Menu
Publications
2025
2024
2023
2022
2021
2020
2019
2018
2017
2016
2015
2014
2013
2012
2011
2010
2009
2008
2007
2006
2005
2004
2003
2002
2001
Editor-in-Chief
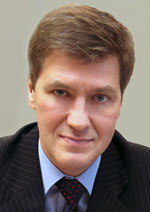
Nikiforov
Vladimir O.
D.Sc., Prof.
Partners
MATROIDAL REPRESENTATION FOR THE ADJACENCY GRAPHS FAMILY BUILT ON A SET OF KNOWLEDGE PATTERNS
Read the full article

Abstract
The paper considers a problem appeared in machine learning of uncertain knowledge patterns bases represented as algebraic Bayesian networks. The problem is to build an adjacency graph as a representation of a global (secondary) structure of such a network using its primary structure. Under the given primary structure of an algebraic Bayesian network, the corresponding adjacency graphs family can be characterized with a special matroid. It leads to further results: the sets of minimal adjacency graphs and non-reducible join graphs are equal; a minimal adjacency graph can be built with a greedy algorithm; the vertices number of minimal adjacency graph can be expressed with the vertices number of maximal adjacency graph and the matroid rank.
Keywords:
knowledge patterns bases, algebraic Bayesian networks, machine learning, global structure, matroid, adjacency graph