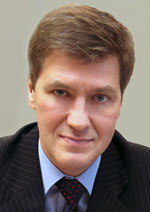
НИКИФОРОВ
Владимир Олегович
д.т.н., профессор
doi: 10.17586/2226-1494-2016-16-6-967-995
УДК 004.9+004.21
СУПЕРКОМПЬЮТЕРНОЕ МОДЕЛИРОВАНИЕ КРИТИЧЕСКИХ ЯВЛЕНИЙ В СЛОЖНЫХ СОЦИАЛЬНЫХ СИСТЕМАХ
Читать статью полностью

Ссылка для цитирования: Слоот П.М.А., Холист Я., Кампис Дж., Лис М.Х., Митягин С.А., Иванов С.В., Боченина К.О., Гулева В.Ю., Мухина К.Д., Насонов Д.А., Бутаков Н.А., Леоненко В.А., Ланцева А.А., Бухановский А.В. Суперкомпьютерное моделирование критических явлений в сложных социальных системах // Научно-технический вестник информационных технологий, механики и оптики. 2016. Т. 16. № 6. С. 967–995. doi: 10.17586/2226-1494-2016-16-6-967-995
Аннотация
В статье рассмотрена проблема компьютерного моделирования критических явлений в сложных социальных системах на основе формализма комплексных сетей с использованием вычислительных систем петафлопсного диапазона производительности. Предложена система вложенных моделей комплексной сети на трех уровнях, включающая агрегированную аналитическую модель для идентификации критических состояний, детализированную модель динамики сети на индивидуальном уровне, а также модель, уточняющую особенности топологической структуры комплексной сети. Разработан масштабируемый параллельный алгоритм, в совокупности покрывающий все уровни моделирования комплексных сетей, и исследована его производительность на различных суперкомпьютерных системах. Обсуждены вопросы программно-информационной инфраструктуры моделирования комплексных сетей, включая организацию распределенных вычислений, сбор данных в социальных сетях, а также визуализацию результатов. Рассмотрены приложения разработанных методов и технологий в части моделирования распада криминальных сетей, быстрого распространения слухов в социальных сетях Интернет, эволюции финансовых сетей и распространения эпидемий.
Благодарности. Работа выполнена при поддержке проекта Российского научного фонда, соглашение № 14-21-00137.
Список литературы
3. Bordogna C.M., Albano E.V. Statistical methods applied to the study of opinion formation models: a brief overview and results of a numerical study of a mod-el based on the social impact theory // Journal of Physics Condensed Matter. 2007. V. 19. N 6. Art. 065144. 10.1088/0953-8984/19/6/065144
4. Fortunato S., Castellano C. Scaling and universality in proportional elections // Physical Review Letters. 2007. V. 99. N 13. Art. 138701. doi: 10.1103/PhysRevLett.99.138701
5. Klimek P., Lambiotte R., Thurner S. Opinion formation in laggard societies // EPL. 2008. V. 82. N 2. Art. 28008. doi: 10.1209/0295-5075/82/28008
6. Chmiel A., Sienkiewicz J., Thelwall M., Paltoglou G., Buckley K., Kappas A., Holyst J.A. Collective emotions online and their influence on community life // PloS One. 2011. V. 6. N 7. Art. e22207 doi: 10.1371/journal.pone.0022207
7. Chmiel A., Holyst J.A. Transition due to preferential cluster growth of collective emotions in online communities // Physical Review E. 2013. V. 87. N 2. Art. 022808. doi:0.1103/PhysRevE.87.022808
8. Gil S., Zanette D.H. Coevolution of agents and networks: opinion spreading and community disconnection // Physics Letters A. 2006. V. 356. N 2. P. 89–94. doi:10.1016/j.physleta.2006.03.037
9. Kacperski K., Holyst J.A. Phase transitions and hysteresis in a cellular automata-based model of opinion formation // Journal of Statistical Physics. 1996. V. 84. N 1-2. P. 169–189.
