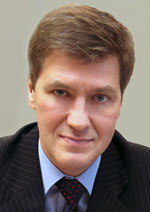
Nikiforov
Vladimir O.
D.Sc., Prof.
doi: 10.17586/2226-1494-2016-16-4-689-696
VISUAL CONCEPT LEARNING SYSTEM BASED ON LEXICAL ELEMENTS AND FEATURE KEY POINTS CONJUNCTION
Read the full article

For citation: Filatov V.I., Potapov A.S. Visual concept learning system based on lexical elements and feature key points conjunction. Scientific and Technical Journal of Information Technologies, Mechanics and Optics, 2016, vol. 16, no. 4, pp. 589–696. doi: 10.17586/2226-1494-2016-16-4-689-696
Abstract
Subject of Research. The paper deals withthe process of visual concept building based on two unlabeled sources of information (visual and textual). Method. Visual concept-based learning is carried out with image patterns and lexical elements simultaneous conjunction. Concept-based learning consists of two basic stages: early learning acquisition (primary learning) and lexical-semantic learning (secondary learning). In early learning acquisition stage the visual concept dictionary is created providing background for the next stage. The lexical-semantic learning makes two sources timeline analysis and extracts features in both information channels. Feature vectors are formed by extraction of separated information units in both channels. Mutual information between two sources describes visual concepts building criteria. Main Results. Visual concept-based learning system has been developed; it uses video data with subtitles. The results of research have shown principal ability of visual concepts building by our system. Practical Relevance.Recommended application area of described system is an object detection, image retrieval and automatic building of visual concept-based data tasks.
Acknowledgements. This work was supported by the Ministry of Education and Science of the Russian Federation and partially by the Government support of leading universities of the Russian Federation (074-U01 subsidy).
References
1. Ito S., Yoshioka M., Omatu S., Kita K., Kugo K. An image recognition method by rough classification for a scene image. Artificial Life and Robotics, 2006, vol. 10, no. 2, pp. 120–125. doi: 10.1007/s10015-005-0353-9
2. Ko B., Peng J., Byun H. Region-based image retrieval using probabilistic feature relevance learning. Pattern Analysis and Application, 2001, vol. 4, no. 2–3, pp. 174–184. doi: 10.1007/s100440170015
3. Li Z. Learning Visual Concepts from Social Images: Master Thesis. Leiden Institute of Advanced Computer Science, Netherlands, 2011, 18 p.
4. Fergus R., Fei-Fei L., Perona P., Zisserman A. Learning object categories from Google’s image search. Proc. 10th IEEE Int. Conf. on Computer Vision. Beijing, China, 2005, vol. II, pp. 1816–1823. doi: 10.1109/ICCV.2005.142
5. Fei-Fei L., Fergus R., Perona P. One-shot learning of object categories. IEEE Transactions on Pattern Analysis and Machine Intelligence, 2006, vol. 28, no. 4, pp. 594–611. doi: 10.1109/TPAMI.2006.79
6. Grauman K., Darell T. The pyramid match kernel: discriminative classification with sets of image features. Proc. 10th IEEE Int. Conf. on Computer Vision. Beijing, China, 2005, vol. II, pp. 1458–1465. doi: 10.1109/ICCV.2005.239
7. Filatov V.I. Concept-based lexical-semantic unsupervised learning system. Journal of Physics: Conference Series, 2014, vol. 536, no. 1, art. 012016. doi: 10.1088/1742-6596/536/1/012016.
8. Naphade M., Huang T. A probabilistic framework for semantic video indexing, filtering and retrieval. IEEE Transactions on Multimedia, 2001, vol. 3, no. 1, pp. 141–151. doi: 10.1109/6046.909601
9. Ranzato M. Unsupervised Learning of Feature Hierarchies: PhD Thesis. New York University, 2009, 167 p.
10. Roy D. Learning from Sights and Sounds: a Computational Model: PhD Thesis. MIT, 1999, 176 p.
11. Roy D.K., Pentland A.P. Learning words from sights and sounds: a computational model. Cognitive Science, 2002, vol. 26, no. 1, pp. 113–146. doi: 10.1016/S0364-0213(01)00061-1
12. Roy D. Learning visually grounded words and syntax of natural spoken language. Evolution of Communication, 2002, vol. 4, no. 1, pp. 33–56.
13. Lowe D.G. Object recognition from local scale-invariant features. International Conference of Computer Vision. Kerkyra, Greece, 1999, vol. 2, pp. 1150–1157.
14. Lowe D.G. Distinctive image features from scale-invariant keypoints. International Journal of Computer Vision, 2004, vol. 60, no. 2, pp. 91–110. doi: 10.1023/B:VISI.0000029664.99615.94
15. Lucas B.D., Kanade T. An iterative image registration technique with an application to stereo vision. Proc. 7th Int. Joint Conference on Artificial Intelligence. Vancouver, Canada, 1981, vol. 2, pp. 674–679.
16. Barron J., Fleet D., Beauchemin S. Performance of optical flow techniques. International Journal of Computer Vision, 1994, vol. 12, no. 1, pp. 43–77. doi: 10.1007/BF01420984
17. Bay H., Ess A. Speeded-up robust features. Computer Vision and Image Understanding, 2008, vol. 110, no. 3, pp. 346–359. doi: 10.1016/j.cviu.2007.09.014
18. Farneback G. Two-frame motion estimation based on polynomial expansion. Lecture Notes in Computer Science, 2003, vol. 2749, pp. 363–370.
19. Farneback G. Disparity estimation from local polynomial expansion. Proc. SSAD Symposium on Image Analysis, 2001.