Menu
Publications
2024
2023
2022
2021
2020
2019
2018
2017
2016
2015
2014
2013
2012
2011
2010
2009
2008
2007
2006
2005
2004
2003
2002
2001
Editor-in-Chief
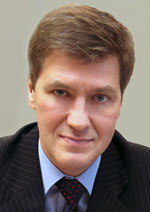
Nikiforov
Vladimir O.
D.Sc., Prof.
Partners
doi: 10.17586/2226-1494-2020-20-2-249-256
DYNAMICS OF CENTRALITY MEASURES OF RANDOM GRAPH MATHEMATICAL MODELS
Read the full article

Article in Russian
For citation:
Abstract
For citation:
Shuvaev F.L., Tatarka M.V. Dynamics of centrality measures of random graph mathematical models. Scientific and Technical Journal of Information Technologies, Mechanics and Optics, 2020, vol. 20, no. 2, pp. 249–256 (in Russian). doi: 10.17586/2226-1494-2020-20-2-249-256
Abstract
Subject of Research. Simulation is one of the most powerful tools among information security provision measures in the design process of communication systems. As compared to other methods, it considers large-capacity communication systems, improves the quality of network resource management solutions and predicts more accurately their consequences. In this case, random graphs are the basic mathematical models for the analyzed systems. They provide fundamental understanding of the analyzed network properties and serve as the basis for simulation. With regard to the processing power high development rate for computers and simulation environments, the study of the topological properties of random graphs becomes especially urgent. It involves analyzing the probabilistic dynamics of centrality measures. Method. In the experiment we used centrality calculation methods for vertices and the graph as a whole based on the scientific apparatus of the graph theory. Comparison method based on span diagrams was used in the study of probabilistic dynamics of graph mathematical models. Main Results. We have studied the dynamics of centrality measures in the Erdös-Renyi random graph model, the Watts-Strogatz small world model and the freely scalable Barabashi-Albert model. The centrality measures of these models have been compared with a real network. We have made it clear that the topological properties of a real network are described by the Barabashi-Albert model to the fullest extent possible. The analysis of centrality measures presented in the paper gives the possibility to trace interconnections between the parameters of various graph models, that, in turn, can be used in the analysis of real networks. Practical Relevance. The obtained results can be applied in modeling of physical and social systems presented in the form of graphs. The paper findings are of interest for professionals involved in the analysis of networks in various fields of science and technology: sociology, medicine, physics and radio engineering.
Keywords: graph, node, betweenness centrality, closeness centrality, centrality based on degree, span diagram
References
References
-
Raigorodskii A.M. Random Graph Models. Moscow, MCCME Publ., 2011, 134 p. (in Russian)
-
Shevchenko D.N., Litvin A.U., Fedianin M.A. Reliability analysis of technical systems by simulation modeling of graph of states. Problems of Physics, Mathematics and Technics, 2018, no. 3(36), pp. 101–104. (in Russian)
-
Chen P-Y., Choudhury S., Hero A.O. Multi-centrality graph spectral decompositions and their application to cyber intrusion detection. Proc. 41st IEEE International Conference on Acoustics, Speech and Signal Processing (ICASSP 2016), 2016, pp. 4553–4557. doi:10.1109/ICASSP.2016.7472539
-
Newman M.E.J.Networks: an Introduction. N.Y., Oxford University Press Inc., 2010, 1042 p.
-
Rodrigue J.-P. The Geography of Transport Systems. Taylor & Francis, 2017, 440 p.
-
Watts D., Strogatz H. Collective dynamics of «Small-world» networks. Nature, 1998, vol. 393, no. 6684, pp. 440–442. doi: 10.1038/30918
-
Hartmann A., Mézard M. Distribution of diameters for Erdős-Rényi random graphs. Physical Review E, 2018, vol. 97, no. 3, pp. 032128. doi: 10.1103/PhysRevE.97.032128
-
Le C., Levina E., Vershynin R. Concentration and regularization of random graphs. Random Structures and Algorithms, 2017, vol. 51, no. 3, pp. 538–561. doi: 10.1002/rsa.20713
-
Bonchi F., De Francisci G., Riondato M. Centrality measures on big graphs: exact, approximated, and distributed algorithms. Proc. 25th International Conference Companion on World Wide Web, 2016, pp. 1017–1020. doi:10.1145/2872518.2891063
-
Shcherbakova N.G. Centrality measures in networks. Problems of Informatics, 2015, no. 2, pp. 18–30. (in Russian)
-
Bredikhin S.V., Liapunov V.M., Shcherbakova N.G. Importance measure of scientific periodicals – “Betweenness centrality”. Problems of Informatics, 2014, no. 3, pp. 53–63. (in Russian)
-
Brandes U., Borgatti S., Freeman L.C. Maintaining the duality of closeness and betweenness centrality. Social Networks, 2016, vol. 44, pp. 153–159. doi: 10.1016/j.socnet.2015.08.003
-
Yudina M.N. The nodes of social networks: measures of centrality and role in network processes. Omsk Scietntific Bulletin, 2016, no. 4, pp. 161–165. (in Russian)
-
Piraveenan M. Topological analysis of complex networks using assortativity. PhD Diss. Sydney, University of Sydney, 2010, 189 p.
-
Van Mieghem P., Ge X., Schumm P., Trajanovski S., Wang H. Spectral graph analysis of modularity and assortativity. Physical Review E, 2010, vol. 82, no. 5, pp. 056113. doi: 10.1103/PhysRevE.82.056113
-
Barzel B., Biham O. Quantifying the connectivity of a network: the network correlation function method. Physical Review E, 2009, vol. 80, no. 4, pp. 046104. doi: 10.1103/PhysRevE.80.046104
-
Gibson H., Vickers P. Using adjacency matrices to lay out larger small-world networks. Applied Soft Computing Journal, 2016, vol. 42, pp. 80–92. doi: 10.1016/j.asoc.2016.01.036
-
Barabasi A. Network Science. Glasgow, Cambridge University Press, 2016, 453 p.
-
Stauffer D., Meyer-Ortmanns H. Simulation of consensus model of deffuant et al. on a Barabási–Albert network. International Journal of Modern Physics, 2004,vol. 15, no. 2, pp. 241–246. doi: 10.1142/S0129183104005644
-
Luke D. A User’s Guide to Network Analysis in R. Springer, 2015, 238 p.