processing (ICCSP). 2020. P. 136–140. https://doi.org/10.1109/ICCSP48568.2020.9182099
- Hajjouz A., Avksentieva E. Evaluating the effectiveness of the CatBoost classifier in distinguishing benign traffic, FTP BruteForce and SSH BruteForce traffic // Proc. of the 9th International Conference on Signal and Image Processing (ICSIP). 2024. P. 351-358. https://doi.org/10.1109/ICSIP61881.2024.10671552
- Sharafaldin I., Lashkari A.H., Ghorbani A.A. Toward generating a new intrusion detection dataset and intrusion traffic characterization // Proc. of the 4th International Conference on Information Systems Security and Privacy (ICISSP). 2018. V. 1. P. 108–116. https://doi.org/10.5220/0006639801080116
- Cabello-Solorzano K., Ortigosa de Araujo I., Peña M., Correia, L., Tallón-Ballesteros A.J., The impact of data normalization on the accuracy of machine learning algorithms: a comparative analysis // Lecture Notes in Networks and Systems. 2023. V. 750. P. 344–353. https://doi.org/10.1007/978-3-031-42536-3_33
- Oleghe O. A predictive noise correction methodology for manufacturing process datasets // Journal of Big Data. 2020. V. 7. N 1. P. 89. https://doi.org/10.1186/s40537-020-00367-w
- Umar M.A., Chen Z., Shuaib K., Liu Y. Effects of feature selection and normalization on network intrusion detection // Data Science and Management. 2025. V. 8. N 1. P. 23-39. https://doi.org/10.1016/j.dsm.2024.08.001
- Chandrashekar G., Sahin F. A survey on feature selection methods // Computers & electrical engineering. 2014. V. 40. N 1. P. 16–28. https://doi.org/10.1016/j.compeleceng.2013.11.024
- Palo H.K., Sahoo S., Subudhi A.K. Dimensionality reduction techniques: Principles, benefits, and limitations // Data Analytics in Bioinformatics: A Machine Learning Perspective. 2021. P. 79–107. https://doi.org/10.1002/9781119785620.ch4
- Dunn J., Mingardi L., Zhuo Y.D. Comparing interpretability and explainability for feature selection // arXiv. 2021. arXiv:2105.05328. https://doi.org/10.48550/arXiv.2105.05328
- Li J., Cheng K., Wang S., Morstatter F., Trevino R.P., Tang J., Liu H. Feature selection: A data perspective // ACM computing surveys. 2017. V. 50. N 6. P. 1–45. https://doi.org/10.1145/3136625
- Kathiravan P., Shanmugavadivu P., Saranya R. Mitigating imbalanced data in online social networks using Stratified K-Means Sampling // Proc. of the 8th International Conference on Business and Industrial Research (ICBIR). 2023. P. 883–888. https://doi.org/10.1109/ICBIR57571.2023.10147677
- Qi J., Ko T.W., Wood B.C., Pham T.A., Ong S.P. Robust training of machine learning interatomic potentials with dimensionality reduction and stratified sampling // npj Computational Materials. 2024. V. 10. N 1. P. 43. https://doi.org/10.1038/s41524-024-01227-4
- Siblini W., Fréry J., He-Guelton L., Oblé F., Wang Y.Q. Master your metrics with calibration // Lecture Notes in Computer Science. 2020. V. 12080. P. 457–469. https://doi.org/10.1007/978-3-030-44584-3_36
- Салахутдинова К.И., Лебедев И.С., Кривцова И.Е. Алгоритм градиентного бустинга деревьев решений в задаче идентификации программного обеспечения // Научно-технический вестник информационных технологий, механики и оптики. 2018. V. 18. № 6. C. 1016–1022. https://doi.org/10.17586/2226-1494-2018-18-6-1016-1022
- Prokhorenkova L., Gusev G., Vorobev A., Dorogush A. V., Gulin A. CatBoost: unbiased boosting with categorical features // Proc. of the Advances in neural information processing systems 31 (NeurlPS 2018). 2018.
- Dorogush A.V., Gulin A., Gusev G., Kazeev N., Prokhorenkova L.O., Vorobev A. 2017. Fighting biases with dynamic boosting // arXiv. 2017. arXiv:1706.09516. https://doi.org/10.48550/arXiv.1706.09516
- Dorogush A.V., Ershov V., Gulin A. CatBoost: gradient boosting with categorical features support // arXiv. 2018. arXiv:1810.11363. https://doi.org/10.48550/arXiv.1810.11363
- Ami A.S., Moran K., Poshyvanyk D., Nadkarni A. «False negative-that one is going to kill you»: Understanding Industry Perspectives of Static Analysis based Security Testing // Proc. of the IEEE Symposium on Security and Privacy (SP). 2024. P. 3979–3997. https://doi.org/10.1109/SP54263.2024.00019
- Heydarian M., Doyle T.E., Samavi R., MLCM: Multi-label confusion matrix // IEEE Access. 2022. V. 10. P. 19083–19095. https://doi.org/10.1109/ACCESS.2022.3151048
- Chicco D., Jurman G. The advantages of the Matthews correlation coefficient (MCC) over F1 score and accuracy in binary classification evaluation // BMC genomics. 2020. V. 21. N1. P. 1–13. https://doi.org/10.1186/s12864-019-6413-7
- Bowen D., Ungar L. Generalized SHAP: Generating multiple types of explanations in machine learning // arXiv. 2020. arXiv:2006.07155. https://doi.org/10.48550/arXiv.2006.07155
- Lee Y.G., Oh J.Y., Kim D., Kim G. SHAP value-based feature importance analysis for short-term load forecasting // Journal of Electrical Engineering & Technology. 2023. V. 18. N 1. P. 579–588. https://doi.org/10.1007/s42835-022-01161-9
- Hamilton R.I., Papadopoulos P.N. Using SHAP values and machine learning to understand trends in the transient stability limit // IEEE Transactions on Power Systems. 2023. V. 39. N 1. P. 1384–1397. https://doi.org/10.1109/TPWRS.2023.3248941
- Berrar D. Cross-validation // Encyclopedia of Bioinformatics and Computational Biology. 2019. V. 1. P. 542-545. https://doi.org/10.1016/B978-0-12-809633-8.20349-X
- Tougui I., Jilbab A., El Mhamdi J. Impact of the choice of cross-validation techniques on the results of machine learning-based diagnostic applications // Healthcare informatics research. 2021. V. 27. N 3. P. 189–199. https://doi.org/10.4258/hir.2021.27.3.189