10. Newman M. Networks: An Introduction. Oxford University Press, 2010. 784 p.
11. Snijders T.A., Van de Bunt G.G., Steglich C.E.G. Introduction to stochastic actor-based models for network dynamics. Social Networks. 2010. V. 32. P. 44–60. doi:10.1016/j.socnet.2009.02.004
12. Kumar R., Novak J., Tomkins A. Structure and evolution of online social networks / In: Link Mining: Models, Algorithms, and Applications. NY: Springer, 2010. P. 337–357. doi: 10.1007/978-1-4419-6515-8-13
13. Leskovec J., Huttenlocher D., Kleinberg J. Predicting positive and negative links in online social networks // Proc. 19th Int. Conf. on World Wide Web. Raleigh, USA, 2010. P. 641–650. doi: 10.1145/1772690.1772756
15. Lamberson P.J., Page S.E. Tipping points // Quarterly Journal of Political Science. 2012. V. 7. N 2. P. 175–208. doi: 10.1561/100.00011061
16. O’Regan S.M., Drake J.M. Theory of early warning signals of disease emergence and leading indicators of elimination // Theoretical Ecology. 2013. V. 6. N 3. P. 333–357. doi:10.1007/s12080-013-0185-5
17. Dorogovtsev S.N., Goltsev A. V., Mendes J.F.F. Critical phenomena in complex networks // Reviews of Modern Physics. 2007. V. 80. N 4. P. 1275–1335. doi:10.1103/RevModPhys.80.1275
18. Seoane L.F., Sole R. Phase transitions in Pareto optimal complex networks // Physical Review E - Statistical, Nonlinear, and Soft Matter Physics. 2015. V. 92. Art. 032807. doi:10.1103/PhysRevE.92.032807
19. Ye C., Torsello a., Wilson R.C., Hancock E.R. Thermodynamics of time evolving networks // Lecture Notes in Computer Science. 2015. V. 9069. P. 315–324. doi: 10.1007/978-3-319-18224-7_31
20. Ni K.-Y., Lu T.-C. Information dynamic spectrum characterizes system instability toward critical transitions // EPJ Data Science. 2014. V. 3. N 1. P. 1–25. doi:10.1140/epjds/s13688-014-0028-7
21. Guleva V.Y. The combination of topology and nodes’ states dynamics as an early-warning signal of critical transition in a banking network model // Procedia Computer Science. 2016. V. 80. P. 1755–1764. doi: 10.1016/j.procs.2016.05.436
22. Luque B., Ballesteros F.J., Muro E.M. Self-organized critical random Boolean networks // Physical Review E - Statistical, Nonlinear, and Soft Matter Physics. 2001. V. 63. N 5. Art. 51913. doi: 10.1103/PhysRevE.63.051913
23. Han H., Ma A., Huang Z. An improved SIRS epidemic model on complex network // Proc. Int. Conf. on Computational Intelligence and Software Engineering. Wuhan, China, 2009. Art. 5365422. doi: 10.1109/CISE.2009.5365422
24. Delpini D., Battiston S., Riccaboni M., Gabbi G., Pammolli F., Caldarelli G. Evolution of controllability in interbank networks // Scientific Reports. 2013. V. 3. Art. 1626. doi:10.1038/srep01626
25. Bisset K.R., Chen J., Feng X., Kumar V.S.A., Marathe M.V. EpiFast: a fast algorithm for large scale realistic epidemic simulations on distributed memory systems // Proc. 23rd Int. Conf. on Supercomputing. Yorktown, USA, 2009. P. 430–439. doi: 10.1145/1542275.1542336
26. Hou B., Yao Y., Wang B., Liao D. Modeling and simulation of large-scale social networks using parallel discrete event simulation // Simulation. 2013. V. 89. N 10. P. 1173–1183. doi: 10.1177/0037549713495752
27. Gonzalez J.E., Low Y., Gu H., Bickson D., Guestrin C. PowerGraph: distributed graph-parallel computation on natural graphs // Proc. 10th USENIX Conference on Operating Systems Design and Implementation. Hollywood, USA, 2012. P. 17–30.
