Menu
Publications
2024
2023
2022
2021
2020
2019
2018
2017
2016
2015
2014
2013
2012
2011
2010
2009
2008
2007
2006
2005
2004
2003
2002
2001
Editor-in-Chief
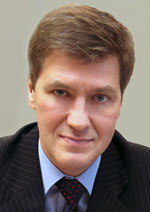
Nikiforov
Vladimir O.
D.Sc., Prof.
Partners
doi: 10.17586/2226-1494-2019-19-3-435-442
COMPARISON OF UNKNOWN PARAMETERS ESTIMATES BY METHOD OF DYNAMIC REGRESSOR EXTENSION AND MIXING AND LEAST SQUARE METHOD IN NOISE PRESENCE
Read the full article

For citation:
Abstract
Korotina M.M.,Aranovskiy S.V.,Vedyakov A.A.Comparison of unknown parameters estimates by method o fdynamic regressor extension and mix ingandleasts quare method innoisepresence.Scientific and Technical Journal of Information Technologies, Mechanics and Optics, 2019, vol. 19, no. 3, pp. 435–442 (in Russian). doi:10.17586/2226-1494-2019-19-3-435-442
Abstract
Subject of Research. The paper deals with identification of the regression model unknown parameters by two estimation algorithms: the classical least square method and a new method of dynamic regressor extension and mixing. To compare the quality of the obtained estimates, the non-stationary parameters of the regression model were considered, and various noise of limited power was added to the input signal of the model. Method. The problem was solved by the dynamic regressor extension and mixing method followed by the gradient algorithm and the least squares method in real time mode ignoring the older values of the measured input signal. Main Results. The numerical simulation was presented illustrating the qualitative comparison of the two used methods. A noisy shifted sinusoidal signal with time-varying and unknown parameters of displacement, amplitude and phase shift was applied to the input of the estimation algorithms. Comparison has shown that with the application of dynamic regressor extension and mixing method, the estimation of the input signal parameters had an aperiodic form, while the least squares method gave unwanted oscillations. Numerical simulation has shown that the method of dynamic regressor extension and mixing qualitatively exceeds the method of least squares. Practical Relevance. The results can be used when solving practical problems in the areas of processing and evaluating for both harmonic signals and also signals with a more complex form.
Keywords: identification, non-stationary parameters, dynamic regressor extension and mixing, least squares method, sinusoidal signal, white noise, colored noise
Acknowledgements. The study is supported by the Ministry of Education and Science of the Russian Federation (Government project No. 8.8885.2017/8.9)
References
Acknowledgements. The study is supported by the Ministry of Education and Science of the Russian Federation (Government project No. 8.8885.2017/8.9)
References
1. Vedyakov A.A., Vediakova A.O., Bobtsov A.A., Pyrkin A.A., Aranovskiy S.V. A globally convergent frequency estimator of a sinusoidal signal with a time-varying amplitude. European Journal of Control, 2017, vol. 38, pp. 32–38. doi: 10.1016/j. ejcon.2017.08.001
2. Aranovskii S.V., Bobtsov A.A., Nikiforov V.O. Observer design of an unknown sinusoidal output distrurbance for nonlinear plant. Scientific and Technical Journal of Information Technologies, Mechanics and Optics, 2010, no. 3, pp. 32–38. (in Russian)
3. Aranovskii S.V., Bobtsov A.A., Kremlev A.S., Luk’yanova G.V., Nikolaev N.A. Identification of frequency of a shifted sinusoidal signal. Automation and Remote Control, 2008, vol. 69, no. 9, pp. 1447–1453. doi: 10.1134/S0005117908090014
4. Le Van Tuan, Korotina M.M., Bobtsov A.A., Aranovskiy S.V. New identification algorithm for linearly varying frequency of sinusoidal signal. Scientific and Technical Journal of Information Technologies, Mechanics and Optics, 2019, vol. 19, no. 1, pp. 52– 58. (in Russian) doi: 10.17586/2226-1494-2019-19-1-52-58
5. Aranovskii S.V., Bobtsov A.A., Pyrkin A.A. Adaptive observer of an unknown sinusoidal output disturbance for linear plants. Automation and Remote Control, 2009, vol. 70, no. 11, pp. 1862– 1870. doi: 10.1134/S0005117909110101
6. Marino R., Tomei R. Global estimation of unknown frequencies. IEEE Transactions on Automatic Control, 2002, vol. 47, no. 8, pp. 1324–1328. doi: 10.1109/tac.2002.800761
7. Hou M. Amplitude and frequency estimator of a sinusoid. IEEE Transactions on Automatic Control, 2005, vol. 50, no. 6, pp. 855– 858. doi: 10.1109/tac.2005.849244
8. Aranovskiy S., Bobtsov A., Kremlev A., Nikolaev N., Slita O. Identification of frequency of biased harmonic signal. European Journal of Control, 2010, vol. 16, no. 2, pp. 129–139. doi: 10.3166/ejc.16.129-139
9. Aranovskiy S., Bobtsov A., Ortega R., Pyrkin A. Performance enhancement of parameter estimators via dynamic regressor extension and mixing. IEEE Transactions on Automatic Control, 2016, vol. 62, no. 7, pp. 3546–3550. doi: 10.1109/ tac.2016.2614889
10. Belov A.A., Aranovskiy S.V., Ortega R., Barabanov N.E., Bobtsov A.A. Enhanced parameter convergence for linear systems identification: the DREM approach. European Control Conference, ECC, 2018, pp. 2794–2799. doi: 10.23919/ ecc.2018.8550338
11. Borisov O.I., Gromov V.S., Vedyakov A.A., Pyrkin A.A., Bobtsov A.A., Aranovskiy S.V. Adaptive tracking of a multi- sinusoidal signal with DREM-based parameters estimation. IFAC-PapersOnLine, 2017, vol. 50, no. 1, pp. 4282–4287. doi: 10.1016/j.ifacol.2017.08.835
12. Gromov V.S., Borisov O.I., Pyrkin A.A., Bobtsov A.A., Kolyubin S.A., Aranovskiy S.V. The DREM approach for chaotic oscillators parameter estimation with improved performance. IFAC-PapersOnLine, 2017, vol. 50, no. 1, pp. 7027–7031. doi: 10.1016/j.ifacol.2017.08.1347
13. Sastry S., Bodson M. Adaptive Control: Stability, Convergence and Robustness. Prentice-Hall, New Jersey, 1989, 377 p.