28. Liu Y.-Y., Slotine J.-J., Barabasi A.-L. Observability of complex systems // Proc. National Academy of Science of the USA. 2013. V. 110. N 7. P. 2460–2465. doi:10.1073/pnas.1215508110
29. Yoo A., Henderson K. Parallel generation of massive scale-free graphs // Proceedings of CoRR. 2010.
30. Ryu E., Choi S. Stochastic Kronecker Graph on Vertex-Centric BSP // Proceedings of CoRR. 2012. Art. arXiv1208.2239.
31. Paul G., Tanizawa T., Havlin S., Stanley H.E. Optimization of robustness of complex networks // The European Physical Journal B - Condensed Matter and Complex Systems. 2004. V. 38. N 2. P. 187–191. doi: 10.1140/epjb/e2004-00112-3
32. Watts D.J., Strogatz S.H. Collective dynamics of ‘small-world’ networks // Nature. 1998. V. 393. N 6684. P. 440–442. doi: 10.1038/30918
33. Albert R., Barabasi A.L. Statistical mechanics of complex networks // Reviews of Modern Physics. 2002. V. 74. N 1. P. 47–97. doi: 10.1103/RevModPhys.74.47
34. Newman M.E.J. The structure and function of complex networks // SIAM Review. 2003. V. 45. N 2. P. 167–256.
35. Kashirin V.V. Evolutionary simulation of complex networks structures with specific topological properties // Procedia Computer Science. 2014. V. 29. P. 2401–2411. doi:10.1016/j.procs.2014.05.224
36. Voloshin D.V., Puzyreva K.A., Karbovskii V.A. Agent-based virtual society polygon for simulation and evaluation in massive mobile services // IERI Procedia. 2014. V. 10. P. 231–238. doi:
37. Mahdian M., Xu Y. Stochastic kronecker graphs // Lecture Notes in Computer Science. 2007. V. 4863. P. 179–186.
38. Knyazkov K.V., Kovalchuk S.V., Tchurov T.N., Maryin S.V., Boukhanovsky A.V. CLAVIRE: e-Science infrastructure for data-driven computing // Journal of Computational Science. 2012. V. 3. N 6. P. 504–510. doi: 10.1016/j.ieri.2014.09.082
39. Xiong F., Liu Y., Zhang Z.-J., Zhu J., Zhang Y. An information diffusion model based on retweeting mechanism for online social media // Physics Letters A. 2012. V. 376. N 30. P. 2103–2108. doi: 10.1016/j.jocs.2012.08.006
40. Gjoka M., Kurant M., Butts C.T., Markoulou A. Walking in facebook: a case study of unbiased sampling of OSNs // Proceedings IEEE INFOCOM. San Diego, USA, 2010. Art. 5462078. doi: 10.1016/j.physleta.2012.05.021
41. Arnaboldi V., Conti M., Passarella A., Pezzoni F. Ego networks in twitter: an experimental analysis // Proceedings IEEE INFOCOM. Turin, Italy, 2013. P. 3459–3464. doi:10.1109/INFCOM.2010.5462078
42. Lee C.H., Xu X., Eun D.Y. Beyond random walk and metropolis-hastings samplers: why you should not backtrack for unbiased graph sampling // Performance Evaluation Review. 2012. V. 40. N 1. P. 319–330. doi: 10.1103/PhysRevLett.86.2050
43. Wang F., Landau D.P. Efficient, multiple-range random walk algorithm to calculate the density of states // Physical Review Letters. 2001. V. 86. N 10. P. 2050–2053. doi:
44. Buccafurri F., Lax G., Nocera A., Ursino D. Crawling social internetworking systems // Proc. Int. Conf. on Advances in Social Networks Analysis and Mining (ASONAM). Istanbul, Turkey, 2012. P. 506–510. doi: 10.1109/ASONAM.2012.87
45. Buccafurri F., Lax G., Nocera A., Ursino D. A system for extracting structural information from Social Network accounts // Software – Practice and Experience. 2015. V. 45. N 9. P. 1251–1275. doi: 10.1002/spe.2280
46. Serrano D., Stroulia E., Barbosa D., Guana V. SociQL: a query language for the socialweb // Advances in Network Analysis and its Applications. 2012. V. 18. P. 381–406. doi:10.1007/978-3-642-30904-5_17
47. Marcus A., Bernstein M.S., Badar O., Karger D.R., Madden S., Miller R.C. Processing and visualizing the data in tweets // SIGMOD Record. 2012. V. 40. N 4. P. 21–27. doi:10.1145/2094114.2094120
48. Psallidas F., Ntoulas A., Delis A. SocWeb: efficient monitoring of social network activities // Lecture Notes in Computer Science. 2013. V. 8181. P. 118–136. doi: 10.1007/978-3-642-41154-0_9
49. Hu Y., Shi L. Visualizing large graphs // Wiley Interdisciplinary Reviews: Computational Statistics. 2015. V. 7. N 2. P. 115–136. doi: 10.1002/wics.1343
50. Auber D., Chiricote Y., Jourdan F., Melancon G. Multiscale visualization of small world networks // Proc. IEEE Symposium on Information Visualization. Seattle, USA, 2003. P. 75–84.
51. Tominski C., Abello J., Van Ham F., Schumann H. Fisheye tree views and lenses for graph visualization // Proc. Int. Conf. on Information Visualization. London, 2006. P. 17–24. doi: 10.1109/IV.2006.54
52. Bender-deMoll S., McFarland D.A. The art and science of dynamic network visualization // Journal of Social Structure. 2006. V. 7. N 2. P. 1–38.
53. Moody J., McFarland D., Bender-deMoll S. Dynamic network visualization // American Journal of Sociology. 2005. V. 110. P. 1206–1241. doi: 10.1086/421509
54. Collberg C., Kobourov S., Nagra J., Pitts J., Wampler K. A system for graph-based visualization of the evolution of software // Proc. ACM Symposium on Software Visualization. San Diego, USA, 2003. P. 77–86.
55. Ahn J.-W., Taieb-Maimon M., Sopan A., Plaisant C., Shneiderman B. Temporal visualization of social network dynamics: prototypes for nation of neighbors // Lecture Notes in Computer Science. 2011. V. 6589. P. 309–316. doi: 10.1007/978-3-642-19656-0_43
56. Egorov D., Bezgodov A. Improved force-directed method of graph layout generation with adaptive step length // Procedia Computer Science. 2015. V. 66. P. 689–696. doi:10.1016/j.procs.2015.11.078
57. Guschin A., Shmelev V., Karbovskii V. Use of modern information and computer technologies in historical and urban studies with the example of epidemiological situation in the city of Simbirsk during the First World War // Proc. 9th Int. Conf. on Application of Information and Communication Technologies. Rostov-on-Don, Russia, 2015. P. 527–530. doi:10.1109/ICAICT.2015.7338616
58. Goodhart C., Hofmann B., Segoviano M. Bank regulation and macroeconomic fluctuations // Oxford Review of Economic Policy. 2004. V. 20. N 4. P. 591–615. doi:10.1093/oxrep/grh034
59. Rudebusch G.D. et al. Macroeconomic models for monetary policy // FRBSF Economic Letter. 2002.
60. Sparrow M.K. The application of network analysis to criminal intelligence: an assessment of the prospects // Social Networks. 1991. V. 13. N 3. P. 251–274. doi: 10.1016/0378-8733(91)90008-H
61. Duijn P.A.C., Klerks P. Social network analysis applied to criminal networks: recent developments in Dutch law enforcement / In: Networks and Network Analysis for Defense and Security. Springer, 2014. P. 121–159. doi: 10.1007/978-3-319-04147-6_6
62. Milward H.B., Raab J. Dark networks as organizational problems: elements of a theory // International Public Management Journal. 2006. V. 9. N 3. P. 333–360. doi:10.1080/10967490600899747
63. Duijn P.A.C., Kashirin V., Sloot P.M.A. The relative ineffectiveness of criminal network disruption // Scientific Reports. 2013. V. 4. Art. 4238. doi: 10.1038/srep04238