14. Barton R.J., Poor H.V. Signal detection in fractional Gaussian noise. IEEE Transactions on Information Theory, 1988, vol. 34, no. 5, pp. 943–959. doi: 10.1109/18.21218
15. Barnes J.A., Allan D.W. A statistical model of flicker noise. Proc. IEEE, 1966, vol. 54, no. 2, pp. 176–178. doi: 10.1109/ proc.1966.4630
16. Flandrin P. Wavelet analysis and synthesis of fractional Brownian motion. IEEE Transaction on Information Theory, 1992, vol. 38, no. 2, pp. 910–917. doi: 10.1109/18.119751
2. Aranovskii S.V., Bobtsov A.A., Nikiforov V.O. Observer design of an unknown sinusoidal output distrurbance for nonlinear plant. Scientific and Technical Journal of Information Technologies, Mechanics and Optics, 2010, no. 3, pp. 32–38. (in Russian)
3. Aranovskii S.V., Bobtsov A.A., Kremlev A.S., Luk’yanova G.V., Nikolaev N.A. Identification of frequency of a shifted sinusoidal signal. Automation and Remote Control, 2008, vol. 69, no. 9, pp. 1447–1453. doi: 10.1134/S0005117908090014
4. Le Van Tuan, Korotina M.M., Bobtsov A.A., Aranovskiy S.V. New identification algorithm for linearly varying frequency of sinusoidal signal. Scientific and Technical Journal of Information Technologies, Mechanics and Optics, 2019, vol. 19, no. 1, pp. 52– 58. (in Russian) doi: 10.17586/2226-1494-2019-19-1-52-58
5. Aranovskii S.V., Bobtsov A.A., Pyrkin A.A. Adaptive observer of an unknown sinusoidal output disturbance for linear plants. Automation and Remote Control, 2009, vol. 70, no. 11, pp. 1862– 1870. doi: 10.1134/S0005117909110101
6. Marino R., Tomei R. Global estimation of unknown frequencies. IEEE Transactions on Automatic Control, 2002, vol. 47, no. 8, pp. 1324–1328. doi: 10.1109/tac.2002.800761
7. Hou M. Amplitude and frequency estimator of a sinusoid. IEEE Transactions on Automatic Control, 2005, vol. 50, no. 6, pp. 855– 858. doi: 10.1109/tac.2005.849244
8. Aranovskiy S., Bobtsov A., Kremlev A., Nikolaev N., Slita O. Identification of frequency of biased harmonic signal. European Journal of Control, 2010, vol. 16, no. 2, pp. 129–139. doi: 10.3166/ejc.16.129-139
9. Aranovskiy S., Bobtsov A., Ortega R., Pyrkin A. Performance enhancement of parameter estimators via dynamic regressor extension and mixing. IEEE Transactions on Automatic Control, 2016, vol. 62, no. 7, pp. 3546–3550. doi: 10.1109/ tac.2016.2614889
10. Belov A.A., Aranovskiy S.V., Ortega R., Barabanov N.E., Bobtsov A.A. Enhanced parameter convergence for linear systems identification: the DREM approach. European Control Conference, ECC, 2018, pp. 2794–2799. doi: 10.23919/ ecc.2018.8550338
11. Borisov O.I., Gromov V.S., Vedyakov A.A., Pyrkin A.A., Bobtsov A.A., Aranovskiy S.V. Adaptive tracking of a multi- sinusoidal signal with DREM-based parameters estimation. IFAC-PapersOnLine, 2017, vol. 50, no. 1, pp. 4282–4287. doi: 10.1016/j.ifacol.2017.08.835
12. Gromov V.S., Borisov O.I., Pyrkin A.A., Bobtsov A.A., Kolyubin S.A., Aranovskiy S.V. The DREM approach for chaotic oscillators parameter estimation with improved performance. IFAC-PapersOnLine, 2017, vol. 50, no. 1, pp. 7027–7031. doi: 10.1016/j.ifacol.2017.08.1347
13. Sastry S., Bodson M. Adaptive Control: Stability, Convergence and Robustness. Prentice-Hall, New Jersey, 1989, 377 p.
14. Barton R.J., Poor H.V. Signal detection in fractional Gaussian noise. IEEE Transactions on Information Theory, 1988, vol. 34, no. 5, pp. 943–959. doi: 10.1109/18.21218
15. Barnes J.A., Allan D.W. A statistical model of flicker noise. Proc. IEEE, 1966, vol. 54, no. 2, pp. 176–178. doi: 10.1109/ proc.1966.4630
16. Flandrin P. Wavelet analysis and synthesis of fractional Brownian motion. IEEE Transaction on Information Theory, 1992, vol. 38, no. 2, pp. 910–917. doi: 10.1109/18.119751